Concept Learning in Machine Learning: Unveiling the Path to Intelligent Systems
"Unlocking the Future of Machine Learning: Explore the Power of Concept Learning for Intelligent Systems. Discover the Path to Innovation!"
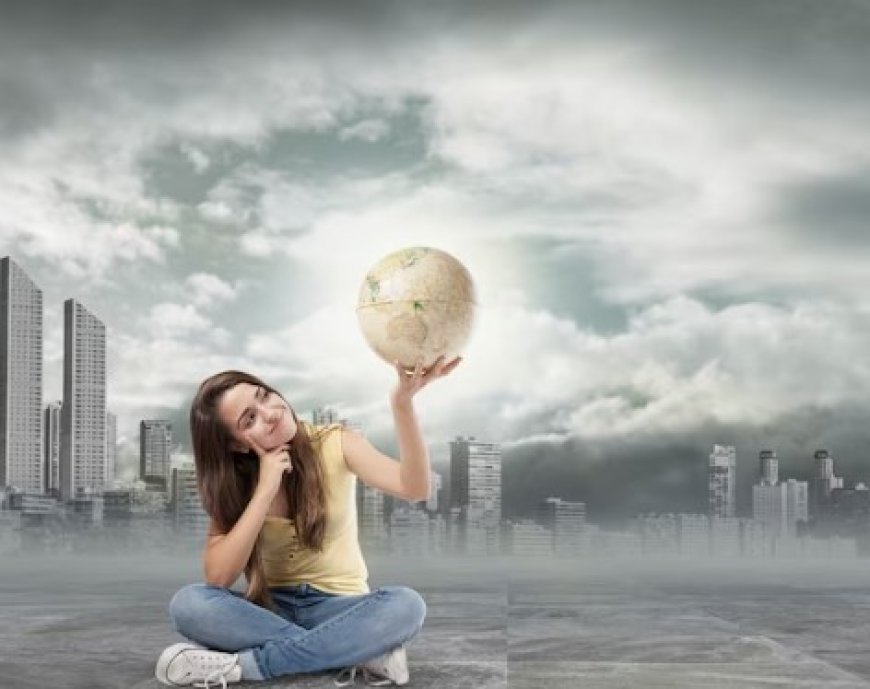
Welcome to the world of machine learning, where algorithms and data converge to enable intelligent systems. At the core of this fascinating field lies the concept of concept learning—a fundamental process that allows machines to acquire knowledge and make informed decisions. In this blog, we will embark on an enlightening journey to understand the intricacies of concept learning in machine learning. Fear not, for we will explore this topic in a friendly and approachable manner, ensuring that you grasp the essence of concept learning and its significance in building intelligent systems. So, let's delve into the captivating world of concept learning and unravel the path to creating machines that can understand and generalize from data.
Understanding Concepts and Concept Learning
Before we dive into the depths of concept learning in machine learning, let's start by defining what concepts are. In simple terms, a concept represents a general idea or a category that encompasses a set of objects or instances sharing common characteristics. For example, the concept of "cat" represents a category of animals with specific features such as fur, whiskers, and meowing.
Concept learning, in the context of machine learning, refers to the process of acquiring and generalizing concepts from a given set of examples or data. It involves teaching machines to recognize patterns, infer rules, and make predictions based on observed instances. By learning concepts, machines gain the ability to classify new, unseen instances into predefined categories, enabling them to understand and interpret the world around us.
The Importance of Concept Learning
Concept learning is a fundamental building block of intelligent systems. It allows machines to acquire knowledge and make informed decisions based on that knowledge. By learning concepts, machines can generalize from limited examples and apply their acquired knowledge to new and unseen instances. This ability to generalize is crucial for machines to navigate complex environments, adapt to changing circumstances, and provide accurate and reliable predictions.
Concept learning also enables machines to identify similarities and differences between objects, recognize patterns, and extract meaningful insights from data. This is particularly valuable in various fields such as image recognition, natural language processing, recommendation systems, and medical diagnosis. By understanding concepts, machines can assist humans in diverse domains, improving efficiency, accuracy, and decision-making processes.
Concept Learning Approaches
In machine learning, there are different approaches to concept learning, each with its own set of techniques and algorithms. Let's explore a few popular approaches:
Supervised Learning: In supervised learning, machines learn concepts by training on labeled examples, where each instance is associated with a predefined category or label. The learning algorithm leverages these labeled examples to build a model that can classify new, unseen instances accurately.
