Data Science in Marketing: Personalization and Customer Insights
Discover the power of Data Science in marketing for personalized campaigns and deep customer insights. Harness data-driven strategies for effective marketing.
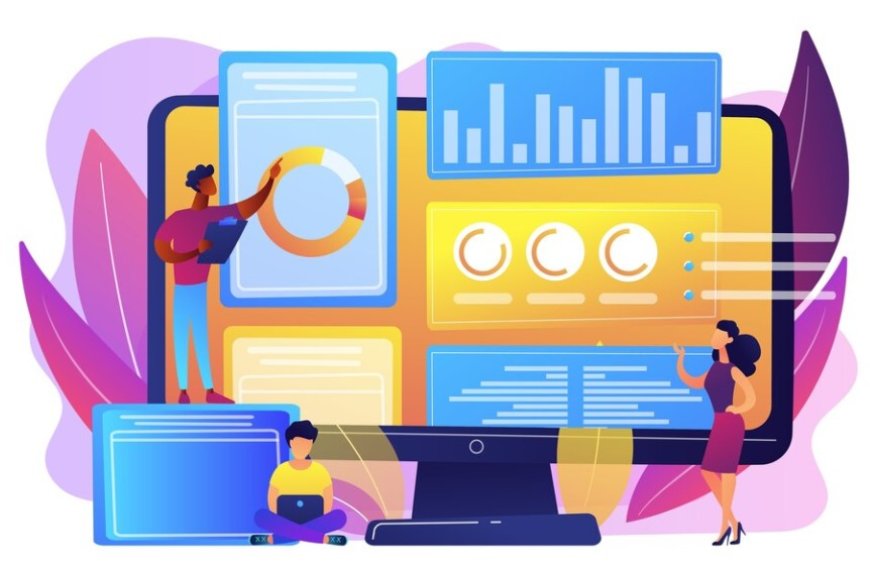
Data science in marketing is the application of data analysis and machine learning techniques to gather insights, enhance personalization, and improve decision-making in marketing strategies. It involves the use of data from various sources to understand customer behavior, optimize campaigns, and deliver tailored experiences. This overview highlights the transformative role of data science in revolutionizing marketing practices and driving businesses towards more effective and customer-centric approaches.
Importance of personalization and customer insights
Personalization and customer insights are paramount in today's highly competitive business landscape. Personalization allows companies to tailor their products, services, and marketing efforts to individual customer preferences. This not only enhances the customer experience but also drives higher engagement and conversion rates.
Customer insights, on the other hand, provide a deep understanding of customer behaviors, needs, and pain points. By analyzing customer data, businesses can make informed decisions, improve product development, optimize marketing strategies, and identify growth opportunities. Ultimately, both personalization and customer insights empower organizations to build stronger customer relationships and achieve sustainable growth in a data-driven world.
Data Collection
Effective data collection is the foundation of data science in marketing. It involves three crucial aspects:
-
Gathering data from various sources: Data can come from diverse channels such as websites, social media, CRM systems, surveys, and more. The ability to aggregate data from these sources is vital for comprehensive analysis.
-
Types of data (structured, unstructured, etc.): Data comes in various forms, including structured data (e.g., databases) and unstructured data (e.g., text, images). Understanding the types of data being collected helps in selecting appropriate analysis techniques.
-
Data quality and preprocessing: Ensuring data quality is essential. This includes handling missing values, outliers, and inconsistencies. Data preprocessing involves cleaning, transforming, and preparing the data for analysis, making it a critical step in the data science pipeline.
Data Analysis
Data analysis is a crucial step in leveraging data science for marketing. It involves several key components:
Exploratory Data Analysis (EDA): EDA is the initial phase of data analysis, aimed at gaining insights into the dataset's characteristics. Data scientists use various techniques like summary statistics, histograms, and scatter plots to understand the data's distribution, identify outliers, and uncover potential patterns.
Descriptive and Inferential Statistics: Descriptive statistics help in summarizing and describing the main features of the data, such as mean, median, and standard deviation. Inferential statistics, on the other hand, allow data scientists to make predictions and draw conclusions about the larger population based on a sample.
Data Visualization Techniques: Data visualization is a powerful tool for communicating insights effectively. Techniques like bar charts, heatmaps, and line graphs help in presenting data patterns and trends in a visually intuitive manner. Effective data visualization can aid decision-makers in understanding complex marketing data.
These components collectively enable data scientists to extract meaningful information from raw data, making it a foundation for informed marketing strategies and personalized customer experiences.
