Machine Learning in Finance: Predictive Analytics and Risk Assessment
Discover the intersection of finance and technology with "Machine Learning in Finance." Dive into the world of predictive analytics and risk assessment, where data-driven insights shape the future of financial decision-making.
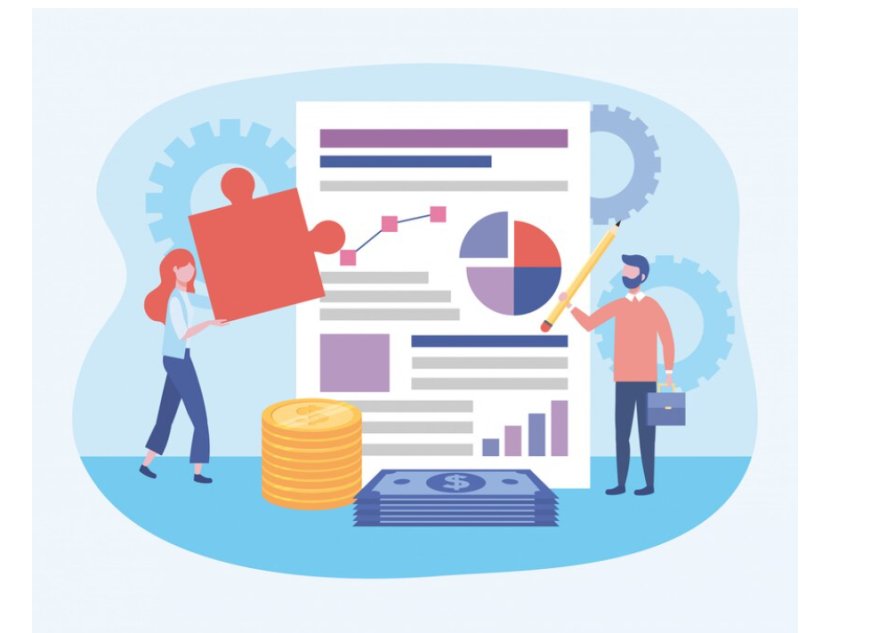
In the fast-paced and complex world of finance, accurate predictions and risk assessments are crucial for making informed decisions. Traditional financial models often fall short when dealing with vast amounts of data and rapidly changing market dynamics. However, with the advent of machine learning, the financial industry has witnessed a revolutionary transformation. In this blog, we will explore how machine learning is revolutionizing finance through predictive analytics and risk assessment.
Machine Learning
Machine learning is a subset of artificial intelligence that focuses on developing algorithms and statistical models, enabling computer systems to learn from data and improve their performance over time. By analyzing and identifying patterns within the data, machine learning algorithms can make predictions and decisions without explicit programming. It finds applications in various fields, such as natural language processing, image recognition, recommendation systems, and predictive analytics, driving innovations across industries and enhancing the capabilities of modern technology.
Importance of Machine Learning in Finance
-
Enhanced fraud detection and risk assessment.
-
Improved credit scoring and lending decisions.
-
Personalized financial recommendations for customers.
-
Automated trading and portfolio management.
-
Efficient fraud prevention and cybersecurity.
-
Data-driven insights for investment strategies.
-
Real-time market analysis and forecasting.
-
Customer sentiment analysis for better service.
-
Reduced operational costs and increased efficiency.
-
Minimized human errors in financial processes.
Key Applications in Finance: Predictive Analytics and Risk Assessment
-
Predicting stock market movements and price trends based on historical data.
-
Credit risk assessment for loan approvals and evaluating borrower creditworthiness.
-
Fraud detection and prevention in financial transactions and activities.
-
Customer churn prediction and retention strategies for financial institutions.
-
Forecasting cash flows and financial performance of companies.
-
Algorithmic trading and automated investment strategies.
-
Portfolio optimization to maximize returns while managing risk.
-
Real-time market sentiment analysis using natural language processing (NLP) techniques.
-
Anomaly detection to identify unusual patterns or behaviors in financial data.
-
Stress testing and scenario analysis to assess potential risks and impacts on financial institutions.
Predictive Analytics in Finance
Predictive Analytics in Finance is a powerful application of machine learning that leverages historical data and statistical algorithms to forecast future trends and outcomes in the financial sector. This blog explores the essence of Predictive Analytics, breaking it down into comprehensible segments. It delves into the use cases of Predictive Analytics in finance, encompassing stock market prediction for informed investment decisions, credit scoring, and loan approval to assess risk and enhance lending practices, as well as customer churn prediction for improved customer retention strategies. The blog further highlights the significance of popular machine learning algorithms, including Linear Regression for continuous value predictions, Decision Trees for creating interpretable models, Random Forests for ensemble learning and better accuracy, and Support Vector Machines for classification tasks. Overall, the blog provides valuable insights into how Predictive Analytics transforms the financial landscape and empowers decision-makers with data-driven foresight.
