Data Science vs Data Analytics: The Differences
Explore the distinctions between Data Science and Data Analytics. Discover key differences in roles, skills, and applications. Make informed career choices with this insightful guide.
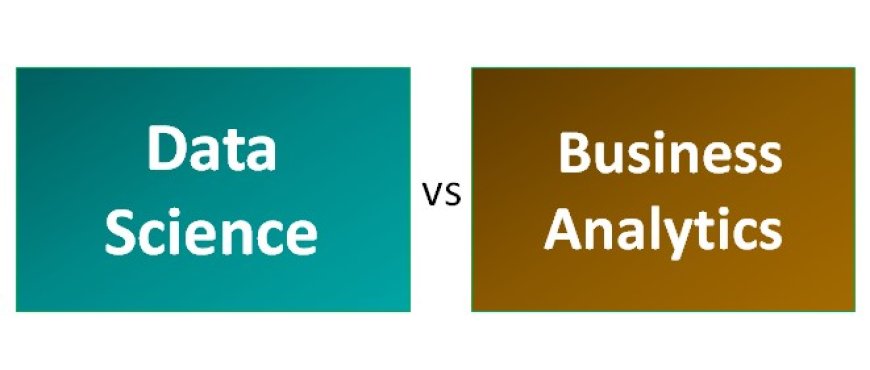
In this discussion of Data Science vs. Data Analytics, we begin with an introduction that provides clear definitions for both fields. Data Science is the comprehensive discipline that encompasses data collection, analysis, and interpretation to derive actionable insights. Data Analytics, on the other hand, focuses on analyzing data to provide insights and support decision-making. We emphasize the importance of distinguishing between these two domains to ensure that organizations can harness their unique strengths effectively in the data-driven landscape.
Data Science
Data science is a multidisciplinary field that involves extracting knowledge and insights from large and complex datasets using various techniques, including machine learning, statistical analysis, and data visualization. Its primary purpose is to uncover hidden patterns, solve complex problems, and support informed decision-making by providing actionable insights based on data.
Skills and Expertise Required:
Professionals in data science need a diverse skill set, including expertise in programming (Python, R), statistical analysis, data manipulation, and domain knowledge. They also require a strong understanding of machine learning algorithms, data preprocessing, and data engineering to effectively work with vast and messy datasets.
Role in Business Decision-Making:
Data scientists play a crucial role in helping businesses make data-driven decisions. They build predictive models, conduct experiments, and provide recommendations that inform strategic choices, optimize processes, and identify opportunities for growth and efficiency improvement.
Examples of Data Science Applications:
Data science has a wide range of applications across industries, including:
-
Predictive maintenance in manufacturing
-
Recommender systems in e-commerce
-
Fraud detection in finance
-
Healthcare diagnostics and personalized medicine
-
Natural language processing for sentiment analysis in social media.
-
Data science's versatility makes it a valuable asset for businesses seeking to harness the power of data for competitive advantage.
Data Analytics
Data analytics involves the examination and interpretation of data to uncover meaningful insights, patterns, and trends. Its primary purpose is to provide actionable information that aids in informed decision-making. Data analysts use statistical and analytical techniques to transform data into valuable knowledge for organizations.
Skills and Expertise Required:
Data analysts need proficiency in data manipulation, statistical analysis, and data visualization tools such as Excel, Python, or R. Strong analytical thinking and problem-solving skills are crucial, along with domain knowledge relevant to the industry they work in.
Role in Business Decision-Making:
Data analytics plays a pivotal role in helping businesses make data-driven decisions. It assists in identifying opportunities for optimization, cost reduction, and revenue growth. By analyzing past and present data, organizations can make informed choices that improve operations and strategy.
Examples of Data Analytics Applications:
Data analytics finds applications in various domains, including:
-
Sales and marketing optimization through customer segmentation and campaign analysis.
-
Fraud detection in financial transactions by identifying unusual patterns.
-
Healthcare, where it's used for patient outcome predictions and resource allocation.
-
Supply chain management to improve inventory levels and distribution efficiency.
-
Social media analysis for sentiment tracking and customer engagement assessment.
Key Differences
Data Science and Data Analytics differ in their focus, scope, skills, tools, and ultimate goals.
