Applications of Machine Learning in Healthcare
Machine learning is changing healthcare by helping doctors find diseases early, create personalized treatments, and improve patient care. Don't miss the future of health!
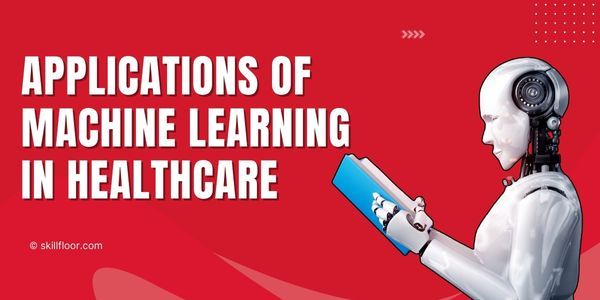
Imagine going to your doctor, and in only a few minutes, they can identify the specific issue, provide the best course of action, and even forecast your response. Even if it seems like science fiction, machine learning (ML) is rapidly making it a reality.
Healthcare is changing as a result of machine learning, which enables physicians to make quicker and more precise judgments. Doctors may identify illnesses sooner and develop individualized treatment regimens because of the applications of machine learning in healthcare, medical imaging, and predictive analytics. This improves patient outcomes and lowers expenses.
Machine learning needs to be precise, objective, and safe in order to be trusted in the medical field. Medical personnel must supervise the technology and make sure the data is diverse and thorough. In healthcare applications, maintaining patient privacy through robust security measures is still of utmost importance.
What is Machine Learning?
Computers may now learn from experience by using a technique called machine learning. These systems can forecast or make judgments without explicit instructions by examining patterns in data. It's similar to educating a computer to improve over time.
Machine learning is used in healthcare to process vast volumes of health data, which benefits physicians and medical personnel. This technology improves the efficiency, accuracy, and patient-centeredness of healthcare by identifying patterns, diagnosing illnesses, and forecasting treatment results.
The Growing Importance of Machine Learning Across Different Industries
1. Healthcare
Machine learning analyzes patient data and medical pictures to assist doctors detect illnesses more quickly and precisely, which results in early treatments and better patient outcomes.
2. Finance
Machine learning in finance makes financial operations safer and more effective by identifying fraudulent activity, forecasting market trends, and automating customer service and other functions.
3. Retail
Retailers employ machine learning to enhance inventory management, promote items, and customize shopping experiences, which improves consumer happiness and boosts revenue.
5. Manufacturing
By automating quality control, streamlining supply chains, and anticipating equipment failures, machine learning increases production efficiency and helps firms save downtime while enhancing product quality.
6. Transportation
Self-driving cars, intelligent traffic control, and predictive maintenance are just a few examples of how machine learning is transforming transportation and making travel safer, quicker, and more effective for everybody.
7. Education
Machine learning in education helps teachers design individualized lessons and gives them insights into student performance to enhance results by customizing learning experiences to each student's requirements.
Additionally, becoming a Certified Machine Learning Professional can further enhance one's ability to apply these technologies effectively across various industries.
How is Machine Learning Used in Healthcare
Healthcare is changing as a result of machine learning, which enables physicians to make judgments more quickly and accurately. Large datasets may be analyzed to find trends and provide insights that enhance patient outcomes, treatment strategies, and diagnosis. Numerous and expanding daily are the applications of machine learning in healthcare.
Machine learning models in medical imaging can identify early indicators of disorders like cancer or cardiac problems that may be too subtle for the human eye to notice. These tools provide physicians with an extra level of knowledge by improving the diagnosis process, guaranteeing quicker and more accurate treatment.
Additionally, by assisting in the identification of patients who are at risk for specific illnesses, machine learning is transforming predictive analytics. Personalized treatment plans and early interventions are made possible by the analysis of patient histories and genetic data, which also lowers costs and improves overall healthcare efficiency while providing higher-quality care.
Applications of Machine Learning in Healthcare
1. Early Disease Detection
Through the analysis of medical imaging and patient data, machine learning assists physicians in early detection of conditions like cancer and heart disease. More precise and prompt diagnoses are made possible by applications of machine learning in healthcare.
2. Personalized Treatment Plans
Medical applications of machine learning can develop customized treatment regimens by examining a patient's genetics, lifestyle, and medical history. This improves patient outcomes and lowers adverse effects.
3. Medical Imaging Analysis
By identifying symptoms of illnesses that the human eye might miss, machine learning improves the examination of medical photographs. The speed and accuracy of diagnosis are increased by these applications of machine learning in healthcare.
4. Predictive Analytics for Health Risks
Health risks are predicted by machine learning algorithms, which allows physicians to take early action and stop diseases from getting worse. The proactive treatment made possible by these applications of machine learning in healthcare enhances patients' general health.
5. Drug Discovery and Development
By evaluating vast collections of chemical compounds and forecasting their efficacy, machine learning speeds up the medication development process. This makes it a useful tool in healthcare as it expedites the process of discovering novel medicines.
Data preparation is essential in the healthcare industry to clean and get medical data ready for analysis. Effective machine learning models are ensured by proper preprocessing, which improves patient care and produces more accurate predictions.
7. Hospital Operations Optimization
By forecasting patient admission rates, controlling staff schedules, and cutting down on wait times, machine learning improves hospital operations. Healthcare systems become more responsive and efficient as a result of these machine learning technologies.
