A Beginner-Friendly Overview of What Data Science Is, Its Importance, and Its Applications in Various Fields
Explore the world of Data Science with this beginner-friendly guide. Learn what Data Science is and its diverse applications across various industries.
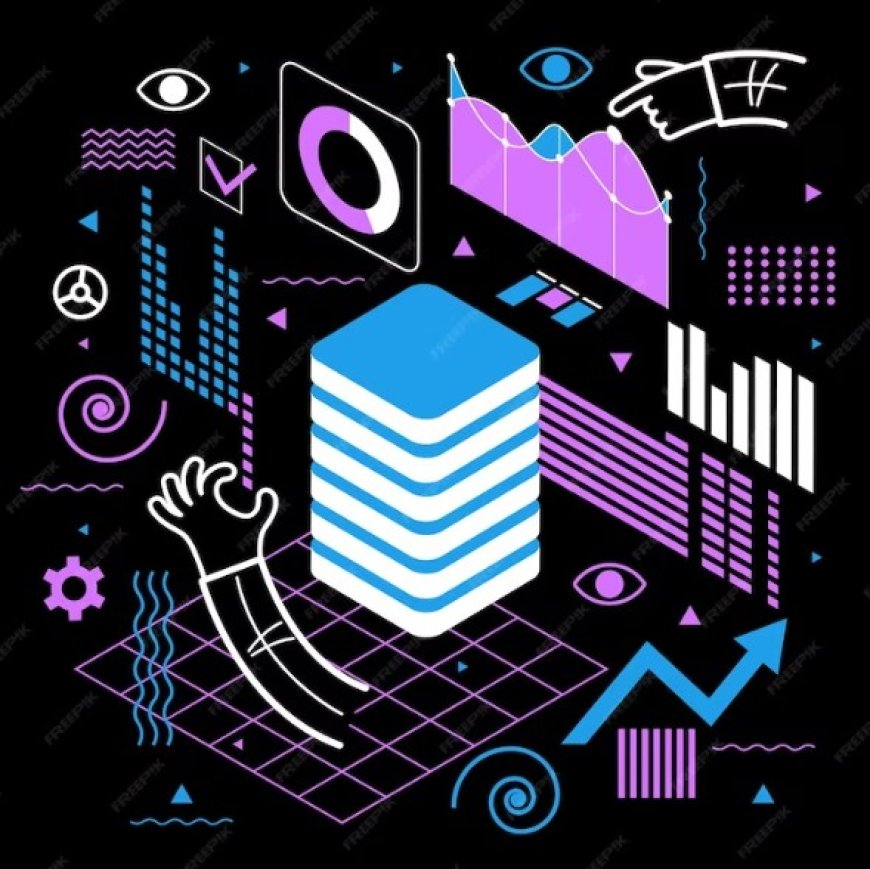
In today's digital era, data science stands as a guiding light in the midst of vast and complex data landscapes. It's more than just numbers and charts—it's the art and science of transforming raw information into meaningful insights. As we navigate this information-rich world, understanding what data science is, its crucial role, and its diverse applications becomes essential. Let's embark on a beginner-friendly journey to uncover the essence of data science and its far-reaching impact.
Data Science
Data science is the art and science of extracting valuable insights and knowledge from raw data using a combination of techniques and tools. It involves transforming complex and unstructured data into understandable information that can drive informed decision-making. Through data science, we gain the power to unravel hidden patterns, trends, and correlations within the data, enabling us to make predictions and optimize outcomes.
Core Components of Data Science
Data science draws upon three foundational components that work in tandem to create meaningful results:
-
Domain Knowledge: A deep understanding of the specific field or industry you're working with. This knowledge is crucial for formulating relevant questions, interpreting results, and making informed decisions based on the insights gained from data analysis.
-
Programming and Data Manipulation Skills: Proficiency in programming languages such as Python or R is essential for efficiently handling and transforming data. These skills enable data scientists to clean, preprocess, and organize data into a usable format for analysis.
-
Statistical and Mathematical Expertise: Data science heavily relies on statistical techniques and mathematical models to extract meaningful insights from data. This expertise helps in choosing the right methods for analysis, assessing model performance, and drawing accurate conclusions.
The synergy of these three components allows data scientists to navigate the complexities of data, extract meaningful patterns, and provide valuable solutions to a wide range of real-world challenges.
Importance of Data Science
Data science plays a pivotal role in extracting valuable insights from vast and complex datasets. By harnessing the power of data analysis, it enables informed decision-making, predictive modeling, and innovative problem-solving across industries. Businesses use data science to optimize operations, improve customer experiences, and drive growth. In research, it aids in understanding patterns and trends, accelerating scientific discoveries. Furthermore, data science's ability to address social challenges and inform policy decisions underscores its vital importance in shaping a data-driven world.
Tools and Technologies
In the realm of data science, proficiency with essential tools and technologies is paramount. Firstly, languages like Python and R are foundational. Python, known for its versatility and extensive libraries, is widely favored for data manipulation and analysis. R, on the other hand, is highly specialized for statistical computing and visualization.
Next, data analytics software, such as Tableau and Power BI, facilitate intuitive data exploration and visualization, enabling insights to be communicated effectively. These tools are instrumental in turning complex datasets into actionable information.
