Overcoming Challenges in Data Quality for Accurate Analytics
Explore strategies for overcoming data quality challenges to ensure precise and reliable analytics. Learn how to enhance data accuracy for better business insights.
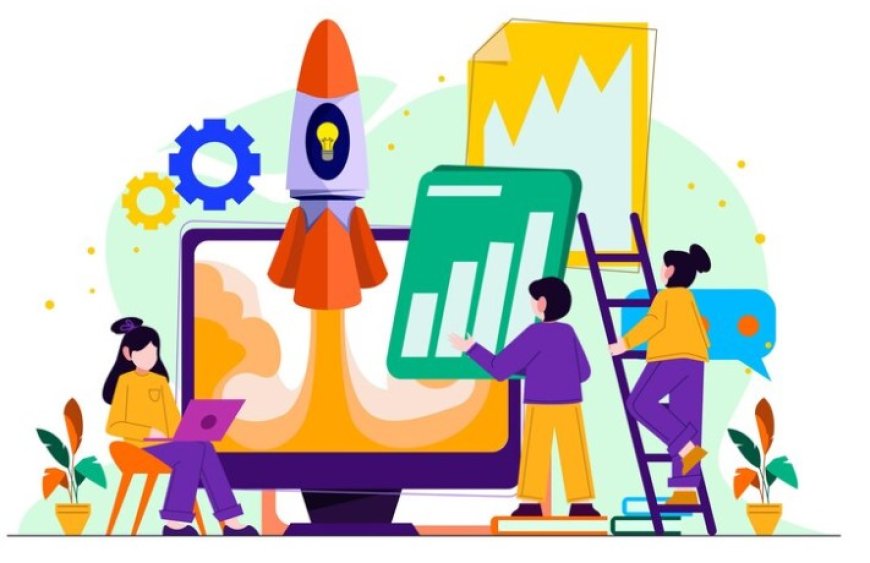
In today's data-driven world, businesses rely heavily on analytics to make informed decisions and gain a competitive edge. However, the success of any analytics initiative hinges on the quality of the data being analyzed. Data quality issues can often lead to inaccurate insights, misinformed decisions, and missed opportunities. In this guide, we will delve into the critical topic of overcoming challenges in data quality to ensure the accuracy and reliability of your analytics endeavors. We will explore strategies, best practices, and tools that empower organizations to address data quality issues effectively, ultimately unlocking the full potential of their data for better decision-making and improved business outcomes.
Understanding Data Quality Challenges
Data quality is the cornerstone of effective data analytics, determining the reliability and trustworthiness of insights extracted from datasets. It refers to the degree to which data is accurate, complete, consistent, timely, and relevant for its intended use. Inaccurate or poor-quality data can lead to misguided conclusions and flawed decision-making, rendering the entire analytical process futile.
Common Challenges Affecting Data Quality:
Incomplete data poses a significant hurdle in achieving accurate analytics. This challenge comprises two primary aspects: missing values and inaccurate data entries. Missing values can distort analysis results, while inaccuracies can stem from human errors during data entry, making it crucial to address both issues.
Inconsistent data presents another obstacle, characterized by data format discrepancies and conflicting information. These inconsistencies can lead to incorrect conclusions and hinder the ability to generate reliable insights.
Data redundancy, although seemingly harmless, can inflate data volumes and complicate analytics efforts. Identifying and eliminating redundant data is essential for maintaining data quality.
Data privacy and security concerns are paramount in the age of data breaches and regulations. Ensuring the protection of sensitive data is a critical aspect of data quality, requiring robust safeguards and compliance with relevant privacy laws.
Addressing these common data quality challenges is essential to ensure that data analytics efforts yield accurate and reliable insights. Organizations must adopt robust strategies to mitigate these issues and maintain high standards of data quality for successful analytics outcomes.
Implications of Poor Data Quality
Using low-quality data for analytics can lead to a series of detrimental outcomes that undermine the integrity of decision-making processes. These consequences not only hinder progress but can also have lasting repercussions on an organization's overall performance.
-
Misleading Insights: The foundation of data analytics relies on accurate and reliable data. Poor data quality introduces inaccuracies, leading to misleading insights. Decision-makers may base their strategies on flawed assumptions, resulting in actions that are misaligned with actual trends and patterns in the data.
-
Flawed Decision-Making: Inaccurate data can significantly impact the quality of decisions made by organizations. Decisions made based on faulty data can be suboptimal, leading to missed opportunities, ineffective resource allocation, and misguided strategies. Inconsistencies in data can cause leaders to overlook crucial aspects, leading to poor choices.
-
Wasted Resources and Time: Utilizing low-quality data requires efforts to rectify errors, wasting valuable time and resources that could have been better spent on productive tasks. Cleaning and correcting data consumes manpower and diverts attention from more valuable analytical tasks that contribute to growth and innovation.
