Data Analytics in Supply Chain Management: Optimizing Operations
Elevate your supply chain with Data Analytics. Learn to optimize operations, enhance efficiency, and drive strategic decisions.
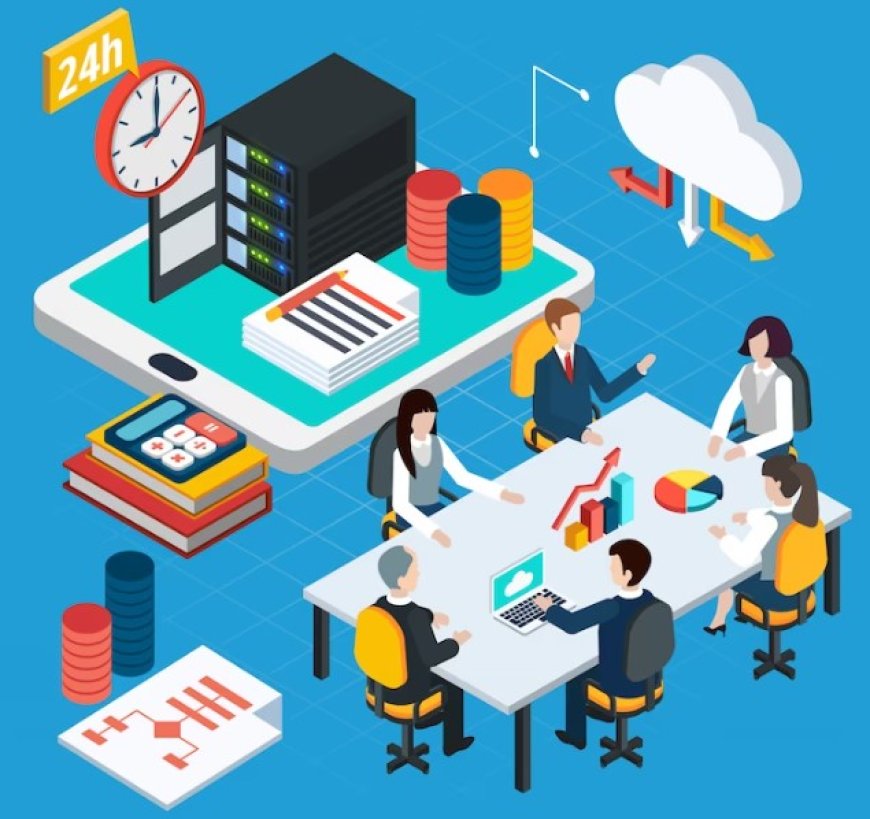
Data Analytics in Supply Chain Management (SCM) is the process of collecting, processing, and analyzing data to enhance the efficiency and effectiveness of supply chain operations. In today's competitive business landscape, optimizing SCM operations is paramount for organizations aiming to streamline processes, reduce costs, and improve customer satisfaction. By harnessing the power of data analytics, businesses can gain valuable insights, make informed decisions, and stay agile in the ever-evolving world of supply chain management.
Key Components of Supply Chain Management
In the realm of data analytics, the various components of Supply Chain Management (SCM) play critical roles in optimizing operations:
-
Planning and Forecasting: Data analytics enables precise demand forecasting by analyzing historical data and market trends. This ensures better inventory management and production planning, reducing overstock or shortages.
-
Sourcing and Procurement: Data analytics helps in supplier evaluation, cost analysis, and risk assessment. It identifies cost-saving opportunities and ensures timely and cost-effective procurement.
-
Manufacturing and Production: Analytics optimizes manufacturing processes by monitoring production metrics, quality control, and equipment maintenance. It aids in predictive maintenance to prevent disruptions.
-
Distribution and Logistics: Analyzing transportation data and route optimization improves distribution efficiency. Real-time tracking enhances visibility into shipments, reducing delays and costs.
-
Customer Service and Returns: Data analytics enhances customer service by analyzing customer feedback and behavior, enabling companies to offer personalized experiences. It also helps streamline returns and warranty processes.
Incorporating data analytics into each SCM component enhances decision-making, efficiency, and overall supply chain performance.
Role of Data Analytics in SCM Optimization
Data analytics plays a pivotal role in optimizing Supply Chain Management (SCM) by providing valuable insights and solutions at different levels of decision-making.
