The Essential Applications of Data Science for Businesses
How data science empowers businesses by enhancing decision-making, optimizing operations, and driving customer engagement for sustainable growth.
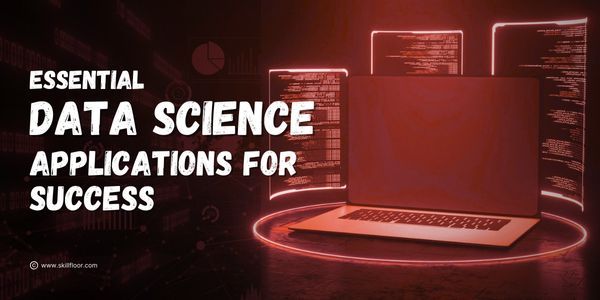
Over the years, I have personally witnessed the transformative power of data in business. By monitoring customer patterns, habits, and preferences and making focused, intelligent adjustments, businesses may enhance engagement, create happier consumers, and eventually boost revenue.
Data science is more than just statistics; it's about gaining insights that help companies stay competitive, increase efficiency, and provide better customer service. Predictive technologies help businesses foresee trends, maximize resources, and make more informed decisions that lead to actual development.
Businesses that wish to succeed must use data science. Studies show that data-driven decision-making can increase productivity by 5% and profitability by 6% for companies that adopt this approach (SharpGrid). Data insights aid small businesses, industry professionals, and customers by reducing waste, increasing customer happiness, and optimizing resources. This builds confidence and trust over time.
What is Data Science?
Data science is transforming a lot of unprocessed data into insightful knowledge that may inform business choices. To find patterns and trends in data and assist organizations in forecasting results and making more informed decisions, it integrates expertise from domains such as statistics, programming, and machine learning.
Data science has several benefits for organizations. By making data-driven decisions, it enables them to stay competitive, optimize operations, and comprehend client demands. Businesses employ data science to improve customer experiences, reduce wasteful spending, and foster audience trust, all of which contribute to more solid long-term partnerships.
Key Components of Data Science
1. Data Collection
Accurate and pertinent data collection is crucial to data science. Information from a variety of sources is gathered in this stage, serving as the basis for all further research and insights.
2. Data Cleaning
Data must be cleansed before analysis. This process guarantees that data utilized in data science initiatives is accurate and devoid of duplicates, producing trustworthy outcomes.
3. Data Exploration
Data scientists analyze trends and patterns in datasets in this stage. Businesses may better comprehend their data and find hidden insights by using data analysis.
4. Feature Engineering
For machine learning, this entails turning unprocessed input into valuable characteristics. Through the highlighting of important data points, feature engineering improves the accuracy of models in data-driven processes.
5. Model Building
Data scientists use algorithms to develop machine learning models in this case. Businesses may make predictions using data science's predictive modeling by using trends in past data.
6. Model Evaluation
Models are assessed after construction to make sure they are successful. This stage in data science applications verifies that the model generates precise and significant outcomes for practical use.
Importance of Data Science in Business
-
Enhanced Decision-Making: Businesses may use data science to create well-informed judgments based on data analysis, which results in plans supported by facts rather than conjecture and more precise and assured commercial decisions.
-
Personalized Customer Experience: Data Science Impact on Business enables companies to improve customer satisfaction and cultivate enduring loyalty by customizing products, services, and marketing campaigns to individual preferences through the analysis of consumer data.
-
Optimized Operations: Data science analytics helps businesses find inefficiencies, optimize processes, and cut expenses. Effective resource allocation and increased production are made possible by operational insights derived from data.
-
Market Insights: By using data-driven insights, companies may better understand consumer behavior, competition activity, and market trends. This enables businesses to swiftly adjust and maintain their lead in a cutthroat market.
-
Risk Management: By recognizing trends that point to fraud, default, or other dangers, data science models help businesses anticipate possible risks and take preventative action to protect their assets.
-
Revenue Growth: Businesses may estimate demand, enhance product offers, and optimize pricing by utilizing predictive data analysis. In addition to satisfying client demands, this strategy increases profitability.
Why Data Science Matters in Business
From startups to well-known corporations, I have seen the genuine impact that data science has on organizations. Businesses may improve engagement and propel development in ways that are truly satisfying by using data to understand consumer behaviors and preferences.
The goal of data science is to transform data into insights that support the success of enterprises, not just to collect information. Businesses may improve customer satisfaction and operational efficiency by utilizing machine learning and predictive analytics. Leading businesses like Amazon and Netflix demonstrate how data-driven tactics increase revenue, productivity, and customer happiness, proving that data science is crucial for sustained success and expansion.
By depending on data-driven insights rather than conjecture, data science promotes trust. Businesses are better able to respond to the requirements of their customers when they make open, fact-based choices. This enhances their industry image for honesty and accountability while also boosting client confidence.
