The Data Science Life Cycle
Discover the key stages of the data science life cycle and unlock the power of data-driven insights. Boost your analytics journey now!
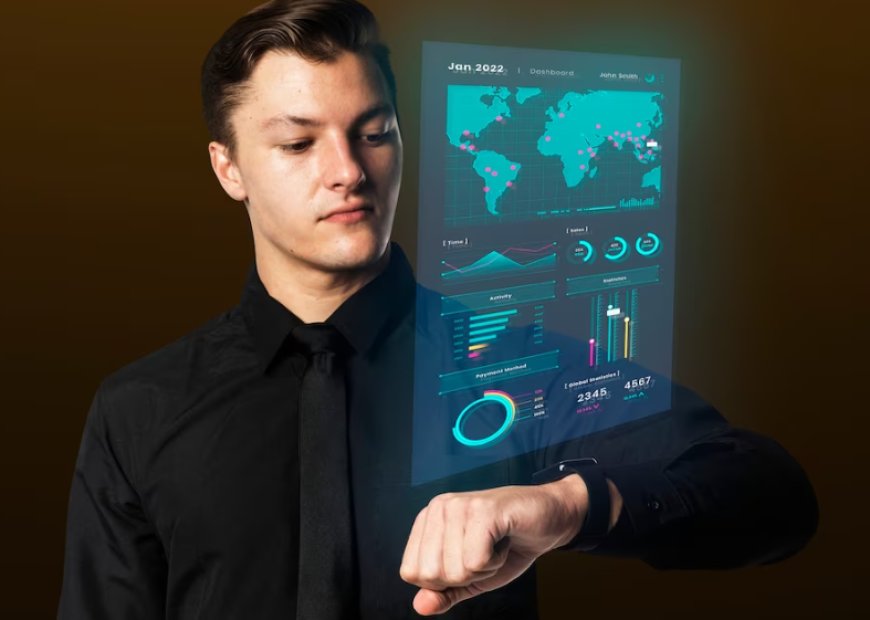
Hey there, data enthusiasts! Have you ever wondered about the secret recipe behind those mind-blowing data-driven insights? Well, let me tell you, it all begins with the data science life cycle. The data science life cycle is like a magical journey that takes raw data and transforms it into valuable insights. In this blog, we'll explore the various stages of the data science life cycle and unravel the secrets behind this captivating process.
1. Define the Problem:
Defining the problem is a critical step in problem-solving and decision-making processes. It involves clearly understanding and articulating the issue or challenge that needs to be addressed. By defining the problem accurately, individuals and teams can focus their efforts on finding the most effective solutions.
To define a problem, it is important to gather relevant information and insights. This may involve conducting research, analyzing data, and seeking input from stakeholders or subject matter experts. The goal is to gain a comprehensive understanding of the situation, its underlying causes, and the potential impact on various aspects.
Once the information is gathered, it is necessary to break down the problem into its key components or elements. This helps in identifying the specific aspects that need to be addressed and allows for a more targeted approach to finding solutions. The problem definition should be specific, measurable, achievable, relevant, and time-bound (SMART), providing clarity and guiding the problem-solving process.
2. Data Collection and Exploration:
Data Collection and Exploration are the initial steps in the data science journey. It involves gathering relevant data from various sources and embarking on a quest to uncover hidden insights. Data collection encompasses identifying the right sources, whether it's databases, APIs, or external datasets, and extracting the data in a structured format. Once the data is collected, data scientists dive into exploration. They analyze, visualize, and manipulate the data to identify patterns, trends, and anomalies. Exploratory data analysis allows for a deeper understanding of the data, uncovering valuable insights that form the basis for further analysis and modeling. It's like unraveling a puzzle, where each piece of data provides a clue to the bigger picture. So, let the data collection and exploration phase ignite your curiosity and set the foundation for your data-driven discoveries.
3. Data Preprocessing and Cleaning:
As you dig deeper into the data, you might stumble upon some messy surprises. Fear not! Data preprocessing and cleaning come to the rescue. In this stage, data scientists clean up the data, handle missing values, address outliers, and transform the data into a format suitable for analysis. It's like polishing gemstones to reveal their true beauty.