-
Descriptive Analytics: This phase involves the examination of historical data to understand past performance. It helps SCM professionals gain insights into what has happened in the supply chain. Analyzing historical trends, order fulfillment rates, and inventory turnover allows companies to identify areas that need improvement and set benchmarks for future performance.
-
Predictive Analytics: Predictive analytics leverages data to forecast future trends and demand. By analyzing historical data and external factors such as market trends and seasonality, companies can make more accurate predictions about future demand. This aids in inventory management, ensuring that the right products are available at the right time, minimizing stockouts, and reducing excess inventory costs.
-
Prescriptive Analytics: Prescriptive analytics takes data analysis a step further by providing actionable recommendations for real-time decision-making. It considers various scenarios and suggests the best course of action to optimize supply chain operations. This can include decisions related to production scheduling, transportation routes, and inventory allocation, ultimately enhancing overall efficiency.
-
Diagnostic Analytics: Diagnostic analytics focuses on identifying the root causes of issues or inefficiencies within the supply chain. By analyzing data, companies can pinpoint bottlenecks, process inefficiencies, or supply chain disruptions. This knowledge is invaluable for making informed decisions aimed at eliminating these obstacles and improving SCM performance.
Data analytics in SCM optimization encompasses a range of techniques and tools that help companies assess past performance, predict future demand, make real-time decisions, and diagnose problems. By effectively harnessing these analytics capabilities, organizations can streamline their supply chains, reduce costs, and enhance customer satisfaction.
Data Sources in Supply Chain Analytics
In the realm of supply chain analytics, a multitude of data sources converge to provide valuable insights and drive informed decision-making. These sources can be categorized into three main types:
Internal Data: This category encompasses data generated and stored within an organization's own systems. Examples include data from Enterprise Resource Planning (ERP) systems, Customer Relationship Management (CRM) platforms, and internal databases. These internal data sources hold crucial information about inventory levels, order history, customer interactions, and operational processes, offering a rich foundation for supply chain analysis.
External Data: To gain a comprehensive view of the supply chain landscape, organizations tap into external data sources. These may include market trends, economic indicators, competitor data, and even non-traditional sources like social media sentiment analysis. External data enriches the understanding of market dynamics and helps in adapting supply chain strategies to the broader business environment.
IoT Devices and Sensors: In today's digitally connected world, IoT (Internet of Things) devices and sensors play a pivotal role in supply chain analytics. These devices provide real-time data on the status and location of goods in transit, temperature conditions in storage, and the performance of equipment along the supply chain. This real-time data is invaluable for tracking and optimizing operations, enhancing supply chain visibility, and responding swiftly to disruptions.
Leveraging these diverse data sources is essential for a robust supply chain analytics strategy, enabling organizations to enhance efficiency, reduce costs, and better meet customer demands.
Implementing Data Analytics in SCM
Data Collection and Integration
Effective data analytics in supply chain management (SCM) begins with robust data collection and integration practices. Organizations need to identify and capture data from various sources within the supply chain ecosystem. This involves leveraging data from enterprise resource planning (ERP) systems, Internet of Things (IoT) devices, RFID technology, and external data streams.
Integration is crucial to harmonize data from disparate sources. Implementing middleware and data integration platforms ensures seamless data flow, enabling a unified view of the supply chain. This step lays the foundation for meaningful analysis and insights.
Tools and Technologies
Selecting the right tools and technologies is pivotal for SCM data analytics success. Invest in advanced analytics platforms, data warehouses, and business intelligence tools that suit your organization's needs. Machine learning and artificial intelligence can further enhance predictive and prescriptive analytics capabilities, providing valuable insights into demand forecasting, inventory optimization, and logistics planning.
Furthermore, consider cloud-based solutions for scalability and accessibility. Cloud platforms offer the flexibility to store and process large volumes of data, making them increasingly popular for SCM analytics.
Building a Data-Driven Culture
A data-driven culture is essential to maximize the benefits of SCM analytics. Organizations should promote a mindset where data is seen as a valuable asset. To cultivate this culture, provide training and education to employees at all levels, enabling them to understand and interpret data insights. Encourage cross-functional collaboration, where SCM professionals work closely with data analysts and IT teams to harness the power of data.
