The Future of Machine Learning
Discover the future of machine learning - AI advancements, predictive analytics, and automated decision-making. Unlock endless possibilities!
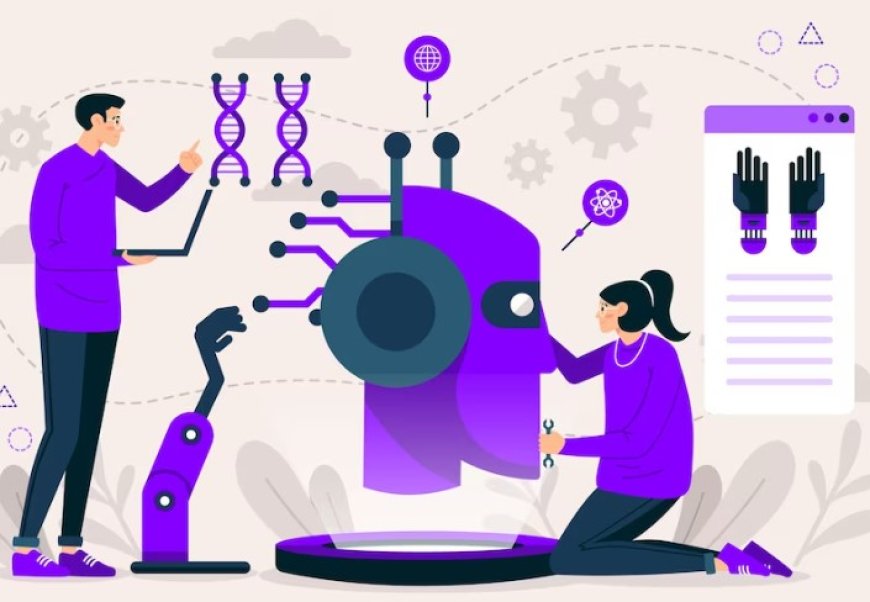
Machine learning has rapidly transformed the way we live, work, and interact with technology. From personalized recommendations on streaming platforms to self-driving cars, the applications of machine learning are seemingly limitless. But what does the future hold for this groundbreaking field? In this blog, we will explore the exciting possibilities and emerging trends that will shape the future of machine learning.
Advancements in Deep Learning
Deep learning, a subset of machine learning that mimics the human brain's neural networks, has already made significant strides. However, we can expect even more remarkable advancements in this area. Researchers are exploring ways to improve deep learning architectures, develop more efficient training algorithms, and enhance model interpretability. These advancements will enable deep learning to tackle more complex problems and provide deeper insights.
Explainable AI
As machine learning systems become increasingly autonomous and integrated into critical decision-making processes, the demand for explainable AI grows. Understanding how a machine learning model arrives at a particular prediction is crucial for building trust and accountability. Researchers are actively working on developing techniques to make AI models more transparent and interpretable, allowing users to understand the reasoning behind their decisions.
Federated Learning
Privacy concerns have led to the development of federated learning, a decentralized approach to machine learning. In this paradigm, models are trained locally on individual devices, and only aggregated updates are shared with a central server. Federated learning allows for collaborative training without sharing sensitive data, offering a promising solution for industries such as healthcare and finance
Edge Computing
With the proliferation of Internet of Things (IoT) devices, edge computing is gaining prominence. Edge computing involves processing data locally on devices rather than sending it to the cloud. This approach reduces latency and enhances real-time decision-making. Machine learning models can be deployed directly on edge devices, enabling them to perform complex tasks independently. This trend will have significant implications for industries like autonomous vehicles, robotics, and smart homes.
Ethical Considerations
As machine learning becomes increasingly embedded in our lives, addressing ethical considerations is crucial. Questions regarding bias, fairness, and accountability arise when decisions that impact individuals or communities are made by algorithms. The future of machine learning necessitates a strong focus on designing ethical frameworks, ensuring fairness, and promoting transparency in algorithmic decision-making.
Continued Collaboration and Interdisciplinarity
The future of machine learning lies in collaboration and interdisciplinarity. Machine learning researchers are working closely with experts in diverse fields such as neuroscience, psychology, and social sciences to gain a deeper understanding of human intelligence and behavior. This collaboration will drive advancements in areas like cognitive computing, human-robot interaction, and adaptive learning systems.
