Ethical Considerations in Data Science: Privacy, Bias, and Fairness
Explore ethical considerations in data science, including privacy, bias, and fairness. Learn how to Guide these crucial issues in the world of machine learning.
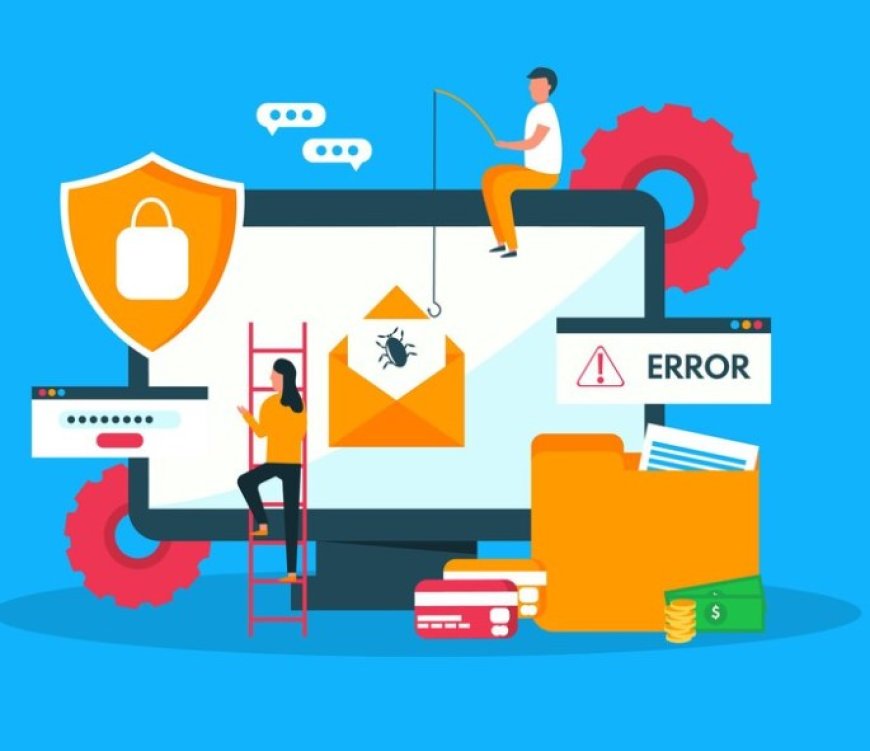
The introduction sets the stage for understanding the critical role of ethics in Data Science. It emphasizes the significance of ethical considerations in guiding the responsible use of data. Additionally, it provides a brief overview of the key pillars: Privacy, Bias, and Fairness, which form the foundation of ethical practices in data-driven decision-making processes. These elements collectively ensure that data science endeavors are conducted with transparency, integrity, and societal well-being in mind.
Importance of Ethical Considerations in Data Science
Ethical considerations in data science are of paramount importance. They ensure that data-driven decisions and technologies are developed and used in a responsible and socially acceptable manner. Ethical data practices safeguard individual privacy, prevent discrimination and bias, and promote fairness. Failing to address ethical concerns can lead to harmful consequences, such as privacy breaches and algorithmic discrimination. Moreover, adhering to ethical principles in data science builds trust with stakeholders and the public, ultimately fostering a more responsible and sustainable data-driven future.
Privacy
Privacy in data science refers to the protection of individuals' personal information and the responsible handling of data. It is of paramount significance as it safeguards individuals from potential harm, discrimination, and misuse of their data, while also maintaining trust in data-driven systems.
Data Collection and Consent: Ensuring privacy starts with transparent and informed data collection practices. Obtaining informed consent from individuals to collect their data is essential. This involves explaining the purpose, scope, and potential uses of the data to the individuals whose information is being gathered.
Data Storage and Security: Secure storage of data is crucial to protect it from unauthorized access, breaches, or leaks. Robust encryption, access controls, and secure infrastructure are essential components of data security. It helps in safeguarding sensitive information from falling into the wrong hands.
GDPR and Other Regulatory Frameworks: In the context of data privacy, compliance with regulations like the General Data Protection Regulation (GDPR) in Europe is vital. Such regulatory frameworks establish guidelines for the responsible handling of personal data, including data subjects' rights, data protection officers, and data breach notifications. Adherence to these regulations ensures that data science practices align with legal and ethical standards, reducing the risk of privacy violations.
Bias
Bias in data refers to the presence of systematic errors that skew the representation or analysis of information. It can result from various factors, such as the way data is collected, processed, or interpreted. Understanding the sources and nature of bias is crucial for ethical data science.
There are different types of bias in data, including selection bias (arising from non-random sampling), sampling bias (caused by incomplete or unrepresentative data), and algorithmic bias (stemming from biased training data or flawed algorithms). Identifying these types is essential for pinpointing potential problems.
Bias in data can have significant consequences, leading to unfair or discriminatory outcomes in decision-making processes. It can affect individuals and communities, perpetuating inequalities and reinforcing stereotypes. Recognizing the impact of bias underscores its ethical importance.