Key Applications of Data Science that Can Empower Businesses
1. Customer Insights and Personalization
-
Understanding Customers: Businesses may use data to identify consumer preferences, buying trends, and habits, which helps them develop strategies that meet actual requirements and forge closer, more meaningful bonds with their clients.
-
Personalized Recommendations: Data-driven insights make purchasing and browsing more relevant by enabling personalized recommendations, such as how Netflix suggests movies you'd like or how Amazon emphasizes things you're interested in.
-
Customer Segmentation: By classifying clients based on shared characteristics, companies may develop targeted advertising campaigns that appeal to each market niche, boosting engagement and giving each encounter a more unique and significant sense.
-
Predicting Customer Behavior: Applications of Data Science allow businesses to provide timely promotions, reminders, or help by using data insights to predict client activities. Recognizing needs improves client happiness and fosters loyalty.
2. Operational Efficiency and Cost Optimization
-
Predictive Maintenance: Businesses may anticipate equipment maintenance requirements by utilizing data science insights, which lowers the cost of repairs and unplanned failures. By reducing downtime, this proactive method maintains efficient and on-time production.
-
Inventory Management: Businesses can maintain appropriate inventory levels by using data science to analyze trends of demand. This lower holding costs, avoids stockouts and overstocking, and guarantees that items are available when customers need them.
-
Process Optimization: By identifying workflow inefficiencies, data science tools help businesses optimize their operations. This improvement boosts overall productivity in addition to saving money and time.
-
Resource Allocation: Businesses may more efficiently manage resources by determining where money and labor should be concentrated thanks to data science insights. This focused utilization of resources improves departmental cost savings and operational efficiency.
3. Risk Management and Fraud Detection
-
Fraud Detection: Algorithms using data science look at transaction data to find trends that might indicate fraud. In banking and e-commerce, where fraud prevention safeguards significant assets, this proactive strategy is particularly beneficial.
-
Risk Assessment: Applications of Data Science allow businesses to assess possible risks using data insights, helping make informed decisions. For instance, lenders analyze history to determine credit risk, while insurers use data-backed evaluations.
-
Anomaly Detection: Data science keeps organizations safe and better prepared by enabling prompt reactions to hazards like operational interruptions or cybersecurity attacks by identifying anomalous activity.
-
Compliance Monitoring: By monitoring regulatory compliance, data science tools help make sure businesses are meeting industry requirements. Businesses maintain adherence, avoiding expensive penalties and preserving confidence by keeping an eye out for inconsistencies.
4. Product Development and Innovation
-
Market Research and Trend Analysis: Businesses use data science insights to monitor consumer preferences and market trends, which helps them develop goods that meet modern needs and increase their chances of success.
-
A/B Testing for Product Features: Businesses test different versions of their products using data science studies to see which aspects people respond to the most. New goods are guaranteed to successfully satisfy client expectations thanks to this data-backed strategy.
-
Feedback Analysis: Data science tools look for opportunities for development by analyzing surveys, social media, and reviews. Real client feedback helps businesses improve their goods for increased customer loyalty and pleasure.
-
Demand Forecasting: Data science uses historical sales, seasonality, and market changes to forecast future product demand. By taking a proactive strategy, companies may better manage resources, avoid stockouts, and increase customer satisfaction.
5. Marketing Optimization and Campaign Targeting
-
Audience Segmentation: Businesses may use data science to establish targeted client groups according to interests, actions, or demographics. Ad relevance is increased by this focused strategy, which enhances engagement and forges closer ties with clients.
-
Campaign Performance Prediction: Applications of Data Science enable marketers to use historical data to forecast campaign outcomes. By improving techniques and budget distribution, this approach maximizes return on investment.
-
Real-Time Ad Personalization: Businesses may utilize data insights to customize advertisements on the fly, displaying items according to user activities in real time. This is particularly useful for e-commerce sites looking to boost conversions.
-
Customer Journey Mapping: By monitoring every phase of the customer journey, data science assists businesses in comprehending how clients go via the sales funnel. More efficient marketing outreach and better touchpoints are made possible by this knowledge.
6. Sales Forecasting and Revenue Optimization
-
Sales Prediction: Data science technologies may predict future sales patterns by evaluating historical sales data, which aids companies in effectively planning and allocating resources to fulfill anticipated demand.
-
Churn Prediction: Businesses may reach out to "at-risk" consumers with tailored offers that improve retention by using data insights to spot early indicators of possible client departure.
-
Revenue Optimization: Data science assists companies in examining elements that affect sales, such as price or demand patterns, and informing tactics that optimize profits, particularly during periods of high demand.
-
Customer Lifetime Value Prediction: By using data insights, businesses may calculate the lifetime worth of a client, enabling customized engagement tactics that improve long-term profitability and customer loyalty.
