Big Data and Data Science: Challenges and Opportunities
Explore the dynamic landscape of Big Data and Data Science, uncovering the challenges and opportunities that shape the future of technology and business.
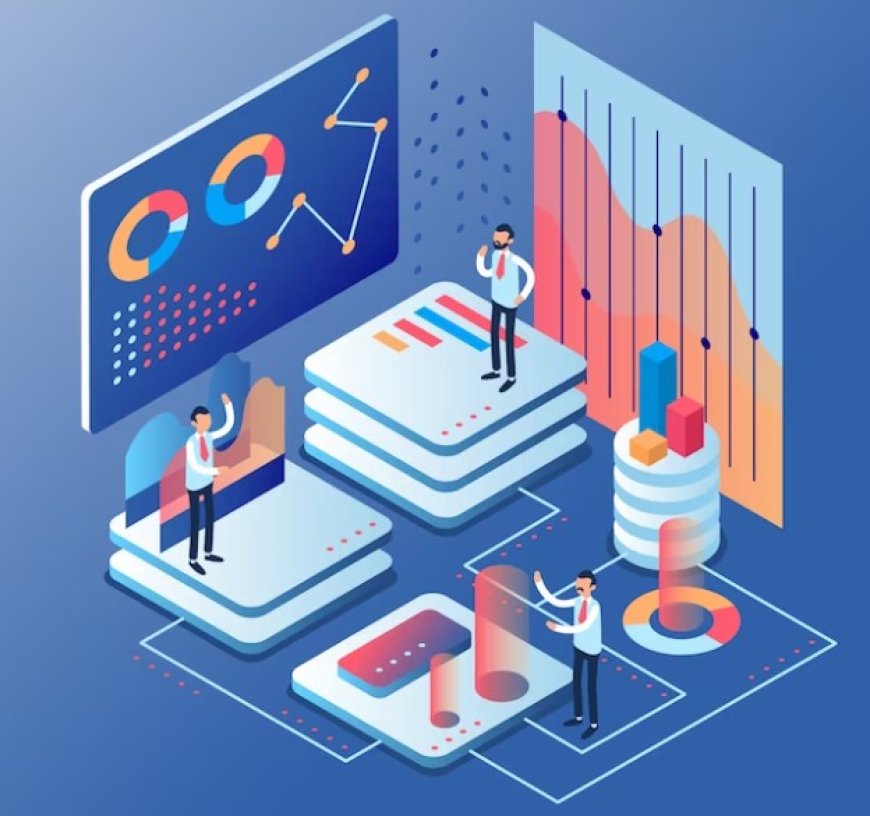
In today's data-driven world, the fields of Big Data and Data Science have emerged as transformative forces across industries. The sheer volume, velocity, variety, and veracity of data generated daily present both challenges and opportunities. This article delves into the multifaceted landscape of Big Data and Data Science, examining the hurdles they pose, the advantages they offer, and the ethical considerations within. As we explore these themes, it becomes clear that in this era of information overload, harnessing the power of data is not only a technological endeavor but also a strategic imperative.
Big Data and Data Science
Big Data refers to exceptionally large and complex datasets that exceed the capacity of traditional data processing tools to capture, store, manage, and analyze efficiently. It encompasses vast volumes of structured, semi-structured, and unstructured data from various sources, such as social media, sensors, and transactions.
Data Science, on the other hand, is a multidisciplinary field that employs scientific methods, algorithms, processes, and systems to extract valuable insights and knowledge from Big Data. It combines elements of statistics, machine learning, computer science, and domain expertise to solve complex problems, make data-driven decisions, and uncover patterns and trends within these massive datasets. Essentially, Data Science bridges the gap between data and actionable insights in the context of Big Data's challenges and opportunities.
Challenges in Big Data and Data Science
-
Volume: Dealing with the sheer volume of data generated daily presents a significant challenge. Storing and processing massive datasets require robust infrastructure and specialized tools. Organizations must invest in scalable storage solutions and distributed computing frameworks to manage this data influx effectively.
-
Velocity: In today's fast-paced digital landscape, data is generated and updated in real-time. This velocity requires data scientists to employ stream processing techniques and technologies to extract insights promptly. Failure to keep up with data velocity can result in missed opportunities or delayed decision-making.
-
Variety: Data comes in various formats, including structured, semi-structured, and unstructured data. Combining and making sense of these heterogeneous data sources can be complex. Data scientists need to adapt their techniques to work with different data types, ensuring a comprehensive analysis that captures the full spectrum of available information.
