What is Data Science?
Discover what data science is and its significance in today's digital world. Learn about data analysis, machine learning, and more in this comprehensive guide.
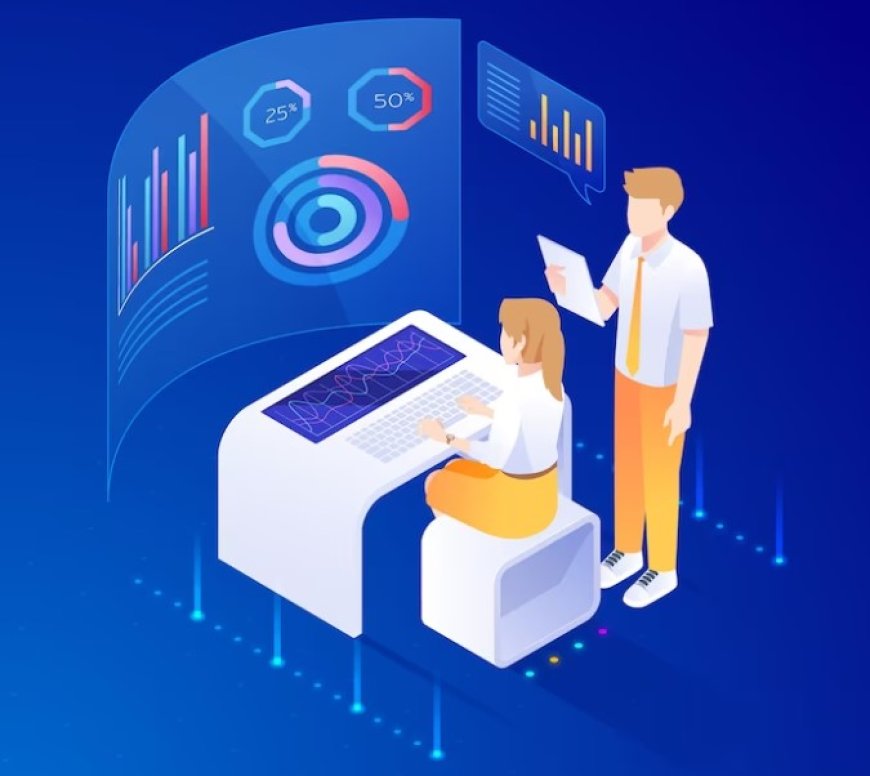
Data Science is a multidisciplinary field that combines statistical analysis, data exploration, machine learning, and domain expertise to extract valuable insights and knowledge from data. It involves collecting, cleaning, and transforming raw data into meaningful information, which can then be used to make data driven decisions, solve complex problems, and drive innovation. Data Science plays a pivotal role in various industries, from healthcare and finance to marketing and technology, empowering organizations to harness the power of data and gain a competitive edge in the modern data driven world.
What Is Data Science?
Data Science is an interdisciplinary field that employs scientific methods, algorithms, and processes to extract valuable insights and knowledge from data. It involves collecting, cleaning, and analyzing large datasets using statistical and machine learning techniques. Data Science enables organizations to make data driven decisions, predict trends, and gain a competitive edge in various industries. Its applications range from solving complex problems to developing innovative solutions across different domains, making it a critical discipline in the era of big data and digital transformation.
The historical background of Data Science can be traced back to the early 20th century, when statisticians like Ronald Fisher and Karl Pearson laid the foundation for statistical methods to analyze data. The term "Data Science" was first used in 1960 when computer scientist Peter Naur discussed its importance in scientific data handling. However, it wasn't until the late 1990s and early 2000s that Data Science as a discipline started to emerge, driven by advancements in computing power, data storage, and the internet. The exponential growth of data and the need to extract valuable insights from it led to the formalization of Data Science as an interdisciplinary field, combining elements of statistics, computer science, and domain expertise to revolutionize decision making and problem solving in the digital age.
Importance and relevance of Data Science in the modern era
-
Extracts valuable insights from large and complex datasets.
