The Scope Of Data Analytics
Explore the vast scope of data analytics in diverse industries. Discover its impact on decision-making, predictive modeling, and operational efficiency. Stay ahead with this comprehensive overview of data analytics opportunities.
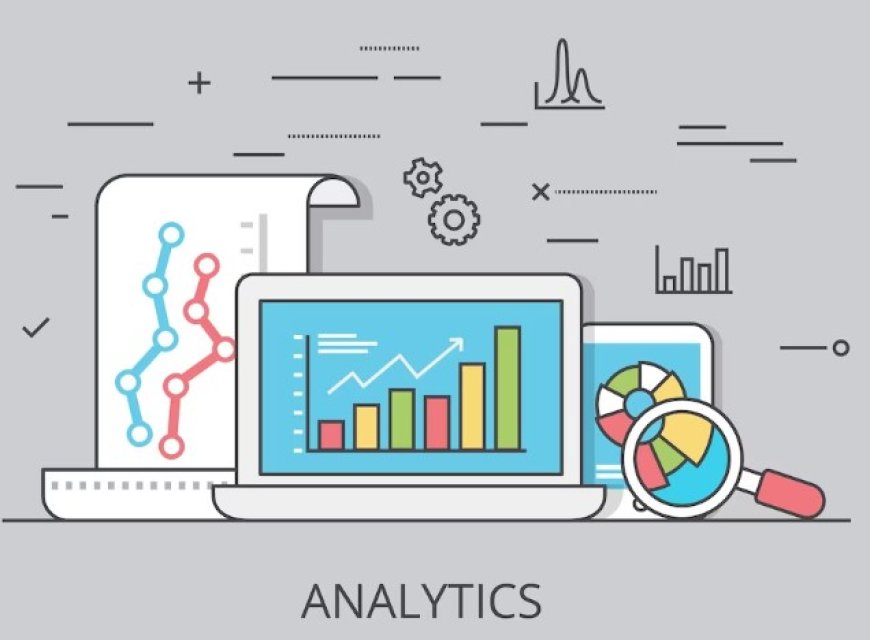
Data analytics, the process of examining and interpreting data sets to draw meaningful insights, plays a pivotal role across diverse industries. Its significance lies in its ability to uncover patterns, trends, and valuable information from vast datasets, empowering organizations to make data-driven decisions for improved performance and competitiveness. This introduction sets the stage for exploring the wide-ranging applications and implications of data analytics in the modern world.
Types of Data Analytics
Data analytics encompasses four primary types, each serving distinct purposes:
Descriptive Analytics offers a retrospective view, summarizing historical data to provide insights into what has happened. It forms the foundation by presenting trends, patterns, and key metrics, aiding in understanding past performance.
Diagnostic Analytics delves deeper into data to uncover the reasons behind past events or trends. It focuses on identifying the root causes of specific outcomes, allowing organizations to rectify issues or replicate successes.
Predictive Analytics employs statistical algorithms and machine learning techniques to forecast future trends based on historical and current data. By recognizing patterns, it aids in anticipating potential outcomes, thus enabling proactive decision-making.
Prescriptive Analytics takes the analysis a step further, not only predicting future scenarios but also offering recommended actions to optimize outcomes. It leverages advanced algorithms and optimization techniques, providing actionable insights for making informed choices.
Each type of data analytics serves a crucial role in extracting meaningful information from data, contributing to enhanced decision-making processes across various industries.
Applications of Data Analytics
Data analytics finds extensive applications across various industries, enabling organizations to derive valuable insights and make informed decisions:
-
Business Intelligence and Reporting: Data analytics aids in generating comprehensive reports and dashboards, allowing businesses to monitor key performance indicators, track trends, and gain a competitive edge.
-
Customer Relationship Management (CRM): By analyzing customer data, organizations can personalize marketing strategies, enhance customer experiences, and improve customer retention rates.
-
Financial Analysis and Risk Management: In the finance sector, data analytics helps detect fraudulent activities, assess investment risks, and optimize trading strategies.
-
Healthcare and Life Sciences: Healthcare professionals utilize data analytics to improve patient outcomes, manage healthcare costs, and streamline operations through predictive analytics and patient data analysis.
-
Marketing and Sales Optimization: Marketers leverage data analytics to target the right audience, optimize ad campaigns, and measure marketing ROI, ultimately boosting sales and revenue.
-
Supply Chain and Operations Management: Data analytics optimizes supply chain logistics, inventory management, and production processes, reducing costs and improving overall efficiency.
