Exploring the Role of Machine Learning in Data Science
Discover the pivotal role of Machine Learning in Data Science. Explore its applications, concepts, and impact on data-driven decision-making in various industries.
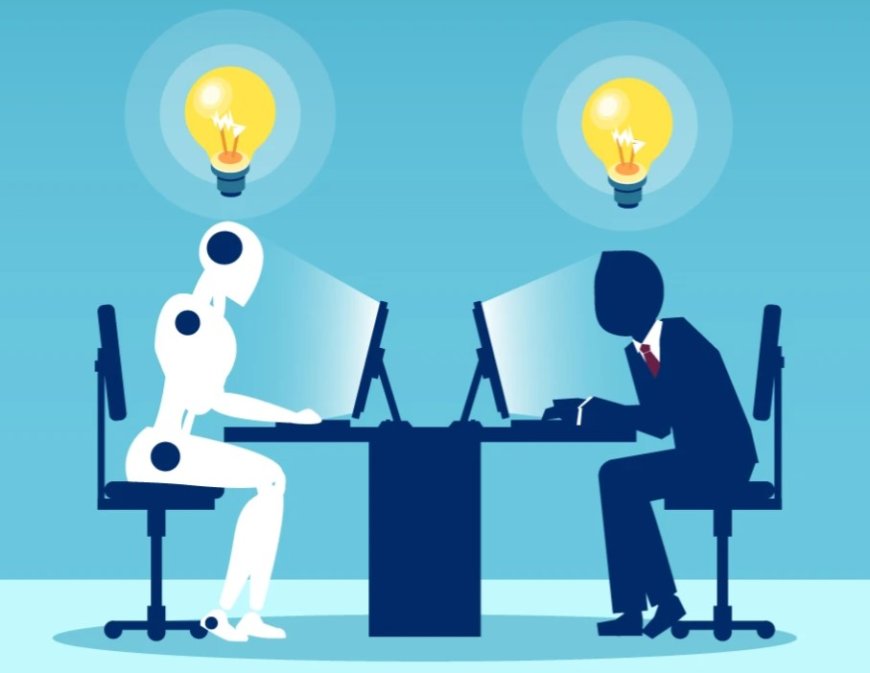
In the ever-evolving landscape of data science, one technology stands out as a driving force behind transformative insights and innovation: machine learning. As data continues to grow in volume and complexity, the ability to extract meaningful patterns and knowledge from it becomes increasingly vital. Machine learning, a subset of artificial intelligence (AI), empowers data scientists with the tools and techniques to do just that. In this exploration of the role of machine learning in data science, we'll delve into the fundamental concepts, applications, and the profound impact this technology has on our ability to unlock the hidden potential within data.
Data Science:
Data science is the art of extracting insights from complex datasets, offering a multidisciplinary toolkit to empower informed decisions. It encompasses a spectrum of techniques and methodologies for transforming raw data into actionable knowledge. By gathering, cleaning, analyzing, and visualizing data, data scientists uncover hidden trends and correlations. This holistic approach melds statistical analysis, domain expertise, and technological proficiency, acting as the bridge that connects raw data to meaningful outcomes.
Machine Learning:
At the core of data science lies the dynamic duo of data science and machine learning. While data science orchestrates the processes to extract insights, machine learning amplifies its capabilities. Machine learning, a subset of artificial intelligence, equips data science with predictive prowess and the ability to decipher complex patterns that elude conventional methods. Together, they form an extraordinary partnership, enabling businesses to anticipate trends, personalize experiences, optimize operations, and unearth concealed insights. As machine learning continues to evolve and absorb advanced techniques, this collaboration promises a future where data-driven decisions revolutionize industries and innovations.
Collaborative Insights: How Machine Learning Enhances Data Science
Predictive Modeling: Machine learning algorithms excel in analyzing historical data to predict future outcomes with exceptional accuracy. This predictive power empowers businesses to forecast sales, customer behavior, market trends, and more, allowing them to proactively adapt strategies.
Personalization: Through machine learning, data scientists can craft models that customize recommendations and experiences to individual preferences. This personalized approach enhances customer engagement and fosters loyalty across diverse industries.
Pattern Recognition: Machine learning stands out in identifying intricate patterns and anomalies within data, which may pose challenges for traditional statistical methods. This capability proves invaluable for tasks like fraud detection, quality control, and outlier identification.
Optimization: Machine learning can analyze intricate datasets to optimize processes and decision-making. From supply chain management to resource allocation, machine learning algorithms assist businesses in identifying the most efficient paths forward.
Automation: By automating routine tasks, machine learning liberates data scientists to concentrate on the more strategic aspects of analysis, resulting in heightened productivity and expedited insights delivery.
