What Are Predictive Analytics Tools?
Don't fall behind—unlock the power of predictive analytics tools to boost decision-making, efficiency, and accuracy while minimizing costs and risks.
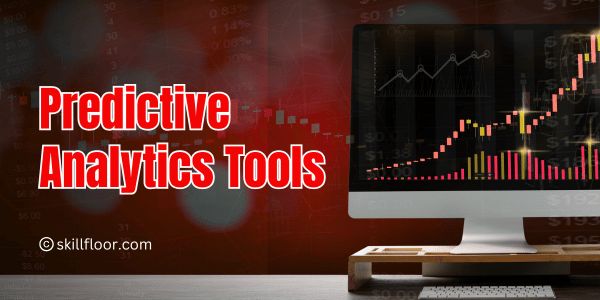
Let me ask you this: how often do you look at your business data and wonder what’s coming next? I’ve been there—going through lots of reports, trying to figure out what will happen in the future for my business. For a long time, I made decisions based on my feelings and what had happened before. But then I found predictive analytics tools, and everything changed. These tools completely changed the way I make decisions, and I want to show you how they’re helping businesses do better in today’s world.
What is Predictive Analytics?
Before going into the details of predictive analytics tools, let’s get the basics right. Predictive analytics is the process of using historical data, statistical algorithms, and machine learning techniques to identify patterns and predict future outcomes. It’s not magic—it’s data science at its finest. These tools help businesses anticipate opportunities and threats, enabling proactive rather than reactive decision-making.
I often describe predictive analytics as a compass. It doesn’t just tell you where you are but shows you where you’re likely headed. This is invaluable in a world where customer preferences, market trends, and risks shift faster than ever.
What is a Predictive Analytics Tool?
A predictive analytics tool is software designed to harness the power of data. It takes raw, often messy information from various sources and transforms it into actionable insights. These tools don’t just analyze the past; they forecast the future. Imagine having a tool that can predict customer behavior, optimize your inventory, or even flag potential fraud before it happens. That’s the kind of power we’re talking about.
These tools integrate seamlessly with existing systems like CRMs, ERPs, or marketing platforms, making them indispensable in today’s tech ecosystem. But what sets them apart are the features they bring to the table.
Top Predictive Analytics Tools
IBM SPSS (Statistical Package for the Social Sciences) is a comprehensive software suite used for statistical analysis and predictive modeling. It is widely used for analyzing large datasets and providing actionable insights through statistical methods.
Key Features:
-
Statistical and Predictive Analytics: Offers a wide range of statistical techniques and predictive analytics models, including regression, classification, and forecasting methods.
-
Regression, Classification, and Forecasting: Provides tools to perform different types of regression (linear, logistic), classification (decision trees, neural networks), and time series forecasting.
-
Data Manipulation Tools: Includes a variety of tools for data cleaning, transformation, and manipulation, such as handling missing data, data merging, and reshaping.
Who Should Use It?
-
Data scientists, business analysts, and researchers: Ideal for professionals working with statistical data to generate actionable insights for decision-making.
SAS Viya
SAS Viya is a cloud-based, scalable analytics platform designed for advanced data science, predictive modeling, machine learning, and artificial intelligence (AI). It facilitates high-performance analytics to deal with large volumes of data and provides powerful predictive capabilities.
Key Features:
-
Cloud-based with AI and Machine Learning: Built to operate on a cloud infrastructure, it integrates with AI and machine learning to create predictive models on large datasets.
-
High-performance Analytics for Big Data: Optimized to handle large-scale datasets with high-speed processing capabilities, making it suitable for big data environments.
Who Should Use It?
-
Large enterprises and financial institutions: Perfect for businesses and organizations that need scalable predictive analytics and machine learning on complex and vast datasets.
Alteryx
Alteryx is a self-service data analytics platform that enables users to blend, analyze, and visualize data with minimal coding. It automates many aspects of data preparation, making it a valuable tool for faster insights.
Key Features:
-
Drag-and-drop Interface: Simplifies the analytics process with an intuitive drag-and-drop interface that doesn't require programming knowledge.
-
Integration with Machine Learning Tools: Seamlessly integrates with other machine learning tools, such as R and Python, to enhance predictive capabilities.
-
Automated Data Preparation: Automatically prepares data by performing tasks such as cleansing, transformation, and aggregation without manual intervention.
Who Should Use It?
-
Business analysts and marketing teams: Ideal for professionals who need a quick, automated solution to extract insights from diverse datasets.
RapidMiner
RapidMiner is an open-source platform designed to support data science, machine learning, and predictive modeling. Its focus on ease of use makes it accessible to users with varying levels of technical expertise.
Key Features:
-
Open-source, Customizable: Offers full access to source code, allowing customization and flexibility in how models are built and deployed.
-
Drag-and-drop Workflow: Provides an intuitive interface for creating workflows without writing code, making it accessible to both technical and non-technical users.
-
Supports Machine Learning Algorithms: Includes a wide variety of machine learning algorithms, from basic models to complex ensemble methods.
