What Are the Applications of Machine Learning?
The different applications of machine learning, from healthcare to finance and beyond. Learn how ML is transforming industries and shaping our future.
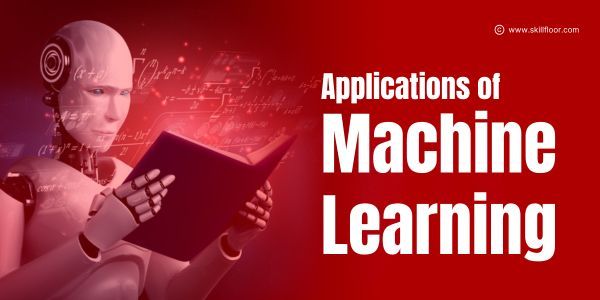
Understanding the Basics of Machine Learning
Machine learning (ML) is changing the way we live and work every day. But what does it actually do? Simply put, ML is a way for computers to learn from data and make decisions without being specifically programmed for each task. From improving our health to making shopping more fun, it’s already everywhere.
Let’s look at where and how machine learning is being used, breaking it down into different areas that impact our daily lives.
Why It Matters: Making Sense of Machine Learning
Many people don’t realise how often they interact with ML. It’s working quietly in the background, helping businesses, schools, hospitals, and many other sectors work better. To understand why it’s so important, let's explore its real-world applications, making complex uses easy to grasp.
Applications of Machine Learning
1. Healthcare: Transforming Patient Care
Machine learning has a transformative effect on healthcare, addressing complex problems and improving patient outcomes. As someone deeply interested in its practical value, I can’t help but highlight a few crucial areas:
-
Disease Diagnosis: One standout application is using ML models to detect diseases, often earlier and with more precision than human doctors. For example, ML-powered algorithms analyze imaging data, such as X-rays and MRIs, spotting tumors that may escape a human’s eyes. Consider the case of breast cancer screening where machine learning systems now achieve highly accurate results, helping detect cancer in its earliest stages.
-
Medical Imaging: Algorithms can analyze thousands of images to identify patterns indicative of medical conditions. Deep learning networks, a subset of ML, excel at this, transforming medical fields such as radiology, pathology, and ophthalmology.
-
Personalized Medicine: Traditional medicine offers treatments based on broad populations, but machine learning allows for the personalization of therapies. By considering a patient’s genetics, environment, and lifestyle, ML-based systems recommend individualized treatment plans, maximizing efficacy and minimizing side effects.
-
Predictive Analytics in Patient Care: Healthcare providers are using ML to predict patient outcomes, readmission risks, and disease progression. Predictive tools analyze historical patient data, alerting healthcare professionals to potential issues.
2. Finance: Enhancing Security and Efficiency
Financial institutions were early adopters of machine learning due to its ability to quickly process vast amounts of data. Here’s how it’s changing finance:
-
Fraud Detection: Financial fraud evolves constantly. ML systems detect suspicious behavior by analyzing transaction patterns. When something seems “off”—like an unusual purchase location or an abnormal spending spree—ML models alert authorities in real time.
-
Algorithmic Trading: ML powers complex trading algorithms that analyze data, news, and market trends in seconds. These systems make data-driven decisions and execute trades much faster than human brokers.
-
Credit Scoring and Risk Assessment: Financial organizations use ML models to assess an individual’s creditworthiness by analyzing various factors like income, debt history, spending habits, and more. This makes the loan approval process fairer and faster.
-
Customer Support Automation: ML-driven chatbots have become the norm in customer service. They handle common customer inquiries, reducing human workload while maintaining high efficiency.
3. Retail and E-commerce: Tailoring Customer Experiences
In retail and e-commerce, the customer experience reigns supreme. ML’s ability to personalize user interactions is revolutionizing the sector.
-
Product Recommendations: E-commerce platforms like Amazon rely heavily on ML models that analyze a user’s browsing and purchase history to offer tailored product recommendations. This personalization drives higher sales conversions and improves customer satisfaction.
-
Dynamic Pricing: ML models adjust product prices based on market demand, competitor pricing, and historical sales data. This ensures that businesses stay competitive and maximize profitability.
-
Inventory Management: Predictive analytics helps businesses optimize inventory by forecasting demand. This reduces excess stock and prevents shortages, leading to better resource allocation.
-
Customer Sentiment Analysis: Retailers analyze customer feedback using natural language processing (NLP) to understand sentiment toward products or services. Insights derived from this analysis inform marketing and product development.
4. Marketing and Advertising: Precision Targeting
As a content creator in the digital marketing space, I’ve seen firsthand how ML revolutionizes marketing:
-
Targeted Advertising: Machine learning algorithms track user behavior, building profiles that allow marketers to deliver highly personalized ads. By understanding a user’s preferences, ML-driven campaigns are far more relevant and effective.
-
Churn Prediction: For businesses, keeping existing customers is crucial. ML algorithms analyze user data to detect signs of dissatisfaction, predicting the likelihood of churn. This allows for timely intervention, increasing customer retention.
-
Customer Segmentation: ML algorithms group customers with similar characteristics for highly targeted marketing efforts. This segmentation is dynamic, evolving as customer behavior changes.
