The Growing Scope of Machine Learning in Technology
Learn how machine learning is transforming fields like healthcare, finance, retail, and more, driving innovation and automation.
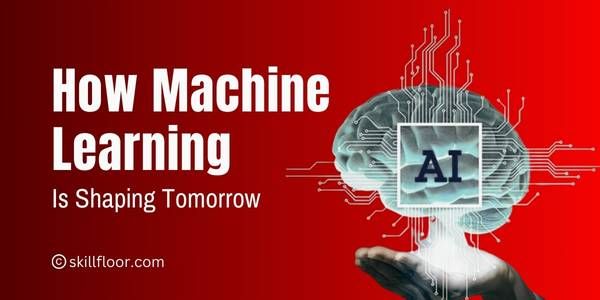
Everyone now uses machine learning in their daily lives, which simplifies chores and personalizes encounters. It enables features like voice assistants and Netflix recommendations, which facilitate work, shopping, and leisure. It's influencing sectors and improving entertainment, from identifying financial fraud to making life easier for everyone.
The term "machine learning" has evolved into much more than that. Now an essential component of technology, its capacity to analyze data and generate astute predictions is revolutionizing businesses. Solutions in healthcare, finance, and other fields are being shaped by the scope of machine learning, which is growing thanks to practical applications and innovative thinking.
Although machine learning offers many advantages, there are drawbacks as well, such as issues with data privacy and equity. Developing trust requires being open and honest about how it operates and making sure it is utilized sensibly. By taking care of these issues, we may realize our full potential and provide just, moral, and advantageous solutions for everybody.
What Is Machine Learning?
Machine learning is the process of teaching computers to make judgments on their own without continual human guidance by using data. Numerous industries employ it, such as assisting physicians in early illness detection or enhancing consumer experiences in retail, demonstrating the versatility and strength of this technology.
Consider how a music app can identify your favorite tunes or how internet retailers may recommend items you might find interesting. That's machine learning in action—making life easier and tailoring experiences. Modern technology has made it a necessary component, influencing our interactions with the outside world in more intelligent and natural ways.
Key Differences Between ML, AI, and Deep Learning
Aspect |
Artificial Intelligence (AI) |
Machine Learning (ML) |
Deep Learning (DL) |
Definition |
Broad field aiming to create machines that mimic human intelligence. |
Subset of Artificial Intelligence, focused on enabling machines to learn from data. |
Subset of ML using complex neural networks to analyze data deeply. |
Scope |
Encompasses reasoning, problem-solving, learning, and decision-making. |
Focused on data-driven learning and improving predictions or decisions. |
Specializes in recognizing patterns in large datasets with high accuracy. |
Examples |
Chatbots, robots, and virtual assistants. |
Fraud detection, personalized recommendations. |
Facial recognition, voice assistants like Siri. |
Complexity |
Combines various approaches, including ML and DL. |
Relies on simpler algorithms like regression or decision trees. |
Uses multi-layered neural networks for high-level tasks. |
Data Dependency |
Can function with limited or structured data. |
Requires structured or semi-structured data to learn. |
Needs massive amounts of unstructured data to perform well. |
Use Cases |
General-purpose intelligence systems. |
Targeted tasks like spam filtering or credit scoring. |
Advanced tasks like image or speech recognition. |
Types of Machine Learning
The three primary categories of machine learning—supervised learning, unsupervised learning, and reinforcement learning—are based on how computers learn from data. Every kind is utilized for a particular purpose and has a distinct methodology. Each of them will be examined in further detail.
1. Supervised Learning
Supervised learning is like teaching a child with examples. In this method, the system is trained using labeled data, which means the input data comes with the correct answers (or labels). The algorithm learns by comparing its predictions with the correct output and adjusts itself to improve accuracy.
How it works:
There are input-output pairs available for the system. If you want the model to recognize fruits, for example, you might feed it pictures of fruits, and the output (label) would indicate if it's an orange, banana, or apple. The algorithm gains the ability to link inputs to the appropriate outputs over time.
2. Unsupervised Learning
Labeled data is not necessary for unsupervised learning to function. Rather, it works with unstructured, raw data and looks for hidden patterns or groups without giving particular directions.
How it works:
The system is given a dataset and instructed to identify any patterns or correlations in it. It may, for instance, put related objects in one category without explaining the significance of those groupings.
3. Reinforcement Learning
A dynamic learning technique called reinforcement learning involves a system interacting with its surroundings and getting feedback in the form of rewards or penalties. It is comparable to using positive reinforcement to train a pet.
How it works:
The system acts in a given environment, monitors the outcomes, and modifies its approach over time to optimize rewards. A robot learning to walk, for example, will experiment with various motions and adjust according to the success of each effort.
The Growing Demand for Machine Learning Across Industries
-
Healthcare: By assisting physicians with early illness detection, diagnosis accuracy, patient outcome prediction, and therapy personalization, machine learning improves healthcare quality and makes it more effective and accessible for people all over the world.