-
Loss of Trust in Data-Driven Approaches: The cornerstone of data-driven approaches is trust in the data itself. When data quality falters, trust erodes. Decision-makers and stakeholders become hesitant to rely on data for informed choices, reverting to gut feelings or anecdotal evidence. This lack of confidence can hinder the adoption of data-driven practices within an organization.
Strategies to Overcome Data Quality Challenges
Data Cleansing and Preprocessing: To address incomplete or inaccurate data, organizations should implement robust data cleansing and preprocessing techniques. This involves identifying and rectifying missing values, handling outliers, and standardizing data formats. These steps help ensure that the data is accurate and complete before it's used for analytics, reducing the risk of making decisions based on flawed information.
Data Validation and Verification: Validating and verifying data is crucial to maintain data consistency. This process involves cross-checking data for inconsistencies, duplicates, and errors. By implementing validation rules and verification procedures, organizations can catch discrepancies early and prevent them from negatively impacting analytical outcomes.
Data Governance and Quality Frameworks: Establishing comprehensive data governance policies and quality frameworks is essential. This includes defining data ownership, setting data quality standards, and creating processes for data stewardship. Effective data governance ensures that data quality is upheld throughout its lifecycle, from collection to analysis.
Data Privacy Measures: Ensuring data privacy is paramount, especially in the era of increasing data regulations. Organizations must implement data privacy measures to protect sensitive information. This involves anonymization, encryption, access controls, and compliance with relevant data protection laws. Proper data privacy measures not only enhance data quality but also safeguard against legal and ethical issues.
By employing these strategies, organizations can enhance data quality, leading to more accurate and reliable analytics outcomes, ultimately supporting better decision-making and business success.
Technology Solutions
In the realm of data quality improvement, technology plays a pivotal role in addressing challenges and ensuring the accuracy of analytics. There are several key technological solutions that organizations can leverage:
Data Quality Tools: Specialized data quality tools and software are available to help automate and streamline data cleansing, validation, and enrichment processes. These tools often include features for identifying and rectifying issues such as missing values, duplicates, and inconsistencies.
Machine Learning for Data Quality Enhancement: Machine learning algorithms can be employed to detect patterns and anomalies in data, aiding in the identification of data quality issues. ML models can learn from historical data to predict potential data quality problems and suggest corrective actions.
Data Monitoring and Auditing Tools: Continuous data monitoring tools provide real-time insights into data quality, alerting organizations to issues as they arise. These tools enable proactive data quality management and can trigger automated responses to maintain data accuracy.
By embracing these technology-driven solutions, organizations can strengthen their data quality efforts, ultimately leading to more accurate and reliable analytics outcomes.
Future Trends in Data Quality Management
As the field of data analytics continues to evolve, so does the realm of data quality management. Addressing data quality challenges is no longer solely reliant on traditional methods; instead, emerging trends are reshaping how organizations approach and maintain data quality.
AI-Powered Data Quality Tools
One of the most promising developments in data quality management is the integration of artificial intelligence (AI) technologies. AI-powered data quality tools are revolutionizing the way organizations handle data discrepancies and anomalies. These tools utilize machine learning algorithms to identify patterns and anomalies in data sets, helping to detect errors, inconsistencies, and outliers that may be missed by conventional methods. By harnessing the power of AI, organizations can proactively identify and rectify data quality issues, ultimately improving the accuracy and reliability of their analytics processes.
Automation of Data Quality Processes
Automation is a pivotal trend that is transforming various aspects of business operations, and data quality management is no exception. Automation streamlines data validation, cleansing, and enrichment processes, reducing manual intervention and human errors. Organizations are increasingly adopting automated workflows that continuously monitor data quality in real-time. This not only ensures that data remains accurate and up-to-date but also frees up data professionals to focus on more strategic tasks, thus enhancing overall efficiency.
Proactive Data Quality Monitoring
Rather than addressing data quality issues after they've already caused problems, the trend of proactive data quality monitoring is gaining traction. This approach involves setting up robust monitoring systems that constantly assess data quality metrics and predefined thresholds. When a data quality issue crosses these thresholds, alerts are generated, enabling data professionals to intervene before the problem cascades into more significant challenges. Proactive monitoring empowers organizations to maintain high data quality levels and promptly address issues before they impact critical decision-making processes.
Data quality is the bedrock upon which accurate and insightful analytics stand. Without high-quality data, the foundation of decision-making becomes shaky, leading to misguided outcomes and missed opportunities. A proactive and continuous commitment to data quality management is paramount, as it safeguards against the pitfalls of inaccurate information. By implementing the strategies and best practices highlighted here, organizations can ensure that their data remains reliable and trustworthy, enabling them to extract meaningful insights and drive success in their analytical endeavors.