AI and Explainability: Demystifying the Magic of Artificial Intelligence
Explore the concept of AI explainability in simple terms. Demystify the workings of Artificial Intelligence and understand how it makes decisions.
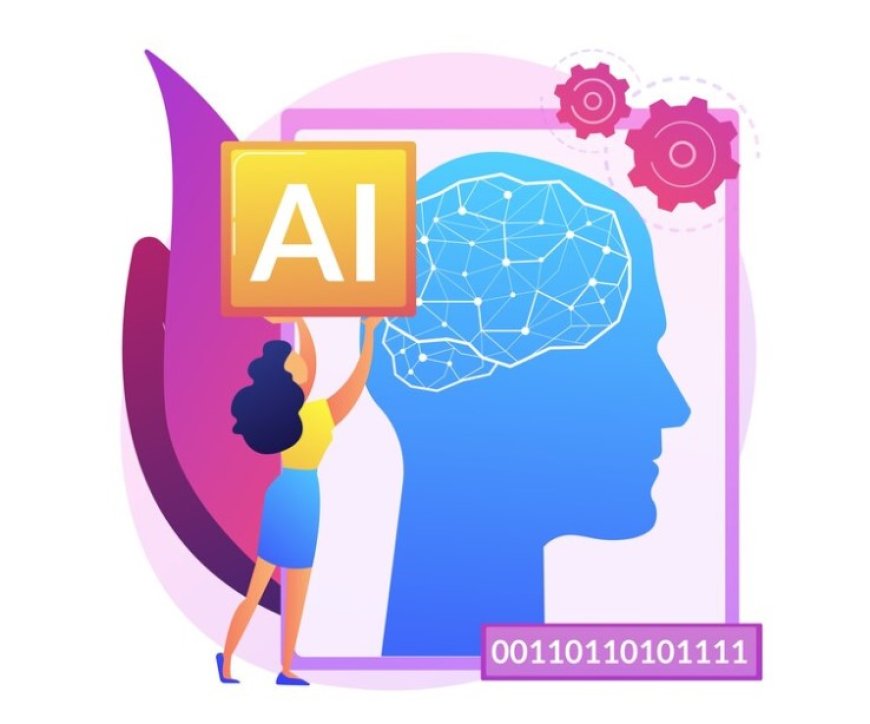
Artificial Intelligence (AI) has become an integral part of our lives, revolutionizing various industries and transforming the way we interact with technology. From personalized recommendations to autonomous vehicles, AI is making remarkable advancements. However, as AI becomes more sophisticated, a critical question arises: Can we trust AI systems if we don't understand how they make decisions? This is where the concept of AI explainability comes into play. We will delve into the fascinating world of AI and explainability, demystifying the magic behind AI algorithms and exploring the importance of transparency in building trustworthy and ethical AI systems.
AI and its Black Box Problem :
AI algorithms are complex and often operate as black boxes, meaning that their inner workings are not readily understandable to humans. They analyze vast amounts of data, recognize patterns, and make predictions based on statistical models. While this black box approach allows AI to achieve impressive results, it can also pose challenges when it comes to understanding how and why certain decisions are made.
The Importance of AI Explainability :
AI explainability refers to the ability to understand and interpret the decisions and actions of AI systems. It plays a crucial role in building trust, accountability, and ethical considerations in AI. Imagine relying on an AI system for medical diagnoses or loan approvals without being able to explain the reasoning behind its decisions. Lack of transparency could lead to biased outcomes, legal implications, and a loss of trust in AI technologies.
Techniques for Explainability
Firstly, model interpretability methods allow us to understand how AI models arrive at their decisions. This involves dissecting the internal workings of the model to reveal which features or inputs hold the most sway over the output.
Additionally, tools like LIME (Local Interpretable Model-agnostic Explanations) and SHAP (Shapley Additive Explanations) provide practical means to break down complex AI outputs into understandable components. These tools enable us to grasp the specific contributions of each input variable to the final prediction.
Furthermore, visualizing AI decision-making offers a powerful way to convey complex processes in a more intuitive manner. Through graphical representations and interactive interfaces, stakeholders can gain a clearer understanding of how AI models arrive at their conclusions.
Together, these techniques form a crucial toolkit for demystifying the inner workings of artificial intelligence, making it more transparent and accessible to a wider audience.
