Exploring Deep Learning: The Power of Neural Networks and Their Applications
The Power of Neural Networks and Their Applications.' Explore neural networks, their real-world applications, and unleash the transformative capabilities of AI.
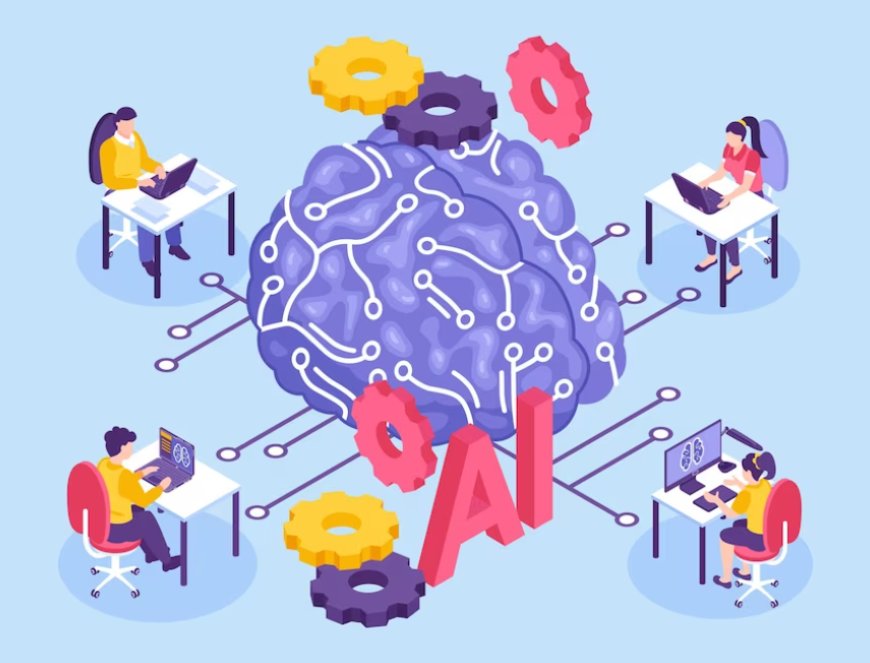
In the ever-evolving realm of artificial intelligence, one paradigm stands out as a true game-changer: deep learning. This groundbreaking approach mirrors the human brain's capacity to process information and learn from it. At its core are neural networks, intricate algorithms designed to solve complex problems and extract patterns from data.
Deep Learning and Neural Networks
Deep learning, a subset of machine learning, revolves around training algorithms to make intelligent decisions by learning from vast datasets. Central to this field are neural networks, designed to mimic the interconnected neurons in the human brain. Neural networks consist of layers of nodes or "neurons," each responsible for processing and transforming data as it flows through the network.
These networks come in various types, each tailored for specific tasks. Convolutional Neural Networks (CNNs) excel in image recognition, Natural Language Processing (NLP) relies on Recurrent Neural Networks (RNNs) for sequential data, and Generative Adversarial Networks (GANs) create data instances resembling existing ones. Neural networks have applications across industries, from computer vision and healthcare to finance and manufacturing.
Despite their remarkable capabilities, neural networks pose challenges such as interpretability and the need for large labeled datasets. Researchers are actively working to address these challenges and enhance the efficiency and transparency of deep learning models, ensuring they remain at the forefront of AI innovation.
Layers of Learning: Types of Neural Networks
Within the expansive realm of deep learning, various types of neural networks have evolved to tackle diverse tasks. These neural network architectures are meticulously designed to cater to specific challenges and domains, each bringing a unique set of capabilities to the table.
Convolutional Neural Networks (CNNs) excel in image recognition and computer vision tasks. Their hierarchical structure enables them to automatically learn and identify patterns, shapes, and features within images, making them indispensable in applications like facial recognition, object detection, and even autonomous vehicles.
Recurrent Neural Networks (RNNs) are tailored for handling sequential data, making them essential in Natural Language Processing (NLP) tasks. They possess the ability to capture dependencies and context within sequences, enabling tasks such as language modeling, sentiment analysis, and machine translation.
Generative Adversarial Networks (GANs), a pioneering innovation, consist of two networks: a generator and a discriminator. GANs are used to generate synthetic data that closely resembles real data. This is particularly valuable in creating realistic images, video synthesis, and even generating art.
Each type of neural network boasts its unique architecture and strengths, allowing deep learning to offer versatile solutions across a myriad of industries and applications. These neural networks, in tandem with vast datasets and powerful computational resources, continue to shape the future of AI and data-driven decision-making.
Applications Across Industries
-
Computer Vision: CNNs have revolutionized image analysis, enabling machines to identify objects, faces, and even emotions in images and videos. From self-driving cars to medical image analysis, computer vision powered by deep learning enhances accuracy and efficiency.