Reinforcement Learning and Autonomous Systems
Reinforcement learning, a branch of machine learning focused on learning through interaction and feedback, is poised to revolutionize autonomous systems. From self-driving cars to robotic assistants, reinforcement learning enables machines to learn and adapt to their environment in real-time. The future will see advancements in reinforcement learning algorithms, allowing machines to make complex decisions and navigate unpredictable scenarios independently.
Quantum Machine Learning
Quantum computing is an emerging field that promises exponential computational power. The intersection of quantum computing and machine learning opens up new possibilities for solving complex problems that are currently intractable for classical computers. Quantum machine learning algorithms will harness the unique properties of quantum systems to enhance data processing, optimization, and pattern recognition.
Interpretable and Trustworthy AI
As machine learning models become more complex and powerful, ensuring their interpretability and trustworthiness becomes paramount. Users need to understand the decision-making process of AI systems and have confidence in their outcomes. Researchers are working on developing techniques to make AI models more interpretable, allowing users to comprehend the factors influencing their predictions. Additionally, measures like model explainability, fairness, and accountability will be critical for building trust in AI systems.
Continuous Learning and Adaptive Systems
Machine learning models are typically trained on static datasets. However, the future of machine learning lies in continuous learning and adaptive systems that can dynamically update their knowledge and adapt to changing circumstances. This will enable models to learn from real-time data, stay relevant in dynamic environments, and continuously improve their performance.
Democratization of Machine Learning
As machine learning becomes increasingly prevalent, efforts are being made to democratize access to this technology. Tools and platforms are being developed to make machine learning more accessible to non-experts, allowing individuals from various backgrounds to harness its power. This democratization will empower more people to leverage machine learning in their work and contribute to the advancement of the field.
Personalization and Hyper-Personalization
In the future of machine learning, personalization will play a central role in enhancing user experiences. Machine learning algorithms will become more adept at understanding individual preferences, behaviors, and needs, allowing for highly personalized recommendations, content, and services. Hyper-personalization takes this a step further by tailoring experiences to an unprecedented level of detail, leveraging vast amounts of data and sophisticated algorithms. This level of personalization will revolutionize industries like e-commerce, marketing, and healthcare, offering customized solutions that cater to specific user requirements.
Robustness and Security
As machine learning systems become more pervasive, ensuring their robustness and security will be critical. Adversarial attacks, data poisoning, and model vulnerabilities pose significant risks to the integrity and reliability of machine learning models. Future advancements will focus on developing techniques to detect and mitigate these threats, making machine learning systems more resilient and trustworthy.
Human-Machine Collaboration
The future of machine learning is not about replacing humans but augmenting their capabilities. Human-machine collaboration will be a key focus, where machines assist humans in decision-making, automating repetitive tasks, and amplifying human creativity and problem-solving skills. The synergy between human expertise and machine learning algorithms will drive innovation and productivity across industries.
Ethical AI Governance
With the increasing influence of machine learning on society, the need for ethical AI governance becomes paramount. Regulatory frameworks, guidelines, and standards will be developed to ensure the responsible and ethical use of machine learning technologies. Transparent and accountable practices, addressing bias and discrimination, and protecting privacy will be crucial elements of ethical AI governance.
Continuous Research and Innovation
The field of machine learning is evolving at a rapid pace, and this trend will continue into the future. Continuous research and innovation will drive advancements in algorithms, models, and techniques. As new challenges arise and data availability expands, researchers and practitioners will work hand in hand to develop novel solutions and push the boundaries of what is possible.
The future of machine learning is incredibly promising and holds the potential to transform various industries and enhance our lives in numerous ways. The advancements in deep learning, explainable AI, personalization, robustness, human-machine collaboration, and ethical AI governance are paving the way for exciting developments.
As machine learning continues to evolve, it is essential to prioritize ethical considerations, promote transparency, and ensure responsible development and deployment of these technologies. Collaboration among experts from different disciplines will be crucial in addressing complex challenges and maximizing the benefits of machine learning.
In the coming years, we can expect machine learning to revolutionize sectors such as healthcare, finance, transportation, entertainment, and more. Personalized experiences, hyper-personalization, and human-machine collaboration will redefine how we interact with technology and make decisions. We must also address the robustness and security of machine learning systems to safeguard against adversarial attacks and vulnerabilities.
Furthermore, ethical AI governance will play a vital role in shaping the responsible use of machine learning, ensuring fairness, accountability, and transparency. Continuous research and innovation will drive advancements, enabling us to tackle new challenges and push the boundaries of what is possible.