-
Automating A/B Testing: Marketing teams can automate the testing of ad campaigns, landing pages, or email templates. ML identifies which variations perform best and optimizes accordingly.
5. Manufacturing and Supply Chain: Boosting Efficiency
In manufacturing, efficiency and quality are key. Machine learning streamlines operations, helping businesses produce more with less.
-
Predictive Maintenance: Manufacturing facilities use sensors to monitor machinery performance. ML analyzes this data to predict failures before they occur, preventing costly downtime and extending equipment life.
-
Quality Control: ML-powered computer vision inspects products for defects. By analyzing product images, these systems can detect flaws faster and more accurately than humans, ensuring high-quality output.
-
Demand Forecasting: Supply chain management benefits from ML’s predictive capabilities. By analyzing historical data and external factors, ML systems accurately forecast demand, enabling better production and inventory planning.
6. Transportation and Automotive: Driving Innovation
Machine learning is at the forefront of reshaping transportation.
-
Autonomous Vehicles: Self-driving cars use ML to interpret sensor data, navigate complex environments, and make real-time decisions. Companies like Tesla, Waymo, and others continuously improve their models through large-scale data collection and analysis.
-
Traffic Predictions: Apps like Google Maps utilize machine learning to provide accurate traffic predictions and suggest optimal routes, saving drivers time.
-
Fleet Management: ML solutions optimize fleet operations, reducing costs and improving efficiency by analyzing data on vehicle performance, fuel usage, and driver behavior.
7. Natural Language Processing (NLP) and Chatbots: Enhancing Communication
NLP is transforming how we interact with technology and access information.
-
Chatbots and Virtual Assistants: ML-driven chatbots and voice assistants like Siri, Alexa, and Google Assistant can interpret and respond to natural language. This simplifies customer support and everyday tasks.
-
Language Translation: Machine learning algorithms power real-time translation, making communication seamless across different languages.
-
Sentiment Analysis: Businesses use NLP to analyze social media posts, reviews, and feedback, deriving insights into customer opinions and preferences.
8. Image and Video Recognition: New Frontiers in Visual Analysis
Image and video recognition are rapidly expanding fields, with applications across security, social media, and beyond.
-
Facial Recognition: Used for security, personalization, and social media tagging, ML systems identify individuals with high accuracy.
-
Content Moderation: Social media platforms deploy ML algorithms to detect and remove inappropriate content, enhancing user safety.
-
Object Detection: ML-powered systems identify objects in photos or videos, used in applications like self-driving cars, surveillance, and more.
9. Cybersecurity: Staying Ahead of Threats
Machine learning helps combat ever-evolving cyber threats.
-
Threat Detection and Mitigation: ML systems identify suspicious network activity and alert security teams in real-time.
-
Anomaly Detection: By learning what normal behavior looks like, ML algorithms quickly spot deviations that may indicate breaches or attacks.
10. Energy and Utilities: Optimizing Resource Usage
Machine learning optimizes energy management, reducing waste and improving resource allocation.
-
Energy Load Forecasting: Predicting energy demand allows providers to better manage energy grids.
-
Smart Grids: ML systems balance energy loads, integrate renewable sources, and ensure consistent supply.
11. Entertainment and Media: Personalized Experiences
Machine learning shapes the way we consume content.
-
Content Recommendations: Platforms like Netflix and Spotify use ML algorithms to suggest shows, movies, or songs based on our preferences.
-
Content Creation: AI-driven tools support creative professionals in generating music, editing videos, and writing scripts.
12. Human Resources: Streamlining Hiring and Retention
HR departments benefit from ML’s ability to automate processes.
-
Talent Acquisition: ML tools analyze resumes and match candidates to job openings, saving time and improving the recruitment process.
-
Employee Retention: ML models predict employee churn and identify strategies to improve retention.
13. Agriculture: Precision and Productivity
Machine learning makes agriculture smarter and more efficient.
-
Crop Monitoring: Drones and sensors collect data on crop health, which is then analyzed to predict yields and detect diseases.
-
Precision Farming: ML-driven systems optimize resource use, increasing crop productivity while minimizing waste.
14. Education: Personalizing Learning Experiences
Education is another area where ML is making strides.
-
Personalized Learning: ML systems adapt lessons to fit each student’s learning pace, providing a more tailored educational experience.
-
Automated Grading: Machine learning speeds up grading and provides consistent assessments.
How Will Machine Learning Change the Future?
Reflecting on all these uses, it's clear that machine learning isn't just a trendy term—it's a powerful tool shaping our everyday lives. The future looks even brighter as different industries push their limits, improve algorithms, and find new ways to use this technology. Whether it’s making healthcare better, guiding financial choices, or creating more personalized experiences, machine learning is here to stay as a major driver of progress.
As I continue to learn about this exciting field, one thing is obvious—machine learning isn’t something far off in the future; it’s already a big part of our lives and is growing rapidly. The possibilities are endless, and we’re only just beginning to understand its full potential.
Machine learning is already a big part of our lives, making everyday tasks smarter and more efficient. From improving healthcare and finance to enhancing shopping experiences and more, its impact is everywhere. As it keeps evolving, the possibilities are endless, showing us that machine learning isn't just a part of the future—it's shaping our present in powerful ways.