Machine Learning Models
In the realm of Data Science in Marketing, Machine Learning Models play a pivotal role in harnessing customer data for personalized strategies. This involves three key applications:
Recommender Systems: These algorithms analyze customer preferences and behavior to suggest products, content, or services tailored to individual tastes. Widely used in e-commerce platforms and streaming services, they enhance user experience and drive engagement.
Customer Segmentation: By clustering customers based on shared characteristics, this model allows for targeted marketing campaigns. It enables businesses to tailor messages, offers, and experiences to specific segments, optimizing marketing efforts and increasing conversion rates.
Predictive Modeling for Customer Behavior: This involves using historical data to forecast future customer actions, such as purchases, clicks, or churn. By understanding potential behavior patterns, businesses can proactively adjust strategies to retain customers, maximize revenue, and drive growth. These machine learning models collectively empower marketers to make data-driven decisions for more effective and personalized customer interactions.
Personalization Strategies
Personalization is a cornerstone of data science in marketing. It involves tailoring marketing efforts to individual customer preferences and behaviors.
-
Content recommendation is a key strategy, leveraging algorithms to suggest products, content, or services based on a customer's past interactions.
-
Dynamic pricing adjusts product prices in real-time based on factors like demand, customer history, and competitor pricing.
-
Email marketing personalization entails crafting customized email campaigns that resonate with recipients, considering their past purchases and engagement. These strategies enhance customer engagement and satisfaction.
Customer Insights
Customer insights play a pivotal role in data science-driven marketing. This phase involves a deep dive into understanding customer behaviors, preferences, and needs. Key components of this stage include customer journey mapping, sentiment analysis, and churn prediction and prevention.
Customer Journey Mapping: Mapping out the customer's interactions with your brand is essential for gaining insights into their experiences. It helps identify touchpoints, pain points, and opportunities for personalization. This process aids in tailoring marketing strategies to enhance the overall customer journey.
Sentiment Analysis: Analyzing customer feedback, reviews, and social media comments using natural language processing techniques provides valuable insights into customer sentiment. It allows marketers to gauge customer satisfaction, identify potential issues, and adapt messaging accordingly.
Churn Prediction and Prevention: Predictive modeling can help anticipate customer churn or attrition. By identifying at-risk customers early, businesses can implement strategies to retain them, such as personalized offers or targeted communication.
Incorporating these insights into marketing strategies can lead to more effective campaigns, improved customer satisfaction, and better business outcomes.
Tools and Technologies
In the realm of data science in marketing, a wide array of tools and technologies play a pivotal role in gathering, processing, and deriving insights from data. These include popular programming languages such as Python and R, which are essential for data manipulation and statistical analysis. Machine learning libraries like Scikit-Learn and TensorFlow empower marketers to build predictive models for customer behavior and recommendation systems. Customer data platforms (CDPs) are instrumental in centralizing customer information. Additionally, data visualization tools like Tableau and Power BI aid in presenting data-driven insights effectively to inform marketing strategies. Staying current with these tools is crucial for success in the field.
Ethical Considerations
In the realm of data science in marketing, it's crucial to address ethical considerations. As businesses leverage customer data for personalization and insights, they must prioritize privacy and data security. Respecting customer consent, adhering to relevant regulations like GDPR or CCPA, and ensuring transparent data usage are paramount. Additionally, guarding against biases in algorithms that might perpetuate discrimination is essential. Striking the right balance between data-driven marketing and ethical responsibility is a fundamental aspect of successful and responsible data science in marketing.
Future Trends
In the rapidly evolving field of data science in marketing, two key areas are expected to shape the future:
AI Advancements in Marketing: Artificial intelligence (AI) will continue to play a pivotal role in marketing strategies. AI-driven chatbots, personalized content generation, and enhanced customer profiling are just the beginning. As AI algorithms become more sophisticated, marketers will harness their power to deliver highly tailored, real-time experiences to customers.
Predictive Analytics Evolution: Predictive analytics will undergo significant advancements, enabling marketers to anticipate customer behavior and market trends with greater accuracy. Machine learning models and big data analytics will be further integrated, empowering businesses to make data-driven decisions, optimize campaigns, and stay ahead of their competitors.
These future trends underscore the importance of staying updated with emerging technologies and methodologies to remain competitive in the ever-changing landscape of data science in marketing.
Data science plays a pivotal role in modern marketing by enabling personalization and providing invaluable customer insights. Through data collection, analysis, and the application of machine learning models, businesses can enhance their marketing strategies and create more meaningful customer experiences. It's essential for professionals in this field to stay updated with the latest tools and technologies, as the landscape continues to evolve rapidly. Continuous learning and adaptation are key to harnessing the full potential of data science in marketing and staying ahead in the competitive market.