Risk Assessment in Finance
Risk assessment is a crucial aspect of finance, enabling organizations to make informed decisions while safeguarding their interests. This blog delves into the significance of risk assessment in the financial domain, highlighting the potential consequences of ignoring it. It then explores various types of financial risks, including credit risk, market risk, and operational risk, with insights into how each type impacts financial stability.
As technology advances, machine learning emerges as a powerful tool for risk assessment. The blog further discusses how machine learning techniques are employed in finance to enhance risk modeling and prediction. Specifically, it delves into credit risk modeling, which aids in evaluating borrowers' creditworthiness, and Value-at-Risk (VaR) prediction, a method to assess potential market losses. Additionally, the article examines the application of machine learning in fraud detection, enabling financial institutions to identify and prevent fraudulent activities effectively.
Overall, this blog provides a comprehensive overview of risk assessment in finance, emphasizing its significance, exploring different financial risk types, and showcasing how machine learning plays a pivotal role in improving risk assessment processes for better decision-making and risk mitigation.
Data Preparation and Feature Engineering
Data preparation and feature engineering are critical steps in the data science pipeline, especially in finance. In this process, data collection and sources play a vital role, ensuring that relevant and reliable data is acquired from various financial sources. Once the data is gathered, data cleaning and preprocessing techniques are applied to handle missing values, outliers, and inconsistencies, ensuring data quality and integrity. Subsequently, feature selection and importance methods are employed to identify the most relevant and informative features that contribute significantly to predictive models. This step helps in reducing dimensionality, improving model efficiency, and avoiding overfitting. By effectively managing data and engineering features, data scientists can build robust and accurate machine learning models that lead to valuable insights and informed decision-making in the dynamic and complex world of finance.
Model Training and Evaluation
Model Training and Evaluation is a fundamental process in machine learning, involving several key components to develop accurate and reliable predictive models. Firstly, the dataset is divided into three subsets: Training, Validation, and Test Sets (A). The training set is used to train the model, while the validation set helps fine-tune hyperparameters and optimize model performance, and the test set assesses the model's final generalization abilities.
Model Training with Machine Learning Algorithms is the core of building predictive models. Various algorithms like Decision Trees, Support Vector Machines, Neural Networks, and Gradient Boosting are employed based on the specific problem and data characteristics. Choosing the right algorithm is crucial to achieving optimal model performance.
Hyperparameter Tuning is a critical step to optimize the model's performance further. Hyperparameters are parameters set before training and can significantly impact the model's accuracy and generalization. Techniques like grid search or random search are used to find the best combination of hyperparameters.
In finance, Model Evaluation Metrics are tailored to assess the model's effectiveness in financial applications. Common metrics include Accuracy, Precision, and Recall (1), which measure the model's correctness and its ability to identify positive cases. The Receiver Operating Characteristic (ROC) curve and the Area Under the Curve (AUC) (2) provide insights into the model's trade-offs between true positive and false positive rates. Profit Curves (3) are specific to financial applications, showing the model's profitability at different classification thresholds.
Challenges and Limitations
Challenges and limitations are inherent in machine learning. Data quality, quantity, and bias pose significant challenges. Overfitting and underfitting affect model generalization. Interpreting complex models remains difficult. Ethical considerations arise from potential biases and privacy concerns. Additionally, selecting appropriate algorithms and hyperparameters is challenging, and demanding through experimentation and validation. Addressing these challenges is crucial to harnessing the true potential of machine learning while ensuring responsible and effective deployment.
Future Trends in Machine Learning in Finance
-
Advancements in Natural Language Processing (NLP) for Sentiment Analysis: In the future, Natural Language Processing (NLP) will play a crucial role in analyzing vast amounts of unstructured financial data, such as news articles and social media content, to gauge market sentiment. NLP algorithms will continuously improve in understanding complex language nuances, enabling more accurate sentiment analysis and better-informed investment decisions.
-
Reinforcement Learning for Algorithmic Trading: Machine learning's potential in finance will extend to reinforcement learning, where algorithms will learn optimal trading strategies through continuous interactions with financial markets. Reinforcement learning models will adapt to changing market conditions and uncover hidden patterns, paving the way for more sophisticated and adaptive algorithmic trading systems.
-
Expanding Use of Machine Learning in Financial Regulatory Compliance: Machine learning will revolutionize financial regulatory compliance by automating and enhancing risk assessment processes. Advanced algorithms will efficiently detect anomalies, fraudulent activities, and compliance breaches, helping financial institutions ensure adherence to regulations and significantly reduce operational risks.
Machine learning continues to transform various industries and aspects of our lives. With its powerful algorithms and models, it enables data-driven decision-making, personalization, and automation. However, ethical considerations and responsible practices must be at the forefront to address potential biases, privacy concerns, and societal implications. As the field evolves, understanding the nuances of machine learning and its applications becomes increasingly essential for individuals and organizations alike.