-
Veracity: Data quality is paramount in data science. Inaccurate or incomplete data can lead to flawed conclusions and decisions. Ensuring data veracity involves rigorous data cleaning, validation, and quality control processes. Establishing trust in data sources and reliability is crucial for the integrity of data-driven insights.
-
Value: The ultimate goal of data science is to extract valuable insights from data. However, identifying what is truly valuable within the data can be challenging. Data scientists must apply advanced analytical techniques, such as machine learning and data mining, to sift through the noise and discover actionable insights that drive informed decisions and create value for organizations.
Opportunities in Big Data and Data Science
Data-Driven Decision Making: Big Data and Data Science empower organizations to make informed decisions based on data analysis. This leads to improved business strategies, better resource allocation, and enhanced customer experiences. In healthcare, it enables personalized medicine and proactive disease management.
Predictive Analytics: These technologies enable organizations to forecast future trends, behaviors, and events. Businesses can use predictive analytics for demand forecasting, risk assessment, and fraud detection. It also plays a crucial role in optimizing supply chains and financial models.
Machine Learning and AI Integration: The integration of Machine Learning and Artificial Intelligence into Big Data analytics allows for automation and efficiency gains. Natural Language Processing (NLP) applications are revolutionizing customer support, content recommendation, and chatbots.
Big Data in Research and Science: Big Data analytics is transforming various scientific fields. Genomics research relies on massive datasets for personalized medicine and genetic mapping. Climate scientists use Big Data to model climate patterns and predict environmental changes. Social sciences and market researchers leverage it for in-depth analysis and understanding of human behavior.
Career Opportunities and Demand: The demand for skilled data scientists and analysts continues to grow. As organizations recognize the value of data, a wide range of career opportunities emerges, including data scientists, data engineers, machine learning engineers, and business analysts. This field's dynamic nature offers professionals exciting career paths and constant learning opportunities.
Ethical and Privacy Considerations
Data Privacy Concerns
In the era of big data and data science, the issue of data privacy looms large. Individuals and organizations are generating vast amounts of data, raising concerns about how this information is collected, stored, and used. This includes questions about the ownership of personal data, the extent to which it can be shared, and the potential for data breaches. Stringent data protection regulations, such as the General Data Protection Regulation (GDPR), have emerged to address these concerns, emphasizing the need for transparency, consent, and secure data handling practices.
Bias and Fairness in Algorithms
Algorithms play a pivotal role in data science applications, making automated decisions that impact various aspects of our lives. However, these algorithms are not immune to bias. They can inherit biases present in the data they are trained on, leading to unfair outcomes, discrimination, and skewed predictions. Addressing bias in algorithms is a critical ethical consideration. Researchers and practitioners are actively working on developing fairness-aware machine learning techniques to mitigate bias and ensure that data-driven decisions are equitable and just. Ethical discussions and guidelines are essential to navigate this complex terrain responsibly.
Future Trends in Big Data and Data Science
As we move forward in the realm of big data and data science, several exciting trends are shaping the future of this field.
One of these trends is the rise of edge computing and edge analytics. With the proliferation of IoT devices, data processing is increasingly happening at the source rather than in centralized data centers. This shift brings challenges and opportunities for real-time analytics and reduced latency.
Quantum computing is another frontier that promises to revolutionize data science. Quantum computers have the potential to solve complex problems exponentially faster than classical computers, opening up new possibilities for data analysis, optimization, and cryptography.
Advancements in data visualization are also on the horizon. As data sets grow in size and complexity, data scientists will need more sophisticated and interactive visualization tools to extract insights effectively and communicate findings to non-technical stakeholders.
Interdisciplinary collaborations between data scientists and experts from various domains, such as healthcare, finance, and environmental science, are expected to become more prevalent. These collaborations will lead to innovative applications of data science in solving domain-specific challenges.
Lastly, the field of big data and data science is known for its dynamism. As technologies and methodologies continue to evolve, it's crucial for professionals to stay updated and adapt to these changes, ensuring the field remains at the forefront of innovation.
The world of big data and data science presents both formidable challenges and unprecedented opportunities. Managing the sheer volume, velocity, variety, veracity, and value of data is essential for success. Embracing -driven decision-making, predictive analytics, machine learning, and artificial intelligence can revolutionize industries. However, ethical concerns and data privacy issues demand careful consideration. Looking ahead, emerging trends like edge computing and quantum computing are set to reshape the field. As we navigate this dynamic landscape, one thing remains certain: big data and data science will continue to play a pivotal role in shaping our future.