-
Finance: By enabling fraud detection, risk assessment, market trend research, and personalized financial advice, machine learning helps banks and financial institutions provide more intelligent, secure, and safe money management solutions that are suited to each customer's needs.
-
Retail and E-commerce: Retailers create a smooth shopping experience while increasing operational efficiency and consumer pleasure by utilizing machine learning for demand forecasts, inventory optimization, dynamic pricing strategies, and tailored product suggestions.
-
Transportation: Self-driving cars, route optimization programs, and predictive traffic management are all made possible by machine learning, which also lowers traffic and improves road safety while facilitating faster, more convenient, and more efficient transportation for regular people.
-
Education: By providing tailored lesson plans, automated grading, and adaptive learning platforms, machine learning improves education by allowing students to study at their own speed and achieving better results for students of all ages.
-
Entertainment: Globally, streaming services and gaming platforms are transforming the way consumers watch movies, listen to music, and play games by using machine learning to identify user preferences, suggest tailored content, and provide captivating experiences.
Scope of Machine Learning in Technology
Machine learning has the power to completely transform how we use technology, find solutions to issues, and open up new possibilities. The scope of machine learning is vast, benefiting both businesses and people. Let's examine its extent in further detail:
1. Automation of Repetitive Tasks
Data input, sorting, and quality checking are just a few of the time-consuming, repetitive operations that may be automated with machine learning. This boosts output while freeing up human attention for more strategic and creative tasks, demonstrating the wide scope of machine learning in process optimization.
2. Enhanced Personalization
Making user experiences tailored is part of the scope of machine learning. ML customizes services to individual interests, enhancing customer pleasure and engagement and fostering loyalty. Examples of this include making product recommendations in e-commerce and curating playlists on streaming platforms.
The capacity of machine learning to examine historical data and forecast future patterns is one of its most significant features. Companies utilize it to estimate demand, evaluate risks, and analyze consumer behavior, which helps them make better and more informed decisions.
4. Improved Healthcare
Machine learning is improving diagnostic tools, making it possible to diagnose illnesses early, forecast patient outcomes, and customize treatments in the healthcare industry. The enormous scope of machine learning in saving lives and enhancing global health systems is shown by its speedy processing of medical data.
5. Smarter Transportation
Machine learning is essential for effective logistical operations, traffic control systems, and self-driving automobiles. It improves road safety, expedites delivery times, and optimizes routes. How we move and distribute things is constantly being redefined by the scope of machine learning in transportation.
6. Real-Time Problem Solving
Machine learning systems can swiftly adjust to changing surroundings and offer prompt answers, improving security and efficiency in anything from real-time cyber threat identification to financial fraud detection.
Challenges and Limitations of Machine Learning in Technology
1. Data Privacy Concerns
Large datasets, which frequently contain sensitive information, are essential to machine learning. One of the biggest challenges facing machine learning techniques today is maintaining user privacy while guaranteeing ethical data utilization.
2. Algorithm Bias
Results using biased datasets may be unjust or erroneous. To prevent discrimination and encourage equitable results, it is essential to make sure that the scope of machine learning encompasses a variety of objective data.
3. High Resource Requirements
It takes a lot of time, storage, and processing power to train machine learning models. The scope of machine learning is expanding, yet this prevents smaller businesses from successfully integrating it across their operations.
4. Lack of Transparency
In delicate applications, complex machine learning models frequently function as "black boxes," with decision-making processes that are difficult to understand, raising questions about responsibility and trust.
5. Scalability Issues
Machine learning systems' scalability and capacity to effectively handle rising demands are impacted by the difficulty of adapting to expanding datasets and complicated contexts.
6. Dependence on Data Quality
The quality of the training data is crucial to machine learning performance. Incomplete or poor data might result in forecasts that are not trustworthy, which reduces the total usefulness of the data in real-world situations.
Future Trends in Machine Learning for Technological Advancement
Machine learning's future depends on its further integration with edge computing, where models run directly on gadgets like smartphones and Internet of Things sensors. By lowering latency and improving real-time decision-making, this trend makes technology quicker, smarter, and more effective—two essential features for applications like driverless cars and smart homes.
The growth of explainable AI, which makes systems more visible and intelligible, is another significant topic in the Future of Machine Learning. In industries like healthcare and finance, where precision and accountability are crucial for effective solutions, this development fosters trust by clearly outlining the rationale behind choices.
Every aspect of technology is being innovated by machine learning, which continues to influence how we live, work, and solve issues. From enhancing healthcare and education to developing transportation and entertainment, the scope of machine learning is broad. We can guarantee that its expansion serves everyone ethically and responsibly by tackling issues like data privacy and transparency. Real-time problem-solving across sectors, customized experiences, and better solutions are all promised by the scope of machine learning. This technology is essential to creating a more connected and efficient society because of its limitless potential to boost industries and make daily living easier.