-
Enables data driven decision making for businesses and organizations.
-
Improves efficiency and productivity by optimizing processes.
-
Facilitates predictive analytics for future trends and patterns.
-
Enhances customer experience through personalized services and recommendations.
-
Drives innovation and fosters growth in various industries.
-
Addresses real world challenges through data driven problem solving.
-
Empowers evidence based research and scientific discoveries.
-
Supports healthcare advancements, disease detection, and personalized medicine.
-
Contributes to smart cities and sustainable development initiatives.
Data Acquisition and Preprocessing
Data acquisition and preprocessing are crucial steps in the data science workflow that lay the foundation for meaningful analysis and insights. Let's explore each of these steps in detail:
-
Data Acquisition: Data acquisition involves gathering relevant data from various sources. This step includes identifying the data requirements for the analysis and obtaining the data from databases, APIs, web scraping, sensors, social media, or other relevant sources. Ensuring data accuracy, completeness, and relevance is essential in the data acquisition phase to set the groundwork for a successful analysis.
-
Data Preprocessing: Data preprocessing encompasses a series of data cleaning and transformation techniques to ensure the data is in a suitable format for analysis. This step involves identifying and handling missing data, removing duplicates, resolving inconsistencies, and addressing outliers. Data normalization, scaling, and feature engineering may also be performed to standardize the data and improve the performance of machine learning algorithms.
The data acquisition and preprocessing phases are critical in data science, as the quality and cleanliness of the data directly impact the accuracy and reliability of the analysis and subsequent decision making. Properly acquiring and preprocessing data sets the stage for meaningful data exploration, modeling, and interpretation, ultimately leading to valuable insights and actionable outcomes.
Data Exploration and Visualization
Data exploration and visualization play a vital role in understanding the characteristics and patterns within the data. In this phase, data analysts use various techniques to gain insights, identify trends, correlations, and outliers. Data visualization tools and methods, such as charts, graphs, and heatmaps, are employed to present the findings in a visually appealing and easily interpretable manner. This process helps data scientists and stakeholders to make informed decisions, validate assumptions, and uncover hidden relationships, contributing to the overall success of data driven projects.
Statistical Analysis and Machine Learning
Statistical analysis and machine learning are essential components of data science that enable data scientists to extract valuable knowledge and predictions from data. Statistical analysis involves using mathematical and probability based techniques to understand data distributions, test hypotheses, and draw inferences. Machine learning, on the other hand, employs algorithms to automatically learn from data and make predictions or decisions without explicit programming. Together, these powerful methodologies form the backbone of data driven decision making, enabling businesses to uncover insights, develop predictive models, and drive innovation across various domains.
Big Data and Data Engineering
Big Data and Data Engineering are critical aspects of Data Science that deal with the management and processing of large volumes of data. Big Data refers to datasets that are too large and complex for traditional data processing techniques to handle efficiently. Data Engineering involves designing and building data pipelines and systems to collect, store, and process data at scale. In Data Science, the ability to handle Big Data and implement effective Data Engineering solutions is crucial for performing advanced analytics, running machine learning algorithms, and deriving valuable insights from massive datasets. These capabilities empower organizations to leverage the full potential of data and drive data driven strategies in the modern era of data intensive applications.
Data Modeling and Evaluation
Data Modeling and Evaluation are crucial stages in the data science process that involve building predictive models and assessing their performance.
-
Data Modeling: Data modeling is the process of creating mathematical representations or algorithms that capture the relationships and patterns within the data. This stage includes selecting appropriate machine learning algorithms, feature selection, and training the models on the dataset.
-
Model Evaluation: Model evaluation involves assessing the performance of the trained models using various metrics, such as accuracy, precision, recall, and F1 score. Cross Validation techniques are often used to ensure the models' generalizability and robustness to unseen data.