Leadership plays a critical role in fostering this culture by setting a clear example of data-driven decision-making. Additionally, establish key performance indicators (KPIs) that align with SCM analytics goals, reinforcing the importance of data in achieving strategic objectives.
Benefits of Data-Driven SCM Optimization
Implementing data-driven supply chain management (SCM) optimization brings forth a multitude of advantages for organizations:
-
Cost Reduction through Inventory Optimization: Data analytics allows for precise inventory management by analyzing demand patterns and supplier performance. This optimization minimizes excess inventory holding costs and avoids stockouts, resulting in substantial cost savings.
-
Improved Demand Forecasting and Planning: Accurate demand forecasting is made possible through data analytics. This helps organizations align their production and inventory levels with actual market demand, reducing waste and ensuring product availability when needed.
-
Enhanced Customer Service and Satisfaction: Data-driven SCM ensures timely deliveries and improved order accuracy, leading to enhanced customer experiences. Satisfied customers are more likely to become loyal, repeat buyers.
-
Reduced Lead Times and Increased Operational Efficiency: SCM analytics streamlines processes, reducing lead times across the supply chain. This increased efficiency not only lowers operational costs but also enables quicker response to market fluctuations and changes in customer demand.
leveraging data-driven SCM optimization is a strategic move that not only enhances cost-efficiency but also elevates overall supply chain performance, ultimately benefiting both the organization and its customers.
Challenges and Considerations
Implementing data analytics in supply chain management comes with its set of challenges and important considerations. One significant challenge is the complexity of integrating diverse data sources from various parts of the supply chain. Ensuring seamless data flow and compatibility between different systems and platforms can be a formidable task.
Data security and privacy also emerge as critical concerns. With the increasing reliance on digital information, safeguarding sensitive data against cyber threats and complying with data protection regulations are paramount.
Additionally, the accuracy and reliability of the data itself can pose a challenge. Inaccurate or incomplete data can lead to flawed insights and misguided decisions. Rigorous data validation and cleaning processes are necessary to mitigate this risk.
Furthermore, there may be resistance to change within the organization. Shifting towards a data-driven culture requires buy-in from all levels of the workforce. Providing adequate training and support for employees to adapt to these new processes is crucial.
Lastly, cost considerations cannot be overlooked. Implementing robust data analytics systems and maintaining them can entail significant investments. Balancing the potential benefits against the associated costs is a key consideration for any organization.
Addressing these challenges and carefully considering these factors will be instrumental in ensuring the successful integration of data analytics into supply chain management operations.
Future Trends in Data Analytics for SCM
The landscape of data analytics in supply chain management (SCM) is poised for exciting advancements. Artificial Intelligence (AI) and Machine Learning (ML) are set to play an even more significant role, enabling predictive and prescriptive analytics to reach unprecedented levels of accuracy and automation.
Blockchain technology is another transformative trend. Its decentralized and immutable ledger system holds immense potential for enhancing transparency, traceability, and security in SCM. Smart contracts within blockchain can streamline contract management and automate transactions, reducing friction in the supply chain.
Edge computing is anticipated to gain prominence. By processing data closer to its source, edge computing reduces latency, making real-time decision-making possible. This is particularly valuable in SCM scenarios where split-second responses are critical.
The Internet of Things (IoT) will continue to expand its footprint in SCM. With a proliferation of connected devices, organizations can gather real-time data on the condition, location, and status of goods throughout the supply chain. This enables proactive decision-making and improved resource allocation.
Lastly, augmented reality (AR) and virtual reality (VR) technologies are poised to revolutionize SCM training, operations, and maintenance. These immersive technologies offer the potential to enhance employee training, optimize warehouse layouts, and streamline maintenance processes.
Staying abreast of these trends will be pivotal for organizations looking to stay competitive and agile in the ever-evolving realm of supply chain management. Embracing these technologies will not only optimize operations but also open up new avenues for innovation and efficiency.
Data analytics plays a pivotal role in revolutionizing Supply Chain Management (SCM). Throughout this outline, we've explored its significance, various analytical approaches, and ethical considerations. By harnessing the power of data analytics, businesses can gain deeper insights, streamline operations, and ultimately achieve a more agile and efficient supply chain. It's not just an option; it's a necessity for organizations looking to thrive in today's competitive landscape. Embracing data-driven SCM practices is the way forward.