The Role of AI and Machine Learning in Cybersecurity
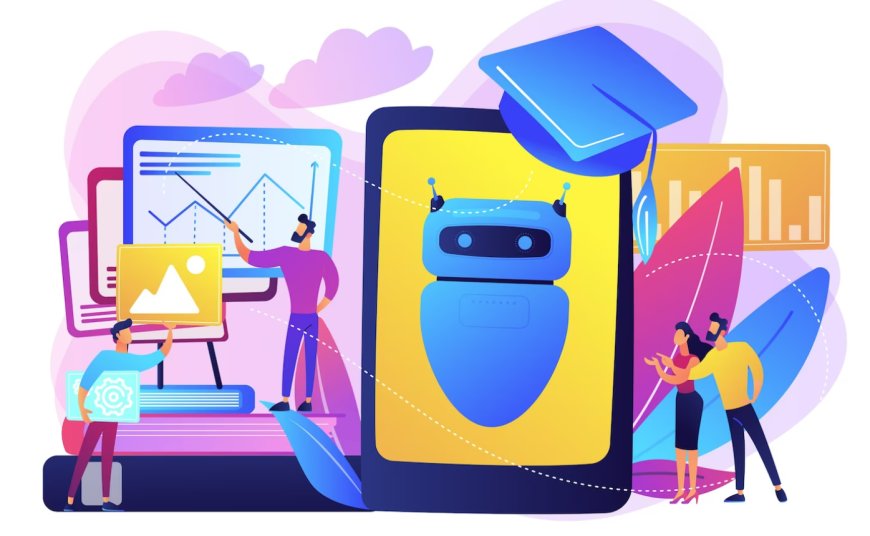
In the introduction of the discussion on the role of AI and Machine Learning in Cybersecurity, we begin by defining AI and Machine Learning. AI refers to the development of computer systems that can perform tasks that typically require human intelligence, while Machine Learning is a subset of AI that involves algorithms enabling systems to learn and improve from data. We also highlight the paramount importance of cybersecurity in today's digital age, where organizations and individuals face an increasing number of cyber threats and attacks. This sets the stage for understanding how AI and Machine Learning play a vital role in enhancing cybersecurity measures.
Fundamentals of Cybersecurity
Key Cybersecurity Challenges
The ever-evolving landscape of cyber threats poses significant challenges for organizations. These challenges include an increase in sophisticated cyberattacks, the constant emergence of new vulnerabilities, and the potential for data breaches. Cybersecurity professionals must grapple with these issues to protect sensitive information and critical systems effectively.
Traditional Approaches to Cybersecurity
Traditional cybersecurity approaches typically involve perimeter defense mechanisms, such as firewalls and antivirus software, to keep threats at bay. Additionally, security teams often rely on signature-based detection methods to identify known threats. While these methods have been effective to some extent, they are becoming less sufficient in combating modern, advanced cyberattacks.
Limitations of Traditional Approaches
Despite their historical effectiveness, traditional cybersecurity approaches have limitations. They struggle to defend against zero-day vulnerabilities and advanced persistent threats (APTs) that can go undetected for extended periods. Signature-based detection is not proactive and requires constant updates to stay relevant, making it less effective against rapidly evolving threats. As a result, there is a growing recognition of the need for more advanced and adaptive cybersecurity solutions.
Understanding AI and Machine Learning
Explanation of Artificial Intelligence
Artificial Intelligence (AI) is a field of computer science that focuses on creating intelligent systems capable of performing tasks that typically require human intelligence. These systems are designed to simulate human-like reasoning, learning, problem-solving, and decision-making processes. AI encompasses a wide range of technologies and techniques, including machine learning, natural language processing, computer vision, and more. It plays a pivotal role in revolutionizing various industries, including cybersecurity, by enabling computers to analyze vast amounts of data and make intelligent decisions to enhance security measures.
Introduction to Machine Learning
Machine Learning (ML) is a subset of AI that empowers computers to learn from data and improve their performance over time without being explicitly programmed. Instead of relying on explicit programming rules, ML algorithms identify patterns and relationships within data, allowing them to make predictions or decisions based on new, unseen data. In cybersecurity, ML is used to recognize and respond to evolving threats by continuously adapting and improving its detection and prevention capabilities.
Types of Machine Learning Algorithms
Machine learning algorithms can be categorized into several types:
-
Supervised Learning: In this approach, algorithms are trained on labeled data, with clear input-output pairs. They learn to map inputs to outputs and can make predictions or classifications based on new, unseen data.
-
Unsupervised Learning: Unsupervised algorithms work with unlabeled data and aim to discover patterns or groupings within the data without predefined categories. Clustering and dimensionality reduction are common applications in cybersecurity.
-
Reinforcement Learning: This type of learning involves an agent that interacts with an environment and learns to make decisions to maximize a reward signal. Reinforcement learning can be used in cybersecurity to optimize security strategies and responses.