-
Natural Language Processing (NLP): RNNs and transformer models, such as BERT, have unlocked machines' potential to understand, generate, and translate human language. This has led to advancements in sentiment analysis, chatbots, language translation, and more.
-
Healthcare: Deep learning is making significant strides in medical diagnosis, assisting doctors in detecting diseases from medical images with remarkable accuracy. It's also enabling the discovery of new drug compounds and predicting patient outcomes based on medical data.
-
Finance: Neural networks are employed to predict stock prices, detect fraudulent transactions, and optimize trading strategies by analyzing vast financial datasets.
-
Manufacturing: Deep learning aids in quality control by identifying defects in manufacturing processes. It also enables predictive maintenance, reducing downtime by forecasting equipment failures.
Challenges and Future Directions
Despite its remarkable achievements, deep learning faces challenges. Neural networks demand massive labeled datasets, and their intricate architectures can sometimes be challenging to interpret, raising concerns about transparency and accountability.
In the future, researchers aim to enhance the efficiency of deep learning models, fortify them against adversarial attacks, and improve their interpretability. Combining deep learning with other AI techniques, such as reinforcement learning, holds promise for creating even more sophisticated and intelligent systems.
The Evolution Continues: Challenges and Ethical Considerations
While the potential of deep learning and neural networks is undeniable, there are challenges and ethical considerations that must be addressed. One major challenge is the "black box" nature of many deep learning models. Understanding why a neural network arrives at a particular decision can be complex, raising concerns about transparency and accountability, particularly in critical applications like healthcare and finance. Researchers are actively working on techniques to make these models more interpretable without compromising their performance.
Another consideration is the reliance on large amounts of data for training. While data availability is increasing, concerns about data privacy, bias, and the potential for perpetuating societal inequalities must be carefully navigated. Ethical frameworks are being developed to ensure that AI systems, powered by deep learning, are used responsibly and fairly.
Collaboration and the Future
The future of deep learning hinges on collaboration between researchers, practitioners, and industries. As the field advances, interdisciplinary collaborations will become indispensable. Experts from fields such as neuroscience, psychology, and ethics will contribute to shaping the direction of deep learning research.
Furthermore, the synergy between deep learning and other AI techniques, like reinforcement learning and unsupervised learning, will pave the way for even more sophisticated AI systems. Hybrid models that combine the strengths of various approaches will likely emerge, creating AI systems that are adaptable, efficient, and capable of nuanced decision-making.
The Next Generation of AI Innovators
As deep learning continues to advance, it becomes more accessible to developers, researchers, and students alike. A plethora of open-source libraries, online courses, and research papers contribute to a thriving community of AI enthusiasts. This democratization of knowledge empowers individuals to explore, experiment, and contribute to the ongoing evolution of AI technologies.
The Pragmatic Benefits of Deep Learning in Everyday Life
Deep learning's impact extends far beyond specialized fields, permeating everyday life in ways we might not even realize. Here are some additional areas where it's making a tangible difference:
-
E-commerce and Recommendation Engines: When you see personalized product recommendations on platforms like Amazon or Netflix, you're witnessing the power of deep learning. These algorithms analyze your past behavior to suggest items or content that align with your preferences.
-
Autonomous Vehicles: Deep learning is a cornerstone of self-driving cars. Neural networks process sensor data to recognize road signs, pedestrians, and other vehicles, enabling autonomous vehicles to navigate safely.
-
Virtual Assistants: Siri, Google Assistant, and Alexa owe their conversational abilities to deep learning. These AI-powered virtual assistants use natural language processing models to understand and respond to voice commands.
-
Social Media: Social media platforms use deep learning for content recommendation, image recognition, and even content moderation, enhancing the user experience and ensuring safer online spaces.
-
Gaming: Deep learning has enriched the gaming experience through advanced graphics rendering, character behavior modeling, and even creating nonplayer characters (NPCs) with humanlike responses.
The Exciting Path Ahead
The exciting path ahead for deep learning and neural networks promises continued innovation and transformation across industries. As researchers delve deeper into the intricacies of these neural architectures, we can anticipate breakthroughs in interpretability, efficiency, and robustness. Moreover, the fusion of deep learning with other AI paradigms will likely lead to the development of highly adaptable and intelligent systems, opening doors to new possibilities. With ethical considerations guiding these advancements, the future of deep learning holds the potential to reshape how we interact with technology and harness its power for the betterment of society.
In this exciting journey, the next generation of AI innovators will play a pivotal role. The democratization of knowledge and the accessibility of resources empower individuals to contribute to this transformative field. Whether you're a student, a researcher, or a curious enthusiast, the world of deep learning invites you to explore its possibilities, tackle its challenges, and be a part of the AI revolution that's shaping the world as we know it.