Data Analytics in Finance: Enhancing Risk Management
Explore how data analytics in finance enhances risk management. Learn how data-driven insights and advanced analytics are transforming the financial industry.
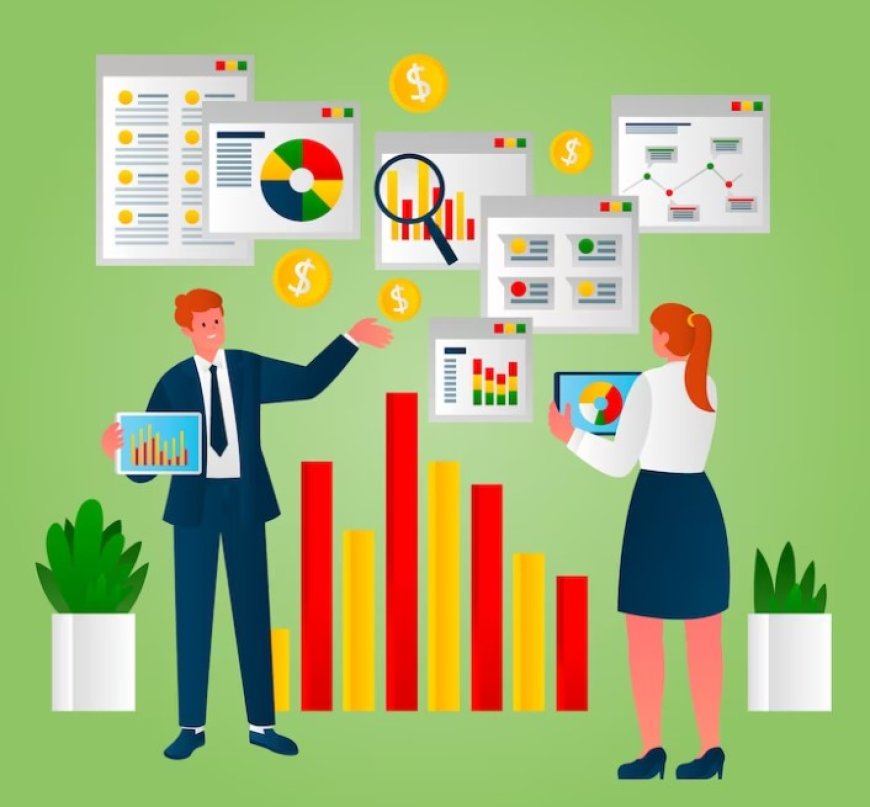
In the introduction section of this document, we first define the concept of Data Analytics in Finance, highlighting its significance within the financial sector. We then emphasize the crucial role of Risk Management in the finance industry, underscoring the need for effective strategies to mitigate financial risks. Finally, setting the stage for a comprehensive exploration of how Data Analytics can enhance Risk Management in the world of finance.
Data Collection and Acquisition
Data collection and acquisition are foundational steps in the process of enhancing risk management through data analytics in finance. In this phase, financial institutions gather relevant data from various sources, ensuring its accuracy and completeness. This involves accessing a wide range of financial information, including market data, transaction records, customer profiles, and economic indicators.
Furthermore, maintaining data quality and integrity is paramount to ensure the effectiveness of subsequent analytical processes. Financial organizations implement rigorous data preprocessing techniques, such as cleaning, transformation, and validation, to prepare the data for analysis. This phase serves as the critical building block upon which advanced analytics and risk assessment will be based, ultimately enabling more informed decision-making and risk mitigation in the financial sector.
Data Analysis Tools and Techniques
In the realm of data analytics in finance, various tools and techniques play a pivotal role in extracting valuable insights and enhancing risk management practices.
Descriptive Analytics:
Descriptive analytics involves the exploration and summarization of historical financial data. It leverages techniques like data visualization and key financial metrics to provide a comprehensive overview of past performance. By examining trends and patterns, financial professionals can gain a clear understanding of their organization's historical financial behavior.
Predictive Analytics:
Predictive analytics goes beyond historical data, utilizing statistical models and machine learning algorithms to forecast future financial outcomes. This empowers finance professionals to anticipate market trends, customer behavior, and potential risks. By identifying early warning signs, organizations can make informed decisions to mitigate future financial challenges.
Prescriptive Analytics:
Prescriptive analytics takes data analysis a step further by offering actionable recommendations. It uses optimization methods and scenario analysis to prescribe specific strategies for risk mitigation and financial decision-making. This enables finance professionals to proactively address potential issues and optimize their financial strategies to achieve desired outcomes.