8. Virtual Health Assistants
Virtual assistants help patients with appointment scheduling, health tracking, and receiving medical advice are powered by machine learning. Better decision-making is supported by these applications of machine learning in healthcare.
Ethical Considerations and Challenges in Machine Learning for Healthcare
1. Data Privacy Concerns
Because machine learning in healthcare depends on private patient data, privacy issues are raised. To prevent breaches of this sensitive data, it is imperative to have strong security measures in place, particularly when analyzing data using predictive modeling.
2. Bias in Data
Inaccurate or unjust results may result from biased data used to train algorithms. Diverse and representative data must be provided by healthcare systems to avoid biases influencing patient treatment.
3. Accountability and Transparency
Machine learning models can occasionally behave like a "black box," making it challenging to comprehend the decision-making process. To preserve confidence in healthcare apps, there must be clear responsibility and openness.
4. Informed Consent
Patients must comprehend completely how machine learning algorithms use their data. Patients' informed permission should be obtained by healthcare practitioners who are aware of how their data is used to make medical decisions.
5. Over-reliance on Technology
Over-reliance on technology may result in mistakes or missed diagnoses, even if machine learning can aid in decision-making. Healthcare workers must utilize technology as a tool, not as a replacement for clinical judgment.
6. Regulatory Challenges
Regulations frequently don't keep up with the rapid pace of machine learning innovation in healthcare. Organizations and governments must establish policies that guarantee the moral and secure use of technology while preserving patient welfare.
The Role of Human Oversight in AI-Driven Healthcare Decision Making
In the healthcare industry, human oversight is crucial when making decisions with cutting-edge technology. Results must be reviewed and interpreted by healthcare experts, even when machine learning algorithms offer insightful information. Clinical knowledge should always be supplemented, not replaced, by applications of machine learning in healthcare.
Doctors and medical teams play a crucial role in ensuring that machine learning outputs are used effectively. By evaluating recommendations, they can consider a patient’s unique circumstances. Proper oversight helps prevent errors and enhances the quality of care in the applications of machine learning in healthcare.
Furthermore, human judgment is necessary to address ethical issues about justice and privacy. When it comes to patient rights and health outcomes, healthcare providers need to make sure that data is handled appropriately. Better decision-making is ensured and confidence is increased by this harmony between technology and human competence.
The Impact of Human-AI Collaboration on Healthcare Outcomes
1. Enhanced Diagnostic Accuracy
Collaboration between humans and AI increases diagnosis accuracy by fusing the knowledge of physicians with AI's capacity to evaluate large amounts of data. Early detection and more accurate treatment plans result from this.
2. Personalized Treatment Plans
By analyzing patient data, AI assists physicians in developing individualized treatment plans. Because of this partnership, patients can receive more efficient treatment that is customized to meet their individual requirements and medical circumstances.
3. Faster Decision-Making
Artificial Intelligence swiftly analyzes big data sets, giving physicians insightful information that helps them make quicker, better decisions. This improves overall patient care efficiency by expediting diagnosis and treatment.
4. Reducing Errors
Errors in prescriptions, medical records, and treatments can be found with AI. It reduces the possibility of errors and guarantees that patients receive the proper treatment and prescription drugs when paired with human monitoring.
5. Improved Patient Monitoring
AI continuously monitors health trends and patient vitals, warning physicians of any problems. Particularly in situations involving critical care, this teamwork enables prompt responses, improving patient outcomes.
6. Streamlined Healthcare Operations
AI streamlines hospital operations, including resource management and appointment scheduling. It improves patient care delivery, decreases wait times, and increases operational efficiency when combined with human input.
The Future of Healthcare: How AI and Machine Learning Will Evolve in the Coming Decades
1. Predictive Medicine at Scale
Machine learning in the future will allow systems to analyze massive data sets and provide early illness predictions. Better public health initiatives and preventative therapies will be possible as a result.
2. Advanced Robotic Surgery
Machine learning-enhanced robotic devices will aid in precise surgery, cutting down on mistakes and recuperation periods. Patients will benefit from less intrusive and more effective procedures because of this technology.
3. Virtual Healthcare Providers
Virtual healthcare assistants will diagnose common ailments and give medical advice around-the-clock. By enabling physicians to concentrate on complicated situations, this technology will increase access to healthcare in general.
4. Personalized Genetic Treatments
With machine learning, customized therapies based on a patient's genetic information will be possible. This will enable medical professionals to create customized treatments that focus on particular genetic disorders in order to improve results.
5. Seamless Integration of Health Data
Medical records and wearable data will be used in future healthcare systems to provide a comprehensive health picture. This will enable recommendations for therapy to be more precise and tailored to the individual.
6. Healthcare Access in Remote Areas
Machine learning and telemedicine will enable healthcare to reach faraway locations. This would close the healthcare gap by providing prompt consultations and diagnosis to patients in disadvantaged areas.
The use of machine learning in healthcare is revolutionizing our approach to treatment and has enormous potential to enhance patient outcomes. The applications of machine learning in healthcare will expand in the future, improving operational efficiency, early diagnosis, and individualized treatment plans. Its effectiveness, however, depends on protecting data privacy, resolving biases, and preserving human oversight. We can create a future in which the applications of machine learning in healthcare result in better, more accessible treatment for everyone by combining the power of technology with compassionate care. The options are genuinely endless.