Unsupervised Learning: Unsupervised learning involves learning concepts from unlabeled data, where machines aim to discover underlying patterns or structures in the data without predefined categories. Clustering algorithms are commonly used in unsupervised learning to group similar instances together based on their intrinsic characteristics.
Reinforcement Learning: In reinforcement learning, machines learn concepts through interactions with an environment, receiving rewards or penalties based on their actions. By exploring the environment and optimizing their actions, machines learn to make decisions that maximize their cumulative rewards.
The process of concept learning typically involves steps such as data collection, preprocessing, feature extraction, model training, and evaluation. The choice of approach depends on the nature of the problem, the availability of labeled data, and the desired outcome.
Benefits and Applications of Concept Learning
Concept learning offers a wide range of benefits and finds applications in various domains. Here are a few key benefits:
Accurate Predictions: By learning concepts, machines can make accurate predictions based on observed patterns and past examples. This is particularly valuable in fields such as finance, stock market prediction, weather forecasting, and disease diagnosis.
Decision Support: Concept learning enables machines to provide decision support by analyzing large volumes of data, identifying relevant factors, and extracting meaningful insights. This can assist businesses in making informed decisions, optimizing processes, and enhancing efficiency.
Personalization and Recommendations: By understanding user preferences and learning concepts from their behavior, machines can personalize recommendations and provide tailored experiences. This is widely used in recommendation systems, personalized marketing, and content curation.
Pattern Recognition: Concept learning allows machines to recognize patterns in various forms of data, such as images, text, and speech. This is crucial for tasks like image recognition, natural language processing, sentiment analysis, and speech recognition.
Exploring Challenges and Future Directions
While concept learning in machine learning has brought about remarkable advancements, it is not without its challenges. Some of the common hurdles include dealing with noisy or incomplete data, handling concept drift (where the underlying concepts change over time), and addressing bias in the training data that can lead to skewed or unfair predictions. Overcoming these challenges requires continuous research and development of robust algorithms and methodologies.
Moreover, as the field of concept learning evolves, there are exciting avenues for future exploration. Researchers are focusing on developing algorithms that can handle more complex concepts, adapt to dynamic environments, and integrate multiple sources of information for a richer understanding. There is also increasing emphasis on interpretable and explainable concept learning, ensuring that the decisions made by machines can be understood and trusted by humans.
Collaboration and knowledge-sharing among researchers, practitioners, and the broader community are vital for advancing concept learning. Open-source libraries, online forums, and conferences provide platforms for exchanging ideas, sharing best practices, and pushing the boundaries of concept learning.
Embracing the Journey of Concept Learning
As you delve deeper into the realm of concept learning in machine learning, remember that it is a journey of continuous learning and exploration. Embrace the challenges and be prepared to adapt and refine your approach as new techniques and algorithms emerge. Stay curious, experiment with different methodologies, and engage in practical applications to solidify your understanding.
Concept learning holds immense potential to revolutionize industries, shape decision-making processes, and enable intelligent systems. By honing your skills in concept learning, you become a valuable contributor to the field, unlocking new possibilities and driving innovation.
So, with enthusiasm and determination, embark on this exciting journey of concept learning. Immerse yourself in the wealth of resources available, connect with fellow learners and experts, and let your passion for understanding and applying concepts guide you towards creating intelligent systems that have a positive impact on our world.
May your concept learning endeavors be rewarding, enlightening, and filled with exciting discoveries. Happy learning, and may your journey in concept learning be marked by continuous growth and success!
We have explored the essence of concepts, the significance of concept learning, and different approaches such as supervised, unsupervised, and reinforcement learning. We have also highlighted the benefits of concept learning, including accurate predictions, decision support, personalization, and pattern recognition.
While concept learning presents exciting opportunities, it also comes with challenges such as noisy data, concept drift, and bias. Overcoming these challenges requires ongoing research, innovation, and collaboration among researchers, practitioners, and the wider community.
To summarize,Concept learning paves the way for the creation of intelligent systems that can understand and generalize from data, leading to better decision-making, personalized experiences, and improved efficiency. So, let your passion for concept learning guide you as you navigate this dynamic field, and may your endeavors be marked by continuous growth and success.