To address bias, data scientists must adopt various strategies, such as careful data preprocessing, diverse and representative data collection, and the development of algorithms that reduce bias. Regular audits and transparency in the modeling process are essential to ensure data-driven decisions are more equitable and reliable.
Fairness
Fairness in data science refers to the equitable treatment of individuals and groups in the collection, analysis, and utilization of data. It ensures that the outcomes and decisions made through data-driven processes do not discriminate or disadvantage specific demographics, and that all individuals have an equal opportunity.
Challenges in Achieving Fairness: Achieving fairness is often complex due to inherent biases in data, algorithms, and historical disparities. Challenges include identifying biases, defining fairness metrics, and balancing competing interests, such as accuracy versus fairness.
Addressing Bias in Algorithms: To promote fairness, data scientists need to mitigate bias in algorithms. Techniques like re-sampling, re-weighting, and fairness-aware machine learning can help reduce bias. It's essential to adjust algorithms without introducing new forms of bias.
Evaluating and Monitoring Fairness: Continuous evaluation and monitoring of models and data are critical. Regularly assess the impact of algorithms on different demographic groups and adjust as necessary. Monitoring ensures fairness is maintained throughout the data science lifecycle.
Ethical Guidelines and Frameworks
Industry Standards: Prominent organizations such as IEEE (Institute of Electrical and Electronics Engineers) and ACM (Association for Computing Machinery) have developed industry standards that outline ethical principles for data science. These standards offer a foundational framework for ethical conduct in data-related professions.
Ethical AI Principles: Leading tech companies like Google and IBM have established their own ethical AI principles. These principles address issues like fairness, transparency, and accountability in AI and data science applications. These principles serve as a valuable reference for aligning projects with ethical considerations.
Implementing Ethical Guidelines in Data Science Projects: The practical application of ethical guidelines is essential. Data scientists and project teams must actively incorporate these guidelines into every phase of their projects, from data collection to model deployment. This implementation ensures that ethical considerations are not just theoretical concepts but integral parts of real-world data science initiatives, promoting responsible and trustworthy practices.
Ethical Frameworks
Ethical frameworks provide essential guidance in navigating the complex ethical landscape of data science. Utilitarianism assesses the consequences of actions, focusing on maximizing overall happiness or benefit while minimizing harm. Deontological ethics emphasizes duty and adherence to moral principles, regardless of outcomes. Virtue ethics centers on cultivating virtuous character traits. Each framework offers unique perspectives on what is right and ethical.
Applying these ethical frameworks to data science involves evaluating the consequences, principles, and virtues relevant to the field. For example, in data collection, utilitarianism may weigh the benefits of data-driven insights against privacy concerns. Deontological ethics could require strict adherence to informed consent protocols. Virtue ethics may emphasize the importance of integrity and transparency in data handling. By considering these frameworks, data scientists can make more ethically informed decisions in their work.
Best Practices
Establishing clear and comprehensive ethical guidelines is essential in guiding data science projects. These guidelines should encompass principles for data collection, storage, and usage, emphasizing the importance of privacy, fairness, and bias mitigation. They should also address informed consent and outline procedures for handling sensitive information.
Ensuring that every member of the data science team is well-versed in ethical considerations is crucial. Conduct regular training sessions on privacy, bias detection and mitigation, and fairness in algorithms. Foster a culture of open discussion where team members feel comfortable raising ethical concerns.
Implementing routine audits and assessments helps monitor adherence to ethical guidelines. These evaluations should encompass the entire data lifecycle, from collection to model deployment. Assessments should not only identify potential privacy breaches or biases but also measure the impact of algorithms on different demographic groups, ensuring fairness and inclusivity. Regularly updated assessments ensure ongoing ethical compliance and continuous improvement in data science practices.
Future Trends and Considerations
Emerging Ethical Issues in Data Science
As data science continues to evolve, new ethical challenges are likely to arise. These might include issues related to deep learning and neural networks, as they become more complex and opaque. Additionally, the increasing use of personal data in healthcare, finance, and other sectors will demand stringent ethical safeguards to protect individuals.
Technological Advancements and Ethical Implications
Advancements in technology, such as artificial intelligence, automation, and the Internet of Things (IoT), will bring about ethical considerations in data science. Questions about the ethical use of AI in decision-making, the potential for job displacement, and the responsible handling of IoT-generated data will be at the forefront of ethical discussions in the field. Data scientists will need to adapt to these changes and ensure that their work aligns with evolving ethical standards.
The ethical considerations in data science, encompassing privacy, bias, and fairness, are pivotal in ensuring responsible and trustworthy data-driven practices. Privacy safeguards individuals' rights, bias mitigation promotes equity, and fairness upholds justice. A strong call to action is essential, urging data scientists, organizations, and policymakers to adhere to ethical guidelines, foster transparency, and continuously work towards a data ecosystem that respects these fundamental principles, ultimately benefiting both individuals and society as a whole.