Bias in AI: Unpacking the Issue of Fairness and Bias in Machine Learning Algorithms
Explore the complex issue of fairness and bias in machine learning algorithms. Understand the impact on AI systems and learn strategies to mitigate bias.
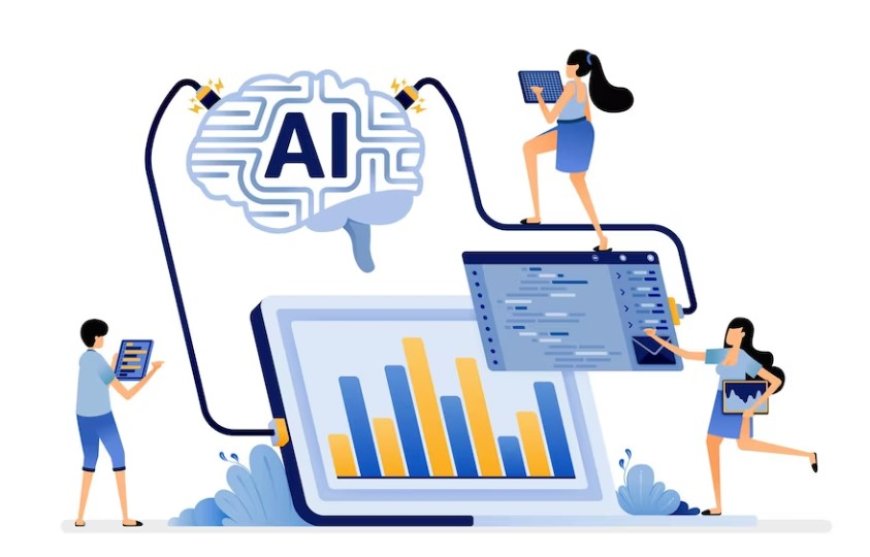
The rapid integration of artificial intelligence into various aspects of our lives has brought to the forefront an urgent concern: bias in AI systems. These systems, while powerful, can inadvertently perpetuate and even amplify societal biases present in their training data and algorithms. Fairness in AI is not just an ethical aspiration, but a critical necessity to prevent discrimination and uphold justice. This exploration delves into the multifaceted issue of bias in machine learning, highlighting its types, causes, real-world implications, and strategies to mitigate its impact.
Bias in AI
Bias in AI refers to the presence of unfair or unjustified outcomes in machine learning algorithms that systematically favor or discriminate against certain individuals, groups, or characteristics. This bias can emerge from various sources, such as biased training data, flawed algorithm design, or the implicit biases of developers. It undermines the equitable and unbiased functioning of AI systems, potentially perpetuating societal inequalities and ethical concerns. Addressing bias in AI is crucial to ensure that these systems produce just and equitable results for all users and stakeholders.
Types of Bias in AI
Data Bias: Data bias refers to the presence of unequal representation or skewed data points in the training dataset used for developing AI models. This can stem from historical inequalities, underrepresentation, or overrepresentation of certain groups. For instance, if a facial recognition algorithm is trained predominantly on data from a particular ethnicity, it may struggle to accurately recognize faces from other ethnicities. Biased data can significantly distort AI outcomes, leading to unfair or discriminatory results. For example, a loan approval system trained on biased data might unfairly deny loans to deserving individuals based on factors such as race or gender.
Algorithmic Bias: Algorithmic bias occurs when the design and operation of an AI model itself perpetuate or amplify existing biases in data. This can happen due to various reasons, including biased training data, flawed model architectures, or inappropriate feature selection. The consequences of algorithmic bias can be profound, as biased algorithms may reinforce stereotypes, exclude certain groups, or amplify existing disparities. An example of algorithmic bias is the "compas" algorithm used in criminal justice systems, which was found to predict higher recidivism rates for certain racial groups, leading to unjust sentencing decisions.
Socioeconomic Bias: Socioeconomic factors can significantly contribute to bias in AI systems. Economic disparities and societal inequalities can lead to uneven access to resources, education, and opportunities. When AI algorithms are trained on data that reflects these disparities, they can perpetuate the existing biases. This often results in AI systems favoring certain socioeconomic groups over others. The impact is particularly pronounced for marginalized communities that already face systemic challenges. For instance, a job recommendation algorithm might inadvertently favor candidates from privileged socioeconomic backgrounds, exacerbating inequality in employment opportunities.