Furthermore, machine learning libraries like TensorFlow, scikit-learn, and PyTorch provide the necessary frameworks for building and deploying machine learning models. These libraries streamline the process, making it more accessible for data scientists to develop predictive models and derive meaningful insights from data.
Proficiency in these tools and technologies equips data scientists with the capabilities to tackle complex problems and extract valuable insights from data.
Data Science Workflow
Data science involves a systematic approach to extracting insights from data, guiding decision-making, and solving complex problems. The workflow encompasses several stages, each contributing to the overall success of the process.
-
Problem Formulation: At the outset, data scientists define the problem they aim to solve or the questions they want to answer using data. A clear problem statement helps guide subsequent stages and ensures alignment with goals.
-
Data Collection: Relevant data is gathered from various sources, such as databases, APIs, or surveys. Quality data is essential for meaningful results, so careful selection and consideration of data sources is crucial.
-
Data Cleaning and Preprocessing: Raw data often contains errors, inconsistencies, and missing values. This stage involves refining the data by addressing these issues, ensuring it's ready for analysis.
-
Exploratory Data Analysis (EDA): Before diving into complex modeling, data scientists explore the data visually and statistically. This step helps uncover patterns, trends, correlations, and potential outliers, providing initial insights.
-
Modeling: Using machine learning algorithms, statistical techniques, or other analytical methods, data scientists build models to predict outcomes, classify data, or uncover relationships within the data.
-
Evaluation: Models are assessed based on their performance using appropriate metrics. This step determines how well the model is likely to generalize to new, unseen data.
-
Refinement and Iteration: Data science is iterative. Insights gained from the evaluation stage lead to refining the model, adjusting parameters, or choosing different algorithms. This process continues until satisfactory results are achieved.
-
Deployment: Once a model is finalized, it's deployed into real-world applications, where it can provide value and support decision-making. This may involve integrating the model into software systems or platforms.
Throughout the entire workflow, data science is characterized by its iterative nature. Insights gained from each stage inform decisions in subsequent stages. This cyclic approach allows for continuous improvement, ensuring the model's effectiveness and relevance.
Applications For Data Science
-
Business and Marketing: Data science drives business decisions by analyzing customer behavior and preferences. Through techniques like customer segmentation and predictive analytics, companies can tailor marketing strategies to specific audiences. Recommendation systems, as seen on streaming platforms, suggest products or content based on users' past interactions, boosting engagement and sales.
-
Healthcare: Data science revolutionizes healthcare through personalized treatment plans and predictive modeling. Machine learning algorithms can analyze patient data to predict disease outcomes, aiding doctors in making informed decisions. In drug discovery, data-driven insights accelerate the identification of potential compounds and streamline the research process.
-
Finance: In the finance sector, data science underpins risk assessment and fraud detection. Algorithms analyze transaction patterns to detect anomalies and potential fraudulent activities, safeguarding financial institutions and their clients. Additionally, quantitative trading models use historical market data to optimize investment strategies.
-
Social Sciences: Data science assists in understanding human behavior and sentiment on social platforms. Sentiment analysis gauges public opinion about products, services, or events, helping companies adapt their strategies. Social network analysis uncovers patterns in connections, influencing marketing campaigns and policy decisions.
-
Environmental Science: Data science aids environmental monitoring and sustainability efforts. Climate modeling analyzes historical data to predict future weather patterns and trends. Optimization algorithms can be applied to resource management, optimizing water usage, energy consumption, and waste reduction.
-
Government and Policy: Data science enhances decision-making in governance and policy-making. Crime prediction models help law enforcement allocate resources efficiently. Urban planning benefits from analyzing data to design more livable cities with improved infrastructure and transportation systems. Public health management employs data to respond to epidemics and optimize healthcare services.
Challenges and Ethical Considerations
Data science is not without its challenges. Ensuring data quality, dealing with missing or biased data, and managing the complexities of large datasets are hurdles that practitioners often face. Moreover, ethical considerations are paramount. Safeguarding user privacy, addressing potential bias in algorithms, and responsibly handling sensitive information are essential for maintaining trust and avoiding harmful consequences. As data science continues to shape our world, addressing these challenges and upholding ethical standards remain critical aspects of the field's growth and impact.
Future Trends in Data Science
As data science continues to evolve, several exciting trends are shaping its future. Artificial intelligence and machine learning are advancing rapidly, enabling more sophisticated and accurate predictive models. Deep learning techniques are making breakthroughs in areas like image recognition and natural language processing. Additionally, the growing availability of big data and improved data processing technologies is paving the way for deeper insights. As data privacy and ethics gain prominence, responsible data handling and unbiased algorithms are becoming key considerations. The future of data science holds the promise of even more innovative solutions across industries, making it an exciting field to watch and be a part of.
Data science is the dynamic practice of extracting insights from data through a combination of domain expertise, programming skills, and statistical knowledge. Its importance lies in its ability to drive informed decisions across industries and fields, from business optimization to healthcare breakthroughs. Data science empowers us to predict outcomes, discover trends, and solve complex challenges. What's even more exciting is the democratization of data science tools and resources, enabling individuals from various backgrounds to engage with this transformative field. I encourage you to delve deeper, acquire new skills, and recognize the potential of data science in shaping your own journey and contributing to a data-driven world.