-
Semi-Supervised Learning: This approach combines elements of both supervised and unsupervised learning by training models on a small amount of labeled data and a larger amount of unlabeled data. It's useful when labeled data is limited, which is often the case in cybersecurity.
Understanding these fundamental concepts of AI and ML is crucial for grasping how they contribute to improving cybersecurity measures.
AI and Machine Learning Applications in Cybersecurity
Threat Detection and Prevention
AI and machine learning excel in identifying anomalies and patterns within vast datasets, enabling early threat detection. These technologies continuously analyze network traffic, system logs, and user behavior to spot deviations from the norm. They can swiftly detect potential intrusions, malware, and other cyber threats, bolstering the security posture of organizations.
Predictive Analysis for Cyber Threats
AI and ML algorithms leverage historical data and advanced analytics to predict potential cyber threats. By analyzing past attack patterns and trends, they can forecast possible future attacks. This proactive approach empowers cybersecurity professionals to take preemptive measures, reducing vulnerabilities and minimizing the impact of potential breaches.
Identity and Access Management
AI and ML enhance identity and access management by employing biometrics, behavioral analysis, and contextual information to verify user identities. These technologies help ensure that only authorized individuals gain access to sensitive systems and data. They can adapt to evolving threats by continuously evaluating user behavior and access requests for potential risks.
Real-time Response and Mitigation
AI and ML enable rapid, automated responses to security incidents. When a threat is detected, these technologies can trigger immediate actions such as isolating compromised systems, blocking malicious traffic, or alerting security teams. This real-time response capability helps minimize damage and prevent the escalation of cyberattacks, strengthening overall cybersecurity defenses.
Benefits and Advantages
Improved Accuracy and Speed: AI and machine learning bring unprecedented levels of accuracy to cybersecurity. They can rapidly analyze vast amounts of data, identify patterns, and distinguish between legitimate activities and potential threats with exceptional precision. This enhanced accuracy reduces false positives and ensures that genuine threats are addressed promptly.
Adaptive Security Measures: AI-driven cybersecurity solutions continuously adapt to evolving threats. They learn from new data and can adjust security measures accordingly, making them highly effective in countering both known and unknown threats. This adaptability is crucial in the ever-changing landscape of cybersecurity.
Scalability and Automation: AI and ML solutions are inherently scalable, allowing organizations to handle increasing volumes of data and traffic without proportionally increasing the workforce.They can automate routine tasks such as threat detection, response, and patch management, freeing up cybersecurity professionals to focus on more complex and strategic aspects of security management. This scalability and automation significantly enhance an organization's overall cybersecurity posture.
Challenges and Concerns
-
Data Privacy and Ethics: The use of AI and machine learning in cybersecurity raises significant concerns regarding data privacy and ethical considerations. As organizations collect and analyze vast amounts of data, protecting sensitive information becomes paramount. Ensuring compliance with data protection regulations like GDPR is challenging. Moreover, there's a growing need to address ethical concerns related to data usage, algorithm bias, and potential misuse of AI for surveillance.
-
Adversarial Attacks: Cyber attackers are increasingly employing adversarial techniques to deceive AI-powered security systems. These attacks involve manipulating input data to trick AI models into making incorrect decisions. Developing robust AI defenses against adversarial attacks is a complex and ongoing challenge for cybersecurity experts.
-
Skill Gap and Resource Constraints: Implementing AI and ML in cybersecurity requires specialized skills and resources. Many organizations face a shortage of cybersecurity professionals with AI expertise. Training existing staff and acquiring the necessary technology can be costly and time-consuming, creating resource constraints for smaller companies and organizations with limited budgets. Bridging this skill gap is crucial for effective AI-driven cybersecurity.
Future Trends and Developments
Evolving Threat Landscape
As technology advances, so do cyber threats. Future trends in cybersecurity will undoubtedly be shaped by an evolving threat landscape. Hackers will continue to develop more sophisticated attack techniques, necessitating the constant evolution of AI and ML models to detect and respond to these threats effectively.
AI/ML Integration in Security Tools
The integration of AI and ML into security tools is expected to become more seamless and pervasive. These technologies will play a central role in threat detection, response, and mitigation, making security tools more intelligent and adaptive. The future will likely see greater automation and real-time analysis powered by AI and ML.
Ethical Considerations and Regulations
With the increasing use of AI and ML in cybersecurity, ethical considerations and regulatory frameworks will become paramount. Striking a balance between innovation and ethical use of these technologies is essential. Governments and organizations will need to collaborate to establish guidelines and regulations to ensure responsible AI and ML practices in the cybersecurity domain.
AI and Machine Learning have firmly established their indispensable role in the realm of cybersecurity. Their capacity to detect, analyze, and mitigate threats in real-time, adapt to evolving attack methods, and enhance overall security posture is undeniable. As we move forward, it is imperative for cybersecurity professionals and organizations to embrace and integrate these technologies as a fundamental component of their defense strategy. The growing significance of AI and ML in cybersecurity demands proactive adoption and continual innovation to stay ahead of the ever-evolving threat landscape.