The Role of Sentiment Analysis in Data Analytics
Explore the crucial role of sentiment analysis in data analytics. Learn how sentiment analysis unlocks valuable insights from text data, business decisions.
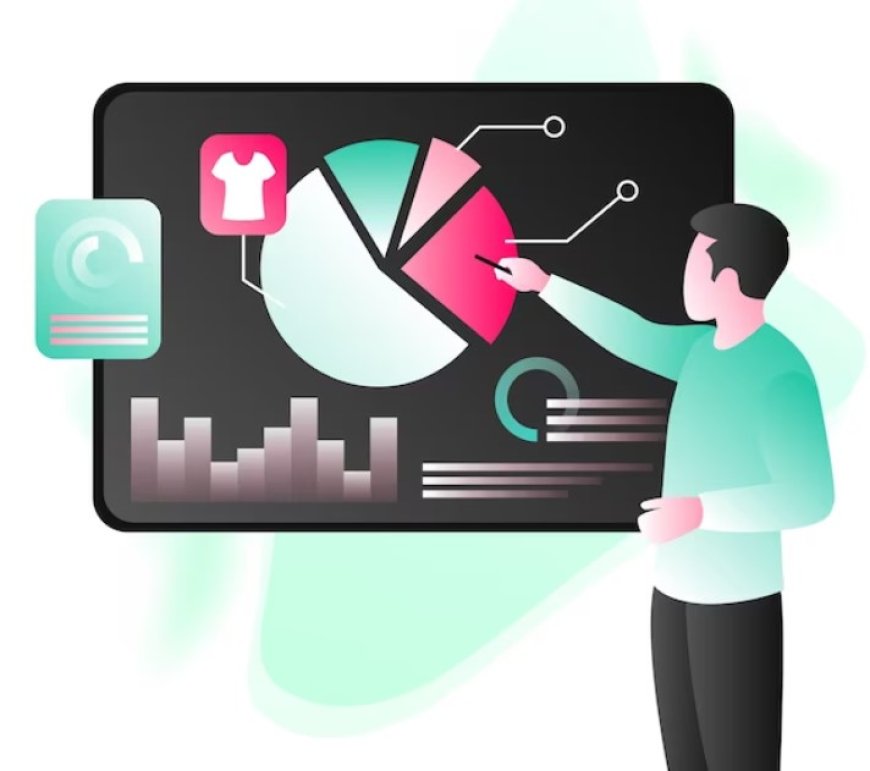
In the realm of data analytics, the power of understanding human emotions cannot be understated. The explosion of digital communication has provided a treasure trove of textual data, ranging from social media posts and customer reviews to news articles and chat logs. Within this vast sea of text lies valuable information about how people feel and perceive the world around them. This is where sentiment analysis comes into play. Sentiment analysis, a subfield of natural language processing (NLP), is the art and science of extracting sentiments, opinions, and emotions from text data. It serves as a crucial tool in the data analyst's arsenal, offering insights that are indispensable for informed decision-making, customer-centric strategies, and proactive reputation management.
Significance of Sentiment Analysis in Data Analytics
The significance of sentiment analysis in data analytics cannot be overstated, as it plays a pivotal role in extracting valuable insights from textual data, ultimately aiding in informed decision-making. This technique allows organizations to delve into the sentiments expressed by individuals in various contexts, offering a deeper understanding of public opinion and customer preferences.
One of the primary aspects of sentiment analysis's significance lies in its capacity to support decision-making processes. By analyzing sentiments expressed in customer reviews, social media posts, or even financial news articles, businesses can make data-driven decisions. For instance, a company can gauge public sentiment towards a new product launch, enabling them to adapt marketing strategies or product features accordingly. Similarly, sentiment analysis is crucial in the finance industry, where understanding market sentiment can help traders and investors make timely and profitable decisions.
Furthermore, sentiment analysis provides invaluable customer insights. By analyzing customer feedback, companies can gain a comprehensive view of customer satisfaction, identifying areas of improvement and tailoring their products and services accordingly. This not only enhances customer experience but also contributes to customer retention and loyalty.
In addition, sentiment analysis plays a vital role in reputation management. Brands and organizations can monitor and manage their public perception effectively. In the age of social media, a single negative tweet or review can go viral and damage a brand's reputation. Sentiment analysis tools can help organizations detect and address potential PR crises early, allowing them to respond proactively and mitigate reputational damage.