4. Model Building and Training:
Now comes the exciting part—the model building stage. Data scientists select the most suitable algorithms and techniques to build predictive or descriptive models. They train these models using historical data and tweak them to achieve the best performance. It's a bit like teaching a model to recognize patterns and make intelligent predictions based on the data it has been trained on.
5. Model Evaluation and Validation:
Before we get too carried away, it's important to evaluate and validate the models. In this stage, data scientists assess the performance of the models against predefined metrics. They use techniques like cross-validation and holdout sets to ensure the models are robust and reliable. It's like putting our models to the test to see if they can truly predict the future or uncover valuable insights.
6. Deployment and Implementation:
Deployment and implementation in data science play a crucial role in bridging the gap between data analysis and real-world applications. Data science encompasses various techniques and algorithms for extracting insights from data, but the value of these insights can only be realized when they are deployed and integrated into practical solutions.
One aspect of deployment in data science is the transformation of analytical models into production-ready systems. This involves taking the trained models and integrating them into existing software infrastructure or developing new applications that leverage the insights derived from the data. This process requires collaboration between data scientists, software engineers, and domain experts to ensure that the models are implemented accurately and efficiently.
Deployment also includes considerations for data governance and privacy. Data scientists must ensure compliance with regulations and ethical standards regarding data privacy and security. Implementing measures such as anonymization, encryption, and access controls helps protect sensitive information and maintain data integrity throughout the deployment process.
7. Monitoring and Maintenance:
Data science is not a one-time affair—it's an ongoing process. Once the models are deployed, they need to be monitored and maintained. Data scientists keep a close eye on the performance of the models, retrain them periodically with fresh data, and make necessary adjustments as new insights or challenges arise. It's like nurturing a living organism that constantly adapts and evolves.
8. Iteration and Improvement:
Data science is a continuous learning process, and iteration is key. Once the models are deployed and insights are generated, it's essential to gather feedback, analyze the results, and refine the models. Data scientists often iterate through the various stages of the life cycle, making improvements and incorporating new data to enhance the accuracy and relevance of their models.
9. Communication and Visualization:
Communication and visualization are integral components of data science that bring insights to life. In the realm of data, where complex information abounds, effective communication is essential to convey findings and drive decision-making. Visualization techniques, such as charts, graphs, and interactive dashboards, allow data scientists to present data in a visually appealing and easily understandable manner. By distilling complex patterns and trends into intuitive visual representations, communication and visualization empower both technical and non-technical stakeholders to grasp the significance of the data. It bridges the gap between data experts and decision-makers, enabling collaborative discussions and informed actions. A well-crafted visualization can tell a compelling story, making data-driven insights accessible, actionable, and impactful. So, let your data speak through visual storytelling and communicate the power of insights to drive positive change.
10. Ethical Considerations:
As data scientists, we bear the responsibility of handling sensitive and personal data. It's essential to approach the data science life cycle with ethical considerations in mind. This involves ensuring data privacy, maintaining data security, and adhering to ethical guidelines when using algorithms or making decisions based on data-driven insights. Upholding ethical practices builds trust and safeguards the integrity of the entire data science process.
11. Collaboration and Teamwork:
Data science is rarely a solitary endeavor. It thrives on collaboration and teamwork. Data scientists often work in interdisciplinary teams, partnering with domain experts, data engineers, and business stakeholders. Effective collaboration ensures a holistic approach to problem-solving and fosters innovative ideas. Embrace collaboration, share knowledge, and learn from the diverse perspectives of your team members.
12. Continuous Learning and Professional Development:
Data science is a rapidly evolving field, and staying up to date with the latest tools, techniques, and advancements is crucial. Embrace a mindset of continuous learning and professional development. Attend workshops, conferences, and training programs to expand your knowledge and network with fellow data enthusiasts. Engage in online communities, read industry blogs, and participate in data challenges to sharpen your skills and stay ahead of the curve.
With keeping this in the mind, Data Science life cycle is a thrilling journey that transforms raw data into valuable insights and empowers decision-making. From problem definition to model deployment, each stage plays a crucial role in the process. Embrace the iterative nature of data science, communicate insights effectively, and uphold ethical considerations along the way.