Advancing Explainable AI Techniques :
Researchers and experts are actively working on developing techniques to enhance the explainability of AI systems. One approach is to use interpretable machine learning models that provide insights into how the decisions are made. These models prioritize transparency over complexity, allowing users to understand the factors influencing the outcomes.
Another technique is post hoc explainability, where AI systems generate explanations for their decisions after the fact. This approach aims to provide a human-readable explanation of the decision-making process, enabling users to assess the validity and fairness of the AI system's outputs.
Additionally, there are efforts to integrate transparency into the design and development of AI systems from the beginning. This includes adopting ethical guidelines, incorporating user feedback, and ensuring that AI systems are accountable and fair.
The Benefits of Explainable AI :
Explainable AI offers several benefits beyond trust and transparency. It enables users to identify biases and discriminatory patterns in AI systems, leading to fairer outcomes. Explainability also helps in debugging and improving the performance of AI algorithms by providing insights into their strengths and weaknesses.
Furthermore, explainable AI fosters human-AI collaboration. When users can understand and interpret the decisions made by AI systems, they can work together more effectively. This collaboration can lead to better-informed decisions, improved user experiences, and increased acceptance of AI technologies.
Real-World Applications of Explainable AI :
Explainable AI has applications in various fields, including healthcare, finance, and law enforcement. In healthcare, explainable AI can assist doctors in understanding the reasoning behind AI-generated diagnoses, allowing for better-informed decisions and reducing medical errors. In finance, it can help regulators and auditors understand the factors influencing AI-driven trading decisions, ensuring fairness and compliance. In law enforcement, explainable AI can provide insights into the decision-making process of predictive policing systems, enabling accountability and addressing concerns related to bias and discrimination.
Challenges and Future Directions :
While progress has been made in developing explainable AI techniques, challenges remain. Balancing transparency with the need for complex AI systems that deliver accurate results is a delicate task. The interpretability of AI models often comes at the cost of performance. Striking the right balance between accuracy and explainability will be a crucial area of focus in the future.
Additionally, establishing standards and regulations around AI explainability is essential to ensure ethical and responsible AI use. This includes addressing issues such as data privacy, algorithmic bias, and the potential manipulation of explanations.
With the rapid advancement of AI technology, the journey towards achieving full AI explainability is ongoing. Researchers and practitioners are committed to finding innovative solutions that strike the right balance between accuracy and transparency. They are exploring techniques such as model-agnostic methods, rule-based approaches, and visualization tools to provide intuitive explanations without sacrificing performance.
In addition to technical challenges, there are also ethical considerations surrounding AI explainability. Ensuring that explanations are understandable, unbiased, and respectful of privacy is crucial. AI systems must be accountable, and users should have the right to question and challenge the decisions made by these systems.
Looking ahead, the future of AI explainability holds great promise. As more organizations and industries recognize the significance of transparency, there is an increasing demand for explainable AI systems. Governments and regulatory bodies are also taking notice, with discussions on the need for policies and standards to govern AI explainability.
Moreover, public awareness and education about AI explainability are essential. By promoting a better understanding of how AI works and its limitations, we can empower individuals to make informed decisions and engage in meaningful discussions about the impact of AI on society.
Lastly,With the rapid advancement of AI technology, the journey towards achieving full AI explainability is ongoing. Researchers and practitioners are committed to finding innovative solutions that strike the right balance between accuracy and transparency. They are exploring techniques such as model-agnostic methods, rule-based approaches, and visualization tools to provide intuitive explanations without sacrificing performance.
In addition to technical challenges, there are also ethical considerations surrounding AI explainability. Ensuring that explanations are understandable, unbiased, and respectful of privacy is crucial. AI systems must be accountable, and users should have the right to question and challenge the decisions made by these systems.
Looking ahead, the future of AI explainability holds great promise. As more organizations and industries recognize the significance of transparency, there is an increasing demand for explainable AI systems. Governments and regulatory bodies are also taking notice, with discussions on the need for policies and standards to govern AI explainability.Moreover, public awareness and education about AI explainability are essential. By promoting a better understanding of how AI works and its limitations, we can empower individuals to make informed decisions and engage in meaningful discussions about the impact of AI on society.