The Ethics of Data Analytics: Privacy, Responsibility, and Future Considerations
Explore the ethics of data analytics in this insightful discussion. Delve into privacy, responsibility, and future considerations surrounding data analysis practices.
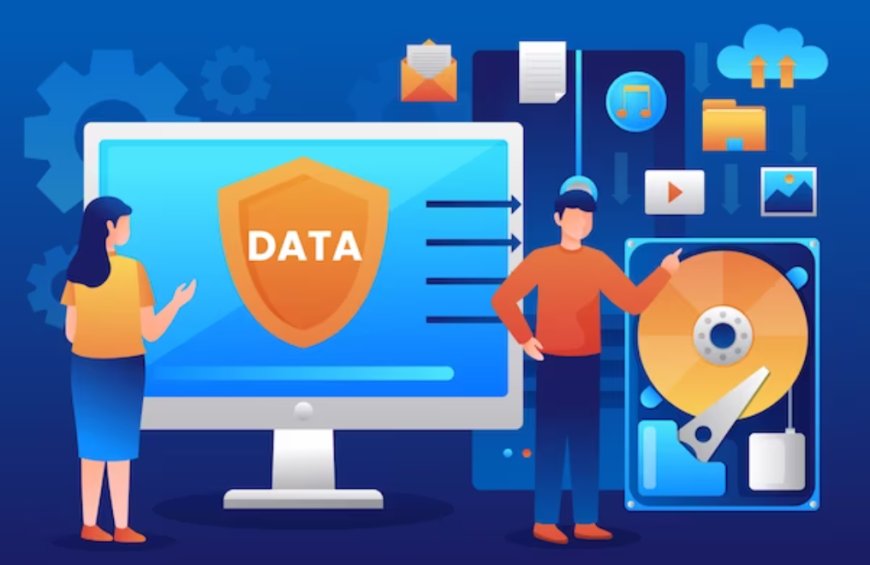
Data analytics is the process of examining vast datasets to extract valuable insights, making it a cornerstone of modern decision-making. However, its rapid integration into society has ignited a crucial discussion on data ethics. Before we embark on this exploration, let's define data analytics and underscore the growing significance of ethical considerations in this data-driven age.
The Data Analytics Revolution:
Data analytics, the process of analyzing vast datasets to unearth valuable insights, stands as a transformative force in modern society. It has reshaped the way we live and work, permeating various aspects of our daily lives. From personalized marketing recommendations that guide consumer choices to groundbreaking medical discoveries that save lives, data analytics has unleashed its potential across diverse sectors. This technological revolution has ushered in a new era where data-driven decision-making has become the norm. However, this power has also brought ethical considerations to the forefront, prompting a crucial discourse on data ethics.
Privacy Concerns in Data Analytics
Privacy is a paramount concern in the realm of data analytics. Within this domain, several significant privacy issues demand attention and careful management.
Firstly, the collection of personal data without informed consent raises ethical dilemmas. Data analytics often involves the aggregation of vast amounts of information from individuals, which can include sensitive data. Ensuring that users are aware of and consent to the collection and usage of their data is crucial to respecting their privacy.
Secondly, data anonymization and de-identification methods are essential to safeguarding privacy. However, they are not foolproof, and there's always a risk of re-identifying individuals from supposedly anonymous data. This underscores the need for robust privacy-preserving techniques.
Moreover, the ever-present threat of privacy breaches and data leaks poses a significant concern. High-profile cases have exposed the vulnerability of personal information, emphasizing the importance of stringent security measures to protect individuals' privacy.
Illustrating these concerns with real-world examples highlights the gravity of privacy issues in data analytics, emphasizing the urgent need for responsible data handling practices.
Ethical Frameworks in Data Analytics
Ethical decision-making in data analytics is often guided by various philosophical frameworks. These frameworks help professionals navigate complex ethical dilemmas. Three prominent ethical theories are commonly applied in the context of data analytics:
-
Utilitarianism assesses the ethicality of actions based on their consequences. In data analytics, this means considering whether a particular data practice maximizes overall happiness or utility. It may involve weighing the benefits of data analysis against potential harm to individuals' privacy or other ethical concerns.
-
Deontology emphasizes the inherent rightness or wrongness of actions, regardless of their outcomes. In data analytics, this framework urges professionals to adhere to ethical principles and rules, such as obtaining informed consent for data collection and respecting individuals' autonomy, regardless of the potential benefits.