Real-world Examples of Bias in AI
Criminal Justice System:
In the context of the criminal justice system, AI's involvement in predictive policing has raised concerns about reinforcing racial biases. Algorithms trained on historical crime data can perpetuate biases, leading to over-policing in certain communities. Moreover, studies have shown disparities in sentencing decisions influenced by AI, with minority individuals often receiving harsher outcomes. Addressing these issues requires a comprehensive examination of the data and algorithms to ensure fair treatment for all.
Hiring and Recruitment:
Biased AI algorithms have infiltrated hiring and recruitment practices, amplifying gender and racial biases. These algorithms can inadvertently favor candidates from specific demographics, resulting in unequal opportunities for job seekers. It is essential to confront these biases head-on by scrutinizing the data used to train these algorithms and implementing corrective measures. Striving for equitable hiring processes through unbiased technology can lead to more inclusive workplaces.
Factors Contributing to Bias
Biased Training Data: Biased training data can arise from the homogeneity of data sources, resulting in skewed representations. Inaccurate or mislabeled data further compounds this issue, perpetuating biases within AI models.
Lack of Diversity in Development Teams: The composition of development teams plays a pivotal role in AI bias. Teams lacking diversity can inadvertently embed their own biases into algorithms. To mitigate this, embracing multidisciplinary teams with varied perspectives is crucial for cultivating fair and unbiased AI systems.
The Ethical Implications of Bias in AI
Reinforcing Prejudices and Discrimination: Bias in AI systems can inadvertently perpetuate and even amplify existing societal prejudices and discrimination. When algorithms reflect historical biases present in training data, they can inadvertently contribute to the marginalization of certain groups. This not only raises ethical concerns but also reinforces harmful stereotypes, hindering progress toward a more just and equitable society.
Impact on Decision-making Processes: AI-driven decisions hold significant influence across various domains, from criminal justice to healthcare and finance. The presence of bias in these decisions can lead to unfair outcomes, denying opportunities or resources to individuals solely based on their demographic characteristics. This challenges the fundamental principles of fairness and justice, requiring careful consideration of bias-related ethical concerns.
Trust and Transparency Issues: Trust is vital for the acceptance and adoption of AI technologies. When bias is introduced, it erodes trust in AI systems. Users and stakeholders may question the fairness and intentions behind algorithmic decisions. Lack of transparency regarding the functioning of AI models can exacerbate this issue. Ethical implications arise not only from the bias itself but also from the opacity that often surrounds its existence in AI systems.
Future Directions
Emerging Technologies for Bias Mitigation
As technology evolves, new tools and approaches are emerging to help mitigate bias in AI. These include advanced fairness-aware algorithms, more comprehensive data auditing techniques, and innovative strategies for transparent decision-making processes. Keeping an eye on these emerging technologies will be essential in the ongoing effort to make AI systems fairer and more reliable.
Potential Impact on AI Development
The adoption of bias mitigation technologies will significantly influence the development of AI systems. By addressing bias, we can expect AI to become more inclusive, trustworthy, and respectful of diverse user needs and perspectives. The integration of these technologies will likely shape the next phase of AI development, emphasizing the importance of fairness and ethical AI practices.
Addressing Bias in AI
Addressing Bias in AI requires a multi-faceted approach. Ethical guidelines and principles serve as a foundational framework, guiding developers and organizations towards responsible AI deployment. Fairness-aware algorithms and techniques play a crucial role in identifying and mitigating biases within machine learning models. Additionally, integrating human-in-the-loop approaches ensures that human judgment and oversight are incorporated, providing a necessary counterbalance to automated decision-making processes. This combination of ethical guidance, technical refinement, and human involvement forms a robust strategy for combating bias in AI systems.
The issue of bias in AI is a multifaceted challenge with profound implications across various sectors. Recognizing the different forms of bias—data, algorithmic, and developer-induced—highlights the complexity of the problem. Addressing bias demands a combination of technical strategies, transparent practices, diverse development teams, and a commitment to ethical considerations. As AI continues to shape our lives, understanding and mitigating bias becomes paramount to fostering fairness, inclusivity, and social responsibility in the development and deployment of machine learning algorithms.