Who Should Use It?
-
Data scientists, analysts, and non-technical users: Suitable for anyone looking to build predictive models, regardless of their coding expertise.
H2O.ai
H2O.ai is an open-source machine learning platform offering robust AutoML capabilities. It's designed to enable rapid model development with an emphasis on scalability and performance.
Key Features:
-
AutoML for Fast Model Creation: Automates the process of model selection, training, and tuning, making it easy for users to create predictive models without extensive machine learning expertise.
-
Scalable Architecture for Big Data: Supports distributed computing for large datasets, making it ideal for big data environments.
-
Supports Deep Learning: Provides tools for deep learning, including neural networks, reinforcement learning, and other complex techniques.
Who Should Use It?
-
Data scientists and IT teams: Best suited for professionals in need of quick, high-performance model development for large-scale datasets.
Oracle Data Science
Oracle Data Science is a cloud-native platform for building and deploying machine learning models. It offers seamless integration with Oracle's ecosystem, making it a great choice for businesses already using Oracle products.
Key Features:
-
Cloud-native Integration: Fully integrated into the Oracle Cloud, facilitating easy access to data stored within the Oracle ecosystem.
-
Python and Jupyter Notebook Support: Supports Python, R, and Jupyter Notebooks, which are common tools used in data science and machine learning workflows.
-
Collaboration Tools: Provides tools for team collaboration on data science projects, allowing multiple team members to work on models and share insights easily.
Who Should Use It?
-
Enterprises using Oracle products: Ideal for organizations already using Oracle Cloud services and infrastructure to extend their analytics and machine learning capabilities.
Tableau
Tableau is a popular data visualization platform that also offers predictive analytics capabilities, allowing users to visually explore trends and forecast future outcomes.
Key Features:
-
Interactive Dashboards: Provides an intuitive interface for creating interactive dashboards that allow users to explore data and generate insights on the fly.
-
Built-in Predictive Modeling: Offers built-in forecasting and predictive models, allowing users to make informed decisions based on data patterns.
-
Integration with R and Python: Allows integration with R and Python, providing advanced analytical capabilities beyond the built-in features.
Who Should Use It?
-
Business analysts and decision-makers: Perfect for teams that need to visualize and interpret complex datasets to make strategic decisions.
TIBCO Spotfire is a data analytics and visualization tool that integrates predictive analytics with interactive reporting, helping businesses gain insights from data quickly.
Key Features:
-
Interactive Visualizations: Users can create powerful visual representations of their data that are interactive and easy to understand.
-
Machine Learning Capabilities: Spotfire includes built-in machine learning algorithms to support predictive analytics, trend analysis, and anomaly detection.
-
Scalable for Big Data: Designed to handle large datasets, making it a scalable solution for enterprises working with complex data.
Who Should Use It?
-
Enterprises and analysts needing advanced analytics and visualization: Best for companies that require both in-depth analytics and visually engaging reporting.
Qlik Sense
Qlik Sense is a self-service business intelligence tool that offers predictive analytics features with an associative data engine. It is designed to help users discover insights by analyzing data relationships and trends.
Key Features:
-
Associative Data Engine: The Qlik associative engine allows users to explore all possible data relationships, providing a deeper understanding of how different data points connect.
-
Predictive Modeling Tools: Includes built-in tools to develop and deploy predictive models and forecast trends.
-
Interactive Reporting: Features interactive dashboards that allow users to drill down into data and extract relevant insights.
Who Should Use It?
-
Business analysts and decision-makers: Ideal for professionals who need both advanced analytics and easy-to-use reporting tools for decision support.
Google Cloud AI Platform
Google Cloud AI Platform provides a set of tools for building, training, and deploying machine learning models at scale. Its integration with other Google Cloud services makes it ideal for businesses already embedded within the Google Cloud ecosystem.
Key Features:
-
Scalable Cloud Infrastructure: Provides on-demand infrastructure for handling large datasets and machine learning workloads.
-
Support for TensorFlow: Native integration with TensorFlow, one of the most widely used machine learning libraries.
-
Full Machine Learning Lifecycle Management: Supports all stages of the machine learning lifecycle, from data preprocessing to model deployment and monitoring.
Who Should Use It?
-
Data scientists and machine learning engineers: Best suited for teams that require advanced machine learning capabilities within a scalable cloud environment.
These tools provide a wide range of predictive analytics capabilities tailored to different industries and user requirements. Whether you're working with big data, machine learning models, or interactive visualizations, these platforms offer solutions to help businesses forecast trends and make data-driven decisions.
The Benefits of Predictive Analytics Tools
So, what’s the big deal? Why should anyone invest in a predictive analytics tool? I’ll share what I’ve personally gained:
-
Proactive Decision-Making: No more second-guessing or waiting for something to go wrong. I can now anticipate challenges and opportunities.
-
Efficiency Gains: Automation and accurate predictions have streamlined workflows and saved countless hours.
-
Personalized Experiences: Whether it’s customer engagement or employee satisfaction, personalization has become effortless.