These applications highlight the versatility and significance of data analytics in today's data-driven world, impacting diverse sectors and facilitating data-informed decision-making.
Tools and Technologies for Data Analytics
Data analytics relies heavily on a range of tools and technologies to process, analyze, and extract insights from large datasets. These tools play a crucial role in making data-driven decisions. Here are some key components:
-
Data warehousing solutions like Amazon Redshift, Google BigQuery, and Snowflake provide the infrastructure for storing and managing vast amounts of structured data, enabling efficient querying and analysis.
-
Algorithms and libraries such as scikit-learn, TensorFlow, and PyTorch are essential for predictive analytics, classification, regression, and clustering tasks, allowing organizations to uncover patterns and make predictions.
-
Tools like Tableau, Power BI, and D3.js enable the creation of interactive and visually appealing dashboards and reports. They help stakeholders understand complex data by presenting it in a comprehensible manner.
-
BI software such as QlikView, MicroStrategy, and Looker facilitates ad-hoc querying and reporting, allowing users to explore data and gain insights without needing extensive technical expertise.
-
Technologies like Hadoop, Apache Spark, and Apache Kafka are vital for handling massive volumes of data, processing it in parallel, and supporting real-time data streaming, making them valuable for data analytics at scale.
These tools, when used effectively, empower organizations to harness the potential of their data, drive informed decision-making, and gain a competitive edge in various industries.
Challenges in Data Analytics
Challenges in Data Analytics encompass a range of hurdles that organizations face in harnessing data effectively. Data quality and cleansing present significant issues, as ensuring accurate, consistent data is crucial for meaningful insights. Privacy and security concerns arise with the increasing volume of sensitive information being processed. Scalability and performance become critical as datasets grow larger and more complex. Additionally, addressing the skill gap and talent shortage in the field remains a persistent challenge, requiring ongoing efforts in training and development. Overcoming these obstacles is essential for maximizing the value of data analytics in any organization.
Future Trends in Data Analytics
Artificial Intelligence and Machine Learning Integration
As data analytics continues to evolve, the integration of artificial intelligence (AI) and machine learning (ML) is set to play a pivotal role. AI and ML algorithms can enhance data analytics by automating insights, improving prediction accuracy, and uncovering hidden patterns in large datasets. This trend will empower businesses to make more informed decisions and drive innovation in various industries.
Edge Analytics
Edge analytics is poised to become a crucial component of data analytics. With the proliferation of IoT devices and sensors at the edge of networks, processing data closer to its source can reduce latency and enable real-time decision-making. This trend will be instrumental in fields like autonomous vehicles, manufacturing, and smart cities, where timely insights are imperative.
Blockchain and Data Security
Data security and integrity are paramount in data analytics. Blockchain technology is emerging as a solution to ensure data transparency, immutability, and secure transactions. Integrating blockchain into data analytics can enhance trust and privacy, particularly in sectors like finance, healthcare, and supply chain management.
Real-time Analytics
The demand for real-time analytics is on the rise as businesses seek to respond to events and trends as they happen. Real-time analytics enables immediate actions and insights, crucial in applications such as fraud detection, e-commerce, and social media monitoring. Advancements in data processing and streaming technologies will drive this trend forward, revolutionizing decision-making processes.
These future trends in data analytics will shape the way organizations derive value from their data and stay competitive in an increasingly data-driven world.
Ethical Considerations in Data Analytics
Ethical considerations play a critical role in the practice of data analytics. It is imperative to address issues surrounding privacy, consent, bias, and fairness. Ensuring individuals' privacy rights are respected and obtaining proper consent for data collection and usage is paramount. Additionally, efforts must be made to identify and mitigate biases in algorithms and data sources, promoting fairness and inclusivity. Transparency and accountability in data handling are essential, fostering trust between organizations and their stakeholders. Upholding high ethical standards in data analytics is not only a legal obligation but also a moral imperative that safeguards the rights and well-being of individuals and communities.
Data Analytics plays a pivotal role across industries, enabling informed decision-making and driving innovation. Its applications span from enhancing business strategies to revolutionizing healthcare and beyond. The potential impact on future industries and society is immense, promising advancements in efficiency, accuracy, and overall progress. However, it is imperative to emphasize responsible data analytics practices, ensuring ethical considerations, privacy, and unbiased insights. As we move forward, a collective commitment to these principles will shape a more ethical and equitable future in the realm of data analytics.