Focus and Scope:
Data Science delves into the entire data lifecycle, encompassing data collection, cleaning, and advanced analysis techniques like machine learning and predictive modeling. It deals with complex, unstructured data to extract deep insights for strategic decision-making.
Data Analytics primarily centers on examining data sets to identify trends, patterns, and draw conclusions. It typically revolves around structured data, focusing on generating actionable insights to address specific business questions.
Skills and Tools:
Data Scientists possess a broader skill set, including programming (Python, R), statistical analysis, machine learning, and expertise in databases (SQL, NoSQL). They are adept at handling big data frameworks like Hadoop and Spark.
Data Analysts are proficient in tools like Excel, Tableau, and programming languages like SQL. They excel in data visualization, reporting, and possess a solid understanding of data querying and transformation.
Goal and Outcome:
Data Science aims at creating predictive models, uncovering hidden patterns, and providing a deeper understanding of data to drive innovation and strategic decision-making. It often involves developing algorithms and implementing machine learning solutions.
Data Analytics focuses on generating insights to address specific business queries, aiding in operational improvements, and optimizing processes. Its outcomes typically result in actionable recommendations for immediate business impact.
Overlaps and Intersections
Commonalities between Data Science and Data Analytics
While Data Science and Data Analytics have distinct focuses, they share commonalities in their methodologies. Both rely on data collection, cleansing, and exploration to extract meaningful insights. Additionally, statistical techniques and programming languages like Python and R are essential in both disciplines. This overlap forms a foundation for collaboration and knowledge transfer between Data Scientists and Data Analysts.
Collaborative Efforts in Real-world Applications
In practice, Data Scientists and Data Analysts often collaborate to solve complex problems. Data Analysts provide valuable preliminary insights by generating reports and visualizations, which Data Scientists can then leverage to develop advanced predictive models or machine learning algorithms. This synergy enhances the overall data-driven decision-making process, illustrating the interdependence of these fields in real-world applications.
When to Use Data Science vs Data Analytics
In scenarios where the goal is to unearth complex insights, develop predictive models, or tackle intricate problems, Data Science is the preferred choice. For example, when building recommendation systems for e-commerce platforms or optimizing supply chain logistics, Data Science techniques like machine learning and deep learning shine by handling large, unstructured data and offering predictive capabilities.
Data Analytics is most effective in scenarios where the primary objective is to explore historical data, generate descriptive reports, or make data-driven decisions based on readily available information. For instance, in marketing campaigns, analyzing past performance metrics, and creating actionable insights for immediate implementation are typical tasks where Data Analytics excels.
Career Paths and Job Roles
Data Scientist Roles and Responsibilities:
Data scientists are responsible for collecting, cleaning, and analyzing large datasets to extract meaningful insights. They develop machine learning models, algorithms, and statistical techniques to solve complex problems. They work closely with stakeholders to translate data-driven findings into actionable business strategies. Data scientists are often involved in research and innovation, pushing the boundaries of data analysis.
Data Analyst Roles and Responsibilities:
Data analysts focus on examining data to provide valuable information that supports decision-making within an organization. They typically work with structured data, perform data cleaning, and conduct descriptive and diagnostic analyses. Data analysts generate reports, dashboards, and visualizations to communicate their findings effectively. Their role is crucial in monitoring key performance indicators and helping teams make data-informed decisions.
Skillset and Qualifications for Each Role:
-
Data Scientist: Data scientists require strong programming skills (e.g., Python, R), expertise in machine learning, statistical modeling, and data visualization. They often hold advanced degrees (e.g., master's or Ph.D.) in fields like data science, computer science, or statistics.
-
Data Analyst: Data analysts should be proficient in data manipulation tools (e.g., Excel, SQL), data visualization (e.g., Tableau, Power BI), and have a solid understanding of statistics. They typically hold bachelor's degrees in fields like business, economics, or a related discipline.
Overall, data scientists tend to have a more advanced skillset and tackle more complex problems, while data analysts focus on providing insights from existing data to support day-to-day operations. Both roles play critical roles in leveraging data for organizational success.
Understanding the distinctions between Data Science and Data Analytics is essential in the data-driven era. Data Science encompasses a broader range of activities, involving complex modeling and prediction, while Data Analytics focuses on extracting insights from data to inform decision-making. The choice between the two depends on specific objectives, with both playing crucial roles in harnessing the power of data to drive innovation and success in various industries.