Risk Assessment and Measurement
In this section, we delve into the critical aspects of risk assessment and measurement in the context of data analytics in finance. We explore the various types of financial risks that organizations face, including market risk, credit risk, and operational risk. Furthermore, we discuss the key risk metrics used to quantify and evaluate these risks, such as Value at Risk (VaR), Credit Risk Models, and stress testing methodologies. Additionally, we examine the importance of stress testing and sensitivity analysis as essential tools for assessing and understanding the potential impact of adverse financial scenarios on an organization's stability and resilience. This section serves as a foundational component in enhancing risk management through data analytics in the finance sector.
Challenges and Considerations
Data Privacy and Security: Ensuring the privacy and security of financial data is paramount. With sensitive financial information at stake, robust data encryption, access controls, and compliance with privacy regulations like GDPR and HIPAA are essential. Organizations must also be vigilant against data breaches and cyber threats, implementing comprehensive security protocols to safeguard their data assets.
Regulatory Compliance: Finance is heavily regulated, and data analytics initiatives must align with industry-specific regulations such as Basel III, Dodd-Frank, and Sarbanes-Oxley Act. Compliance requires meticulous record-keeping, reporting, and adherence to legal frameworks, which can be complex and subject to change.
Skill and Resource Requirements: Effective data analytics in finance demands a skilled workforce and substantial resources. Organizations must invest in training personnel in data analysis, machine learning, and financial modeling. Additionally, the infrastructure for data storage, processing, and analytics tools must be adequately provisioned to handle the volume and complexity of financial data. Balancing these resource investments is a critical consideration for successful implementation.
Data Governance and Quality: Maintaining data quality and governance is a persistent challenge. Inaccurate or incomplete data can lead to flawed analyses and erroneous risk assessments. Establishing data governance frameworks, data lineage tracking, and data quality checks are crucial to mitigate these risks.
Interoperability and Data Integration: Many financial institutions have disparate data sources and systems. Ensuring seamless integration and interoperability among these systems is a challenge. Organizations must invest in robust data integration solutions and data warehouses to consolidate and harmonize data for effective analysis.
Ethical Considerations: As data analytics becomes more powerful, ethical considerations arise. Ensuring that analytics practices are ethically sound and do not lead to biased or discriminatory outcomes is essential. Ethical guidelines and principles must be integrated into the analytics process.
Cost Management: Data analytics can be resource-intensive, both in terms of infrastructure and personnel. Managing costs effectively while achieving desired outcomes is a consideration. This includes optimizing cloud resources, evaluating the cost-benefit of different analytics tools, and maintaining a balance between cost efficiency and analytical capabilities.
Change Management: Implementing data analytics practices often requires a cultural shift within an organization. Change management is crucial to gain buy-in from stakeholders, foster collaboration among departments, and ensure that the organization as a whole embraces the new approach.
Monitoring and Adaptation: Continuous monitoring of data analytics processes and risk models is necessary. Financial markets and regulations evolve, and analytics models must adapt accordingly. Organizations should have mechanisms in place for ongoing model validation, monitoring, and adaptation.
In these challenges and considerations, financial institutions can harness the power of data analytics to enhance risk management and make more informed decisions in an ever-changing financial landscape.
Future Trends
In the ever-evolving landscape of data analytics in finance, it is crucial to anticipate future trends that will shape the industry. Two key aspects to watch are emerging technologies and evolving risk management strategies.
Emerging Technologies: The financial sector is witnessing a rapid integration of cutting-edge technologies such as artificial intelligence, blockchain, and quantum computing. These technologies hold the potential to revolutionize data analytics by enabling more sophisticated data processing, improved predictive modeling, and enhanced security measures. Staying abreast of these emerging technologies and their applications will be essential for staying competitive in risk management.
Evolving Risk Management Strategies: As financial markets become more complex and interconnected, risk management strategies must adapt. This involves a shift towards more dynamic, real-time risk assessment and mitigation. Additionally, holistic approaches that consider not only financial but also environmental, social, and governance (ESG) factors are gaining prominence. Understanding and implementing these evolving strategies will be paramount for effective risk management in the finance sector.
We've discussed the importance of data quality, various analytical tools, risk assessment techniques, and provided real-world case studies illustrating its impact. However, it's crucial to acknowledge the challenges of data privacy, regulatory compliance, and resource requirements. Looking forward, as emerging technologies continue to evolve, data analytics is poised to play an even more significant role in shaping the future of risk management in the finance sector. In light of this, it's recommended that financial institutions continue to invest in data analytics capabilities and stay abreast of industry trends to remain competitive and resilient in an ever-changing landscape.