-
Virtue ethics focuses on the character of individuals and organizations. It encourages cultivating virtues like honesty, integrity, and transparency in data analytics practices. Virtue ethicists argue that ethical behavior arises from virtuous character rather than strict adherence to rules or outcomes.
In practice, data analytics professionals often combine elements of these ethical theories to make decisions. They consider the consequences of their actions (utilitarianism), adhere to ethical principles (deontology), and cultivate virtuous character traits (virtue ethics) to ensure responsible and ethical data practices.
By applying these ethical frameworks, data analytics professionals can navigate the complex ethical terrain of their field and make informed, morally sound decisions.
Legal Frameworks and Regulations:
To address mounting ethical concerns in data analytics, a range of legal frameworks and regulations have been instituted globally. These laws aim to protect individuals' privacy, promote transparency, and hold organizations accountable for their data practices.
-
GDPR: Enforced in May 2018, the General Data Protection Regulation establishes stringent standards for personal data collection, processing, and storage. It grants individuals greater control over their data, including the right to access, correct, and delete it.
-
CCPA: Implemented in January 2020, the California Consumer Privacy Act empowers California residents with greater control over their personal information. It grants them the right to know what data is being collected and how it's used, as well as the ability to opt out of data sales.
These legal frameworks serve as both legal obligations and ethical benchmarks for data handling. Noncompliance can result in significant penalties, reputational harm, and a loss of customer trust. Staying informed about and compliant with these regulations is crucial for both organizations and individuals to uphold data ethics.
Responsible Data Analytics Practices:
Responsible data analytics practices form the bedrock of ethical and trustworthy data handling. These practices encompass a spectrum of measures aimed at ensuring data is collected, processed, and used in a manner that respects individual privacy and societal values.
-
Ethical Guidelines Implementation: Organizations should establish clear policies and principles for data collection and analysis, emphasizing transparency, fairness, and responsible data handling from inception to disposal.
-
Data Governance and Accountability: Clearly delineated responsibilities within an organization for data-related decisions ensure that ethical considerations are integral to data practices. Data governance policies uphold control over data quality, integrity, and security.
-
Ethical Decision-Making Training: Data analysts should receive training that incorporates ethical considerations into their work. This equips them to make choices aligned with ethical principles, fostering responsible data analysis.
By embracing these practices, organizations not only enhance their trustworthiness but also contribute to a broader ethical landscape in our data-driven world. They can harness the power of data analytics while fulfilling their ethical responsibilities to individuals and society.
The Future of Data Ethics:
As data analytics advances, so too will the ethical considerations. Emerging technologies like artificial intelligence and machine learning bring their own ethical challenges, including algorithmic bias and the need for explainable AI.
Addressing issues of algorithmic bias, transparency, and fairness will be paramount as AI and machine learning continue to play a central role in data analytics. Ethical AI frameworks and guidelines will become standardized and integrated into development processes.
With the rise of data breaches and concerns over personal data, there will be a growing emphasis on enhancing data privacy. Stricter regulations and consumer demands for greater control over their data will drive businesses and organizations to prioritize privacy by design.
Navigating the complex web of international data laws and ensuring that ethical data practices extend globally will become increasingly challenging and important.
As technologies like blockchain and quantum computing gain prominence, new ethical questions will arise. Ensuring the ethical use of quantum computing power and securing blockchain-based data will be critical.
Organizations may appoint dedicated data ethics officers or teams responsible for ensuring ethical data practices throughout the data lifecycle, from collection to analysis and sharing.
The general public will become more informed and vigilant about data privacy and ethics. This will drive demand for greater transparency and ethical behavior from both businesses and governments.
Data ethics is not static. Ongoing adaptation will be essential to address emerging challenges and technologies. This adaptability is critical in maintaining trust and ethical standards in data analytics.
This exploration of the ethics of data analytics has highlighted critical aspects. We've discussed privacy concerns, the importance of responsibility, and various ethical frameworks. Regulations and case studies have shed light on real-world implications. Looking ahead, the field faces evolving challenges, underscoring the ongoing significance of ethics in data analytics. Encouraging responsible data practices remains essential to ensure the ethical use of data and maintain public trust in this increasingly influential domain.