Techniques and Approaches in Sentiment Analysis
Sentiment analysis employs various techniques and approaches to extract valuable insights from textual data, gauging the emotions, opinions, and attitudes expressed within. One prominent avenue is the utilization of machine learning methods, where algorithms are trained to classify text into different sentiment categories such as positive, negative, or neutral. Supervised learning, a common technique, involves training the model on labeled datasets, enabling it to recognize patterns and sentiments in new, unseen data. Conversely, unsupervised learning techniques, such as clustering or topic modeling, uncover sentiment without predefined labels, making them effective for large-scale sentiment analysis.
Natural Language Processing (NLP) plays a pivotal role in sentiment analysis by providing tools to process and understand human language. Techniques like tokenization break down text into individual units, enabling the analysis of sentiment on a granular level. Moreover, advanced NLP models, including language models like BERT or GPT, contribute to more nuanced sentiment analysis by capturing context, semantics, and intricate language structures.
Sentiment analysis tools and platforms offer practical solutions for businesses and researchers. These tools often incorporate a combination of machine learning and NLP techniques, providing user-friendly interfaces for sentiment analysis tasks. Some platforms allow customization to fit specific industry needs, making them versatile for applications ranging from social media monitoring to customer feedback analysis.
Challenges in Sentiment Analysis
Sentiment analysis, despite its significant utility, is not without its challenges. One of the foremost obstacles lies in the inherent ambiguity of language. Human communication often involves sarcasm, irony, and nuanced expressions that can confound sentiment analysis algorithms. Distinguishing between positive and negative sentiments becomes particularly challenging when faced with linguistic subtleties. Additionally, the presence of ambiguous terms and phrases makes it difficult for algorithms to discern the true sentiment behind a statement accurately.
Multilingual sentiment analysis poses another formidable challenge. In a globalized world, data analytics often involves processing information in multiple languages. Different languages have unique structures and cultural nuances, adding complexity to sentiment analysis tasks. Overcoming language diversity requires sophisticated algorithms capable of understanding sentiments across various linguistic contexts, ensuring the applicability of sentiment analysis on a global scale.
Real-time analysis is a critical aspect of sentiment analysis, especially in industries where immediate insights can impact decision-making. However, processing sentiments in real-time data streams presents challenges such as the need for rapid analysis without compromising accuracy. Striking a balance between speed and precision is crucial, necessitating the development of algorithms capable of delivering timely sentiment insights in dynamic and fast-paced environments.
Future Trends in Sentiment Analysis
Sentiment analysis, a field of natural language processing, is continuously evolving with emerging technologies and novel approaches. The future of sentiment analysis promises several exciting developments that will enhance its accuracy, applicability, and ethical considerations.
Advanced Machine Learning Models
The future of sentiment analysis is poised to benefit from advanced machine learning models. One notable trend is the integration of deep learning techniques, such as neural networks, to improve accuracy. These models can capture more complex linguistic patterns and nuances in text data, making sentiment analysis more precise and reliable. Additionally, researchers and practitioners are exploring cutting-edge models tailored specifically for sentiment analysis, harnessing the power of transformers and other state-of-the-art architectures.
Integration with Other Data Analytics Techniques
Sentiment analysis is no longer a standalone process but is increasingly integrated with other data analytics techniques. Predictive analytics, for instance, can be combined with sentiment analysis to forecast market trends and consumer behavior more accurately. By synergizing sentiment analysis with data visualization tools, businesses and researchers can gain comprehensive insights into public opinion and sentiment, enabling them to make data-driven decisions effectively.
Ethical Considerations
As sentiment analysis continues to grow in importance, addressing ethical considerations becomes paramount. The field must grapple with biases in sentiment analysis algorithms, ensuring that they do not perpetuate or amplify existing prejudices. Moreover, the responsible use of sentiment analysis in various domains is a crucial trend. Striking a balance between leveraging sentiment analysis for decision-making and respecting privacy and data protection regulations is essential to prevent misuse and maintain public trust.
sentiment analysis plays a pivotal role in the realm of data analytics. By harnessing the power of natural language processing and machine learning, it enables organizations to extract valuable insights from textual data, understand public opinion, and make data-driven decisions. Whether used in market research, social media monitoring, customer feedback analysis, or brand reputation management, sentiment analysis empowers businesses to stay ahead of the curve and adapt to changing trends and sentiments. In an era where data is king, sentiment analysis emerges as a critical tool for extracting actionable intelligence from the vast sea of textual information, driving innovation, and enhancing decision-making processes.