-
Cost Savings: Predictive maintenance and risk mitigation have helped avoid expensive mistakes.
-
Improved Accuracy: Human error is no longer a bottleneck. Machine learning ensures predictions are precise and reliable.
Challenges in Using Predictive Analytics Tools
Of course, it hasn’t been all smooth sailing. There are challenges that I’ve had to tackle along the way:
-
Data Quality Issues: Poor data equals poor predictions. I’ve learned the hard way that clean and comprehensive datasets are crucial.
-
Model Interpretability: Some tools operate like a black box, and it can be frustrating not understanding why a prediction was made. That’s why I prioritize tools with explainable AI features.
-
Scalability: As my business grew, some tools struggled to keep up. Choosing scalable solutions from the start is a lesson I’ll never forget.
-
Skill Gaps: Predictive analytics tools can be intimidating for non-technical users. Tools with user-friendly interfaces bridge this gap but don’t eliminate it.
How Predictive Analytics Tools Work
To truly appreciate these tools, it’s essential to understand how they work. Here’s a simplified version of the process:
-
Data Collection: The tool pulls data from multiple sources—structured and unstructured.
-
Preprocessing: It cleans, organizes, and formats the data to make it analysis-ready.
-
Model Building: Using machine learning or statistical algorithms, the tool builds a predictive model based on historical data.
-
Prediction: The model analyzes new data to forecast future outcomes.
-
Insight Generation: Results are presented through dashboards, reports, or alerts, ready for action.
Real-World Applications
Predictive analytics isn’t just a fancy buzzword; it’s making a real difference in multiple industries. Here
market Growth of Predictive Analytics:
-
The global predictive analytics market was valued at approximately USD 18.89 billion in 2024 and is projected to grow at a compound annual growth rate (CAGR) of 28.3% from 2025 to 2030. Predictive analytics leverages historical data and statistical techniques to forecast future outcomes and behaviors.
Impact on Business Efficiency:
-
A survey by McKinsey found that companies using advanced analytics, including predictive analytics, are 5 times more likely to make faster decisions and 2.5 times more likely to perform better financially than their competitors.
Improvement in Marketing and Sales:
-
Predictive analytics can improve marketing ROI by 30-40%. By accurately forecasting customer behavior, businesses can target the right audience more effectively, leading to better conversion rates.
Fraud Detection in Finance:
-
In the finance industry, predictive analytics has led to a 50% reduction in fraud detection time for certain applications. 30-40% of financial institutions report using predictive tools for fraud prevention.
Healthcare:
-
Predictive models in healthcare have been shown to reduce readmission rates by 20% through early intervention and personalized care predictions. (Source: Journal of Healthcare Information Management)
Retail:
-
Retailers using predictive analytics for demand forecasting can experience up to a 30% improvement in inventory optimization and reduce stockouts by 20%.
Supply Chain Optimization:
-
Predictive analytics in supply chain management can improve demand forecasting accuracy by 15-20%, leading to better route optimization and lower operational costs.
Operational Efficiency:
-
Organizations using predictive maintenance can reduce downtime by up to 50%, leading to cost savings of around $630 billion annually in manufacturing sectors.
Evaluating and Choosing the Right Tool
When I started searching for a predictive analytics tool, I didn’t know where to begin. Here’s the checklist I used, which might help you too:
-
Integration Capabilities: Can it connect with existing systems like CRM, ERP, or cloud databases?
-
Scalability: Will it grow with my business?
-
Ease of Use: Does it require a data scientist to operate, or can non-technical users navigate it?
-
Customizability: Does it offer prebuilt models, or can I customize them for specific needs?
-
Cost Efficiency: What’s the ROI compared to the investment?
The Future of Predictive Analytics Tools
The world of predictive analytics is evolving rapidly. Here are a few trends I’m keeping an eye on:
-
AI-Driven Models: Faster, more accurate predictions with advanced machine learning algorithms.
-
Real-Time Predictions: Tools that offer live analytics and instant insights.
-
Edge Analytics: Predictions happening directly on devices, reducing the need for cloud processing.
-
Democratization of Analytics: Tools designed for non-technical users, making predictive analytics accessible to all.
-
Ethics and Transparency: There’s a growing emphasis on reducing bias and improving the interpretability of models.
Real-Life Success Stories
Nothing convinces me more than hearing real-world applications. For instance, a retail company reduced stockouts by 30% by using demand forecasting. Or a marketing team that increased email campaign engagement by predicting the optimal send time. These stories prove the transformative power of predictive analytics tools.
Making Better Decisions with Data
Here’s the truth: if you’re not using predictive analytics, you’re falling behind. Your competitors might already be using these tools to make better decisions with data, while you’re still using old methods. The missing out is real, and it’s something to worry about.
Predictive analytics tools help businesses make proactive decisions by using historical data and machine learning to predict future outcomes. They improve efficiency, accuracy, and personalization while reducing costs. Despite challenges like data quality, the benefits far outweigh the obstacles, making these tools essential for staying competitive in today’s fast-paced market.