7. Customer Service and Support Automation
-
Chatbots and Virtual Assistants: Chatbots are used by many companies to swiftly respond to basic consumer inquiries, freeing up support staff to concentrate on more complicated problems that require individualized care.
-
Sentiment Analysis: Applications of Data Science allow companies to proactively address issues by analyzing input from social media and reviews, improving customer satisfaction.
-
Predictive Customer Support: Artificial Intelligence helps businesses study past interactions to predict customer needs, offering timely, proactive support that enhances the overall service experience.
-
Automated Follow-Up: Automated systems can follow up after support conversations to make sure problems are fixed completely. This extra step demonstrates a commitment to continuous assistance and boosts client satisfaction.
8. Supply Chain Management and Logistics
-
Demand Forecasting: Businesses may more effectively and sustainably run by using data insights to forecast demand with accuracy, which simplifies supply chain planning and reduces waste.
-
Route Optimization: By using data to determine the most effective delivery routes, logistics organizations may cut down on travel time and fuel expenditures, resulting in quicker deliveries and cheaper charges.
-
Supplier Relationship Management: Businesses may establish more reliable partnerships by assessing supplier reliability, negotiating better contracts, and anticipating supply chain interruptions through the analysis of supplier data.
-
Inventory Replenishment: Businesses may ensure product availability for consumers and cut down on excess inventory expenses by using data-driven inventory tracking to accurately restock stock as needed.
Data Science Workflow and Methodologies in Business
1. Problem Definition
Define the business challenge precisely. Understanding the precise objectives—such as increasing customer loyalty or cutting costs—directs the analytical strategy in many applications of data science.
2. Data Collection and Preparation
Collect information from several sources, then organize and clean it to guarantee its quality for analysis. For corporate applications of data science, where precise data produces more dependable outcomes, this is a critical step.
3. Exploratory Data Analysis (EDA)
By using EDA to find patterns and trends, you may develop hypotheses, improve your analytical methodology, and make data more useful.
4. Modeling and Testing
Test data insights using statistical or machine learning models, then compare them to determine which one best suits the business requirement.
5. Model Deployment
Make the model easily accessible to decision-makers by integrating it into corporate processes through dashboards, applications, or automated workflows.
6. Monitoring and Maintenance
Maintain the model's correctness and relevance by regularly assessing its performance and making adjustments as business requirements change.
Data Science vs. Data Analytics vs. Machine Learning: Understanding the Differences
Aspect |
Data Science |
Data Analytics |
Machine Learning |
Definition |
Field that combines methods to extract insights and solve complex problems using data |
Focuses on interpreting existing data for actionable insights |
Subset of AI where systems learn from data to make predictions |
Objective |
Generate actionable insights and drive decision-making across various domains |
Analyze historical data to understand trends and support specific business decisions |
Develop models that predict or automate tasks without explicit programming |
Methods Used |
Statistics, mathematics, data engineering, machine learning |
Statistical analysis, reporting, visualization |
Algorithms like decision trees, neural networks, and regression |
Typical Tools |
Python, R, SQL, Hadoop |
Excel, Tableau, Power BI |
TensorFlow, Scikit-learn, PyTorch |
Application Examples |
Customer segmentation, recommendation systems, fraud detection |
A/B testing, performance tracking, customer behavior analysis |
Image recognition, predictive text, recommendation engines |
Expertise Required |
Combines knowledge of statistics, programming, domain expertise |
Strong analytical skills, understanding of data patterns and business intelligence |
Advanced math, statistical modeling, and programming skills |
Future Trends in Data Science
Automated Machine Learning (AutoML): Automating processes such as feature engineering, data preparation, and algorithm selection to streamline model creation and increase accessibility to data science.
AI Ethics and Explainability: Encouraging trust and responsible data usage by placing a strong emphasis on developing ethical AI and building transparent, explicable models.
Real-Time Analytics: Using real-time insights from streaming data to facilitate speedier decision-making in industries including IoT, banking, and e-commerce.
Edge Computing Integration: For quicker analysis, data processing should be moved to the "edge"—that is, closer to the data sources—which is particularly helpful in Internet of Things applications.
Interdisciplinary Data Science: Combining data science with domain-specific knowledge to address industry-specific problems, such as environmental monitoring and healthcare.
By transforming data into strategic, actionable insights, data science plays a transformational role in business. Businesses may improve consumer interaction, streamline processes, and make well-informed choices that foster resilience and growth through a variety of applications of data science. These technologies, which range from customized advice to predictive maintenance, let organizations quickly adjust to shifting market conditions. Building enduring relationships with clients while generating value and increasing productivity are all made possible by adopting the applications of data science. Data science is the secret to long-term, forward-thinking company success; it is more than just analysis.