Understanding the Basics: What Is Artificial Intelligence?
Unlock the fundamentals of Artificial Intelligence (AI) with "Understanding the Basics: What Is Artificial Intelligence?" Discover the essence of AI and its real-world applications in this comprehensive guide.
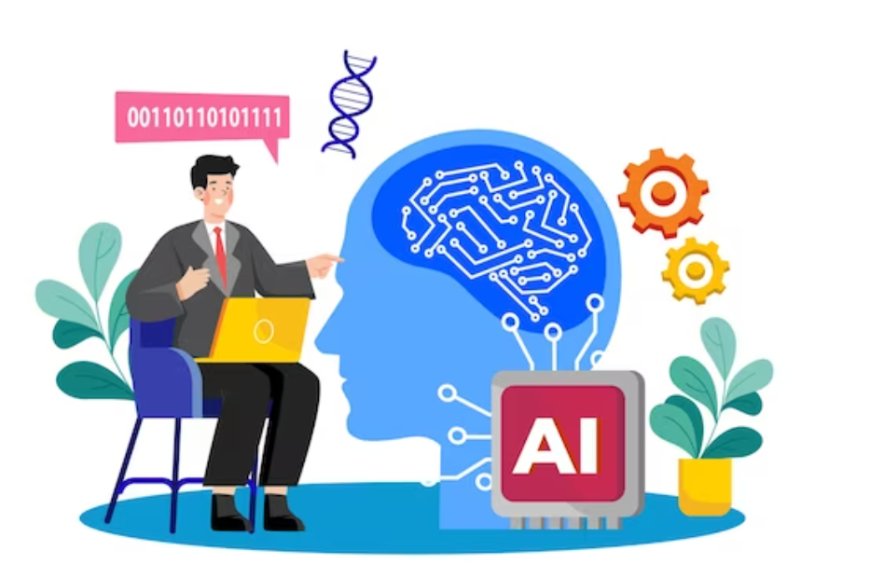
Artificial Intelligence (AI) is a quickly evolving field that involves the development of computer systems capable of performing tasks that typically require human intelligence, such as problem-solving, learning, and decision-making. In today's world, AI has become increasingly essential across various industries and sectors, ranging from healthcare and finance to transportation and entertainment. Its importance lies in its ability to automate and enhance processes, analyze vast amounts of data, and create innovative solutions, ultimately shaping the way we live and work in the 21st century. Understanding the fundamentals of AI is crucial for anyone seeking to navigate and thrive in this technologically driven era.
Types of Artificial Intelligence
Narrow AI (Weak AI): Narrow AI, often referred to as Weak AI, represents the most prevalent form of AI in use today. It is designed to perform specific tasks or solve particular problems. These AI systems excel at their designated tasks but lack the ability to generalize their knowledge to other domains. Examples of Narrow AI include virtual assistants like Siri, recommendation algorithms on streaming platforms, and autonomous vehicles.
General AI (Strong AI): General AI, also known as Strong AI, is an aspirational concept where machines possess human-like intelligence and cognitive abilities. Unlike Narrow AI, General AI would have the capacity to understand and learn from any task, exhibit reasoning, and adapt to new situations. Achieving General AI remains a significant challenge, and it has yet to be realized.
Superintelligent AI: Superintelligent AI is a theoretical concept that goes beyond General AI. It implies AI systems with intellectual capabilities surpassing those of humans in every aspect. This level of AI could potentially solve complex problems, enhance scientific research, and even make decisions far more efficiently than humans. Superintelligent AI raises profound ethical and existential questions and is a topic of extensive debate among experts.
Practical Applications
AI finds applications across various domains, revolutionizing industries and enhancing human capabilities. Some practical applications include:
-
Healthcare: AI aids in medical diagnosis, drug discovery, and personalized treatment plans.
-
Finance: AI algorithms are used for fraud detection, algorithmic trading, and credit risk assessment.
-
Education: AI-powered tools facilitate personalized learning and adaptive tutoring.
-
Manufacturing: AI-driven robotics enhance automation and quality control in manufacturing processes.
