Data Science vs. Data Analytics: Understanding the Key Differences
Explore their definitions, scopes, tools, and real-world applications to make informed decisions for your career or business strategies.
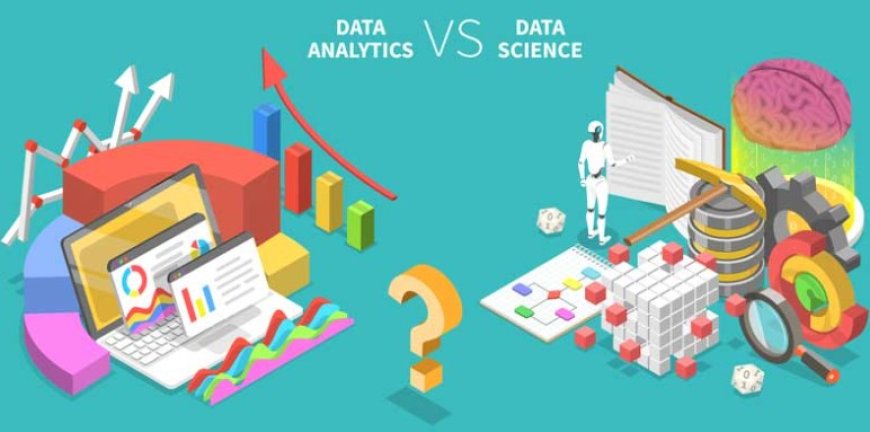
Data Science and Data Analytics are often used interchangeably, but they represent distinct domains in the field of data-driven decision-making. Data Science encompasses a broader spectrum, involving the entire process of gathering, cleaning, analyzing, and interpreting data to extract valuable insights. On the other hand, Data Analytics primarily focuses on examining datasets to draw conclusions and support decision-making. It's crucial to differentiate between these disciplines, as they cater to different organizational needs and require varying skill sets. Understanding these distinctions is vital for businesses to deploy the right strategies and utilize data effectively in today's data-driven world.
Data Science
Data Science encompasses a multidisciplinary field focused on extracting insights and knowledge from complex and unstructured data. Its scope extends from data collection and cleaning, through exploratory data analysis (EDA), to the application of advanced techniques such as machine learning and predictive modeling. These core components enable data scientists to uncover patterns, make predictions, and drive informed decision-making. In the real world, data science finds application in diverse industries, including finance, healthcare, marketing, and more. The skills required for a data scientist include proficiency in programming languages like Python or R, a deep understanding of statistical concepts, and expertise in data manipulation and visualization tools. Additionally, a data scientist must possess a strong problem-solving aptitude and the ability to communicate findings effectively to stakeholders.
Data Analytics
Data Analytics focuses on deriving meaningful insights from data through various techniques and tools. It involves the examination of historical data to identify trends, patterns, and make informed business decisions. The scope of data analytics encompasses descriptive analytics, which summarizes past data to understand what has happened. Diagnostic analytics digs deeper to determine why certain events occurred. The core components of data analytics include data querying and exploration, statistical analysis, data visualization, and the creation of dashboards and reports.
In real-world applications, data analytics plays a crucial role in fields such as marketing, finance, operations, and customer service. For instance, businesses use analytics to optimize marketing campaigns, assess financial performance, and enhance operational efficiency. Additionally, healthcare institutions employ data analytics to improve patient outcomes and streamline operations.
To excel in data analytics, professionals should possess a combination of technical and analytical skills. This includes proficiency in tools like Excel, SQL, and specialized analytics platforms. Statistical knowledge is crucial for interpreting data, and familiarity with data visualization tools aids in conveying insights effectively. Strong problem-solving abilities and the capacity to communicate findings are also vital skills for success in the field.
Key Differences
Objective: Data science primarily focuses on extracting insights, building predictive models, and solving complex problems by analyzing large and unstructured datasets. It aims to uncover hidden patterns and generate actionable insights. In contrast, data analytics primarily revolves around examining historical data to identify trends, answer specific questions, and make data-driven decisions. Its primary objective is to provide a descriptive summary of past events.
Methodology: Data science often employs advanced statistical and machine learning techniques to handle vast and diverse datasets. It involves the entire data lifecycle, from data collection and preprocessing to model development and deployment. Data scientists emphasize experimentation and hypothesis testing. Data analytics, on the other hand, relies on traditional statistical methods, querying databases, and data visualization. It typically follows a structured and predefined analysis process.