Challenges: Ethical Considerations and Bias
The intricate interplay between data science and machine learning demands careful attention to ethical considerations and the potential for bias. As these technologies play an increasingly pivotal role in decision-making, ensuring fairness and unbiased outcomes becomes paramount.
Unveiling Bias: Machine learning models learn from historical data, and if that data contains biases, the models can inadvertently perpetuate those biases. This can lead to unfair or discriminatory decisions that reinforce societal inequalities. Recognizing and mitigating such bias is crucial for ensuring equitable outcomes.
Ethical Dilemmas: As machine learning algorithms impact real-world scenarios, ethical dilemmas arise. Consider self-driving cars making split-second decisions or loan approval algorithms affecting financial futures. Striking the right balance between automation and human intervention becomes a challenge to prevent unintended consequences.
Transparency and Accountability: The complexity of machine learning models can make it difficult to explain how decisions are reached. Ensuring transparency in the decision-making process is essential for building trust with stakeholders and regulators. It also facilitates accountability in case of errors or bias.
Fairness and Inclusivity: Achieving fairness goes beyond merely eliminating bias. It requires actively designing models to be inclusive and considerate of diverse user groups. This involves addressing not only the biases present in data but also the potential biases that may arise during model training.
Data Quality and Representation: Ethical considerations extend to the quality and representation of data used to train models. Biased, incomplete, or skewed datasets can lead to skewed insights. Organizations must critically evaluate data sources and implement strategies to improve data quality.
The Path Forward: Tackling these ethical challenges requires a multifaceted approach. Data scientists and machine learning engineers must be proactive in identifying potential biases, applying debiasing techniques, and regularly auditing models for fairness. Collaboration between domain experts, ethicists, and technical teams is crucial in navigating these complexities.
The Future of Data Science and Machine Learning
The future of data science and machine learning holds immense promise. As these fields continue to evolve, advanced techniques like deep learning and reinforcement learning will unlock insights from diverse data sources. The collaboration between data science and machine learning will drive innovation, enabling businesses to make proactive decisions based on predictive analytics, recognize intricate patterns, and automate processes. However, addressing ethical concerns, ensuring model transparency, and guarding against bias will be pivotal for a responsible and impactful integration of these technologies in shaping our data-driven future.
The Future Potential
The journey of data science and machine learning is an ever-evolving expedition into uncharted territories of knowledge and innovation. In the years ahead, several key trends are set to reshape the landscape:
-
Advanced Techniques: Deep learning, a subset of machine learning, is set to further expand its influence. Neural networks with intricate architectures will enable the extraction of insights from more complex data types, such as unstructured text and images.
-
Interdisciplinary Integration: The boundaries between data science and other fields, including natural language processing, computer vision, and robotics, will continue to blur. This convergence will lead to even more powerful applications and cross-disciplinary collaborations.
-
Edge Computing: The deployment of machine learning models on edge devices, like smartphones and IoT devices, will become more prevalent. This will enable real-time, localized decision-making without relying solely on cloud-based processing.
-
Ethics and Governance: As data and machine learning become increasingly intertwined with society, the importance of ethical frameworks and governance mechanisms will grow. Stricter regulations and accountability measures will be put in place to ensure responsible AI and data practices.
-
Human-AI Collaboration: The focus will shift towards augmenting human capabilities with AI, creating a symbiotic relationship where machines assist humans in decision-making and problem-solving across various domains.
-
Explainable AI: There will be a greater emphasis on making machine learning models interpretable and explainable. This transparency is vital for building trust and understanding model decisions.
-
Bias Mitigation: Continued efforts to identify and mitigate bias in machine learning models will be crucial. Fairness-aware algorithms and comprehensive auditing processes will become standard practice.
-
Data Privacy: With heightened awareness of data privacy concerns, innovations in privacy-preserving techniques, like federated learning and differential privacy, will become integral in the data science and machine learning toolkit.
-
Responsible AI Adoption: Organizations will increasingly prioritize responsible AI adoption, integrating ethical considerations and bias detection and mitigation into their AI development pipelines.
As data science and machine learning continue their extraordinary journey, they hold the promise of transforming industries, shaping decisions, and revolutionizing our understanding of the world. This partnership between data science's holistic approach and machine learning's predictive power is at the heart of this transformation. However, it is not without its challenges, particularly in terms of ethics and fairness. Ensuring that these technologies contribute positively to society while minimizing potential drawbacks is a collective responsibility. The future of data science and machine learning is bright, filled with endless possibilities and opportunities for innovation. By embracing these technologies responsibly, we can pave the way for a data-driven future that benefits us all, pushing the boundaries of what's possible in our increasingly interconnected world.