-
Transportation: Self-driving cars and AI-based traffic management systems aim to improve road safety and efficiency.
-
Natural Language Processing (NLP): NLP applications like chatbots, language translation, and sentiment analysis improve communication and understanding between humans and machines.
-
Computer Vision: Computer vision systems enable image and video analysis, aiding in tasks such as object recognition and autonomous navigation.
Understanding these different types of AI and their practical applications is essential in grasping the diverse impact AI has on our daily lives and the potential it holds for the future.
Machine Learning and Deep Learning
Definitions and Distinctions
Machine Learning (ML) and Deep Learning (DL) are two critical components of artificial intelligence (AI), each with its own characteristics and applications. Machine Learning is a broader concept that refers to the ability of a system to improve its performance on a task through learning from data. It encompasses various techniques like regression, decision trees, and support vector machines. On the other hand, Deep Learning is a subset of ML that focuses on neural networks with multiple layers, known as deep neural networks. The key distinction lies in the complexity and depth of the models. While ML methods can handle a wide range of tasks, DL excels in tasks requiring complex feature extraction and pattern recognition.
Role of Data in AI
Data is the lifeblood of AI, particularly in the context of Machine Learning and Deep Learning. In both cases, algorithms learn from vast amounts of data to make predictions, classify objects, or generate insights. The availability and quality of data significantly influence the success of AI models. High-quality, labeled data is essential for training accurate and reliable models. Additionally, data preprocessing and cleaning play a crucial role in ensuring the effectiveness of AI systems.
Neural Networks and Their Significance
Neural networks are the fundamental building blocks of Deep Learning. They are inspired by the structure and function of the human brain, with interconnected nodes (neurons) organized into layers. Each connection between neurons is associated with a weight, which is adjusted during training to learn patterns in the data. The significance of neural networks lies in their ability to automatically extract features from raw data, eliminating the need for manual feature engineering. This feature extraction capability is particularly valuable in tasks such as image and speech recognition, where traditional methods often fall short. Deep neural networks, with many layers, have shown remarkable performance in various AI applications, propelling the field of AI to new heights.
AI in Action
Real-world applications
AI has found its way into various real-world applications, such as autonomous vehicles. Self-driving cars use AI algorithms and sensors to navigate roads, make decisions, and ensure passenger safety. This technology is rapidly evolving and has the potential to revolutionize transportation. Chatbots and virtual assistants are becoming increasingly prevalent in customer service and support. These AI-powered systems can answer questions, provide information, and even simulate human-like conversations, improving user experiences.
AI in healthcare, finance, and other industries
In healthcare, AI is used for medical image analysis, disease diagnosis, and drug discovery. Machine learning models can analyze medical images like X-rays and MRIs, aiding doctors in identifying diseases and conditions.The finance industry leverages AI for fraud detection, algorithmic trading, and risk assessment. AI algorithms analyze vast amounts of financial data to make investment decisions and predict market trends.
Ethical considerations
As AI becomes more integrated into daily life and industries, ethical considerations have come to the forefront. One significant concern is bias in AI algorithms, which can perpetuate existing inequalities and discrimination. Privacy issues arise as AI systems collect and analyze vast amounts of personal data. Ensuring the responsible use of this data is crucial to protect individuals' privacy. There is an ongoing debate about the impact of AI on employment, with concerns about job displacement due to automation.
These real-world applications and ethical considerations highlight the transformative power of AI and the need for responsible development and deployment.
How AI Works?
Data Collection and Preprocessing
Data collection and preprocessing are fundamental steps in the AI process. Data serves as the foundation upon which AI models are built. In the data collection phase, AI systems gather vast amounts of information from diverse sources such as sensors, databases, and the internet. This raw data may be unstructured, noisy, and incomplete, making preprocessing crucial. During preprocessing, data is cleaned, organized, and transformed to ensure it is suitable for analysis. This involves tasks like removing outliers, handling missing values, and converting data into a standardized format. The quality of the input data greatly influences the effectiveness of the AI system, making data collection and preprocessing essential initial steps.