Tools and Technologies: Data science relies on a broader range of tools and technologies, including programming languages like Python and R, deep learning frameworks like TensorFlow, and big data platforms like Hadoop. Data scientists also use cloud computing extensively for scalability. Data analytics typically uses tools like Excel, SQL, and business intelligence software such as Tableau or Power BI. While both fields can overlap in tool usage, data science typically demands more diverse and specialized tools.
Output and Deliverables: Data science outputs often include predictive models, machine learning algorithms, data-driven recommendations, and automated decision-making systems. These outputs are designed to guide future actions and strategies. Data analytics outputs consist of descriptive reports, dashboards, and visualizations, which provide historical insights and support decision-making based on past data. The key difference lies in the actionable nature of data science outputs compared to the descriptive nature of data analytics deliverables.
When to Use Each
Data Science:
Data science is best suited for scenarios where organizations need to extract complex insights and predictions from large and diverse datasets. It shines in situations where traditional analysis methods fall short. For instance, data science is indispensable in industries like healthcare for predicting disease outbreaks, in finance for fraud detection, and in e-commerce for recommendation systems. Any situation that requires advanced machine learning or deep learning models to make informed decisions benefits from data science expertise.
Data Analytics:
Data analytics is well-suited for scenarios where organizations primarily require descriptive and diagnostic insights from data. It excels at providing historical and current data-driven observations and explanations. Industries like marketing use data analytics for customer segmentation and campaign performance analysis. Additionally, data analytics is vital in supply chain management for optimizing operations. When the primary goal is to answer "what happened" or "why it happened" questions, data analytics is the go-to approach.
Overlapping Areas
Data Science and Data Analytics Intersections
Data science and data analytics share common ground in several areas. One notable intersection is in the realm of data preprocessing. Both disciplines involve data cleaning, transformation, and preparation to ensure data quality and suitability for analysis. Additionally, descriptive analytics, a component of data analytics, often forms the foundation for more advanced data science work. Data scientists may rely on descriptive analytics insights to inform their machine learning models and predictions. These shared tasks highlight the symbiotic relationship between data science and data analytics.
Collaborative Roles in Organizations
In many organizations, data scientists and data analysts collaborate closely. Data analysts excel at extracting meaningful insights from data and creating reports and dashboards that provide actionable information to stakeholders. Data scientists, on the other hand, leverage advanced statistical and machine learning techniques to build predictive models and uncover deeper patterns. This collaboration ensures that data-driven decisions are not only based on historical data (analytics) but also on future predictions and optimizations (data science). Effective communication between these roles is essential for driving data-driven strategies and innovations within an organization.
Career Paths and Opportunities
In the field of data science, professionals often take on roles such as data scientist, machine learning engineer, AI specialist, or data engineer. Data scientists focus on developing predictive models, conducting advanced statistical analyses, and extracting actionable insights from data. Machine learning engineers work on designing and implementing machine learning algorithms, while AI specialists delve into artificial intelligence applications. Data engineers are responsible for data collection, storage, and management. These roles typically require strong programming skills, expertise in data manipulation, and a deep understanding of machine learning.
Data analytics roles encompass positions like data analyst, business analyst, marketing analyst, or business intelligence analyst. Data analysts primarily work on gathering and interpreting data to generate reports and visualizations. Business analysts focus on using data to inform business decisions and strategies. Marketing analysts specialize in leveraging data to optimize marketing campaigns. These roles often require proficiency in data visualization tools, data querying languages, and business acumen.
Both data science and data analytics fields are experiencing rapid growth and high demand in today's job market. As organizations increasingly rely on data-driven insights, professionals in these domains are sought after across various industries such as healthcare, finance, e-commerce, and technology. The job market for data scientists and data analysts is competitive, offering excellent career prospects and the potential for continued growth as businesses continue to recognize the value of data-driven decision-making. Keeping up-to-date with industry trends and continuously improving skills is essential to thrive in these dynamic career paths.
It's crucial to understand the distinctions between data science and data analytics. Data science encompasses a broader range of tasks, including predictive modeling and deep learning, while data analytics primarily focuses on descriptive and diagnostic analytics. Choosing the right approach depends on the specific objectives of a project. Looking ahead, both fields are poised for continued growth, with advancements in AI, automation, and the integration of big data shaping the future of data science and analytics. Staying updated with emerging trends will be paramount for professionals in both domains.