The data modeling and evaluation stages are essential in data science as they enable data scientists to identify the best performing models, refine them if necessary, and ultimately choose the most suitable model to make accurate predictions and derive valuable insights from the data. Proper model evaluation ensures that the data driven decisions made based on the models are reliable and effective.
Predictive Analytics and Business Applications
-
Predictive analytics uses historical data and statistical algorithms to make predictions about future outcomes.
-
It helps businesses identify patterns and trends to forecast customer behavior and demand.
-
Predictive models aid in optimizing marketing campaigns, targeting specific customer segments, and improving conversion rates.
-
Businesses can use predictive analytics to optimize inventory management and supply chain operations.
-
Predictive models can aid in fraud detection and risk assessment in financial industries.
-
Sentiment analysis and customer churn prediction help enhance customer satisfaction and retention.
-
Predictive maintenance in manufacturing improves asset performance and reduces downtime.
-
Data Driven decision making using predictive analytics drives overall business growth and profitability.
Data Ethics and Privacy
Data Ethics and Privacy are essential considerations in the field of data science and analytics. Data ethics involves ensuring that data collection, analysis, and usage adhere to ethical principles, respect individual rights, and avoid harm or discrimination. It involves obtaining informed consent, protecting sensitive information, and being transparent about data practices. Data privacy, on the other hand, involves safeguarding personal information from unauthorized access, use, or disclosure. Addressing data ethics and privacy concerns is crucial to build trust with users, maintain data integrity, and uphold ethical standards in the responsible use of data for the benefit of individuals and society as a whole.
Data Science in RealWorld Scenarios
-
Healthcare: Data Science is used to analyze patient records, diagnose diseases, and predict health outcomes, leading to personalized treatments and improved patient care.
-
Finance: Data Science is employed for fraud detection, credit risk assessment, and algorithmic trading, enhancing financial security and optimizing investment decisions.
-
Ecommerce: Data Science enables personalized product recommendations, demand forecasting, and pricing optimization, improving customer experiences and boosting sales.
-
Marketing: Data Science drives targeted marketing campaigns, customer segmentation, and sentiment analysis on social media, leading to more effective marketing strategies.
-
Transportation: Data Science is used in traffic management, route optimization, and autonomous vehicles, enhancing transportation efficiency and safety.
-
Environmental Science: Data Science aids in analyzing environmental data for climate change research, pollution monitoring, and sustainable development initiatives.
-
Manufacturing: Data Science optimizes production processes, predictive maintenance, and quality control, improving productivity and reducing downtime.
-
Education: Data Science facilitates adaptive learning, educational analytics, and student performance prediction, enhancing personalized education experiences.
-
Sports Analytics: Data Science is applied in sports to analyze player performance, game strategies, and injury prevention, enhancing team performance and player development.
-
Social Sciences: Data Science is used in social research, sentiment analysis on public opinions, and studying social trends, providing insights into human behavior and societal patterns.
Future of Data Science
The future of Data Science is poised to be even more transformative and impactful. As technology advances, data generation will continue to skyrocket, creating new challenges and opportunities for data scientists. Data Science will evolve to handle vast and diverse datasets, leveraging advancements in machine learning, artificial intelligence, and data analytics. With the integration of Data Science into various industries, it will drive innovation, optimize processes, and foster evidence based decision making. As data ethics and privacy gain prominence, responsible Data Science practices will ensure the ethical use of data. The future of Data Science promises a data driven world where its applications will shape industries, enhance our daily lives, and create a more informed and sustainable future.
Data Science has emerged as a powerful discipline that empowers businesses and industries to harness the potential of data for informed decision making, predictive analytics, and innovative solutions. With its ability to extract valuable insights from vast datasets and predict future trends, Data Science plays a pivotal role in shaping the future of various sectors, including healthcare, finance, marketing, and more. As we continue to advance in technology and data capabilities, responsible data ethics and privacy will be paramount in ensuring the ethical use of data for the greater good. The future of Data Science holds immense promise, paving the way for a data driven world that drives progress, fosters innovation, and addresses complex challenges for a better tomorrow.