Training and Algorithms
The training and algorithm phase is where AI models learn from the preprocessed data. Machine learning algorithms play a central role in this process. Supervised learning algorithms learn from labeled data, which means they are provided with input-output pairs and learn to map inputs to desired outputs. Unsupervised learning algorithms, on the other hand, explore data without labeled guidance, seeking patterns, clusters, or anomalies. Deep learning models, often based on neural networks, are employed for complex tasks such as image recognition, natural language processing, and autonomous decision-making. These models iteratively adjust their internal parameters through training to minimize errors and improve performance on the training data.
Testing and Validation
After the AI model has been trained, it must undergo rigorous testing and validation to ensure its reliability and generalization to new, unseen data. Testing is carried out using a separate dataset that was not part of the training process. This evaluation dataset helps assess how well the AI system performs its designated task when faced with real-world scenarios. Various validation metrics, such as accuracy, precision, recall, and F1 score, are used to measure the model's performance. Testing and validation phases serve the critical purpose of detecting overfitting, where the model memorizes the training data but struggles to generalize to new data. The insights gained from this phase guide further refinements and improvements in the AI system.
Challenges and Limitations
Bias and Fairness
One of the foremost challenges in artificial intelligence is the issue of bias and fairness. AI systems, especially those trained on large datasets, can inherit and perpetuate biases present in the data. This can lead to discriminatory outcomes in various applications, such as hiring, lending, and criminal justice. Ensuring that AI algorithms are fair and free from bias is an ongoing challenge that requires constant vigilance and refinement.
Data Privacy and Security
Data privacy and security are critical concerns in the world of AI. AI systems often require access to vast amounts of data, and the misuse or mishandling of this data can have serious consequences. Ensuring that personal and sensitive information is protected while still enabling AI to function effectively is a complex balancing act. The risk of data breaches and unauthorized access is a constant threat that AI developers and organizations must address.
Human-AI Collaboration
Achieving effective collaboration between humans and AI systems is another significant challenge. While AI can automate many tasks and processes, there are limitations to its understanding of human context, emotions, and nuanced communication. Striking the right balance between automation and human involvement, particularly in fields like healthcare and customer service, is an ongoing challenge. Additionally, addressing the psychological and social aspects of human-AI interaction, including concerns about job displacement, is crucial for successful integration.
These challenges and limitations highlight the need for continuous research, development, and ethical considerations in the field of artificial intelligence. Overcoming these obstacles will be essential for harnessing the full potential of AI while mitigating potential risks.
The Future of AI
Emerging trends and technologies
As AI continues to evolve, several emerging trends and technologies are shaping its future. These include advancements in reinforcement learning, generative adversarial networks (GANs), and quantum computing. Additionally, AI is making significant strides in fields such as healthcare, where it is being used for drug discovery and personalized medicine. Robotics and autonomous systems are also becoming more sophisticated, leading to applications in industries like logistics and manufacturing. Understanding these emerging trends is crucial for staying up-to-date with AI's rapid development.
AI's impact on jobs and society
The widespread adoption of AI technologies is bound to have a profound impact on the job market and society as a whole. Automation may lead to the displacement of certain jobs while creating new opportunities in AI-related fields. It's essential to explore how AI can be harnessed to augment human capabilities and promote a balance between automation and human labor. Additionally, addressing the potential societal implications of AI, such as income inequality and job displacement, is crucial to ensuring a fair and equitable future.
Ethical AI development and regulation
As AI becomes increasingly integrated into our lives, ethical considerations are paramount. Developers and policymakers must work together to establish guidelines and regulations that ensure the responsible development and deployment of AI systems. This includes addressing issues related to bias, transparency, and accountability in AI algorithms. Ethical AI practices should prioritize fairness, transparency, and the avoidance of harm. Understanding the ethical dimensions of AI is essential for creating a future where AI benefits society while mitigating potential risks.
This exploration of AI has highlighted its transformative capabilities and potential impact on society. We've emphasized the importance of understanding AI in the modern world, as it plays a pivotal role in various domains, from healthcare to finance. To fully harness its benefits and address its challenges, further exploration and learning about AI are essential. Stay curious, keep learning, and embrace the opportunities AI offers while responsibly navigating its complexities.