Predictive Maintenance: Revolutionizing Supply Chain Reliability with Data Analytics
Discover how Predictive Maintenance is transforming supply chain reliability through Data Analytics. Learn how data-driven insights optimize operations and reduce downtime.
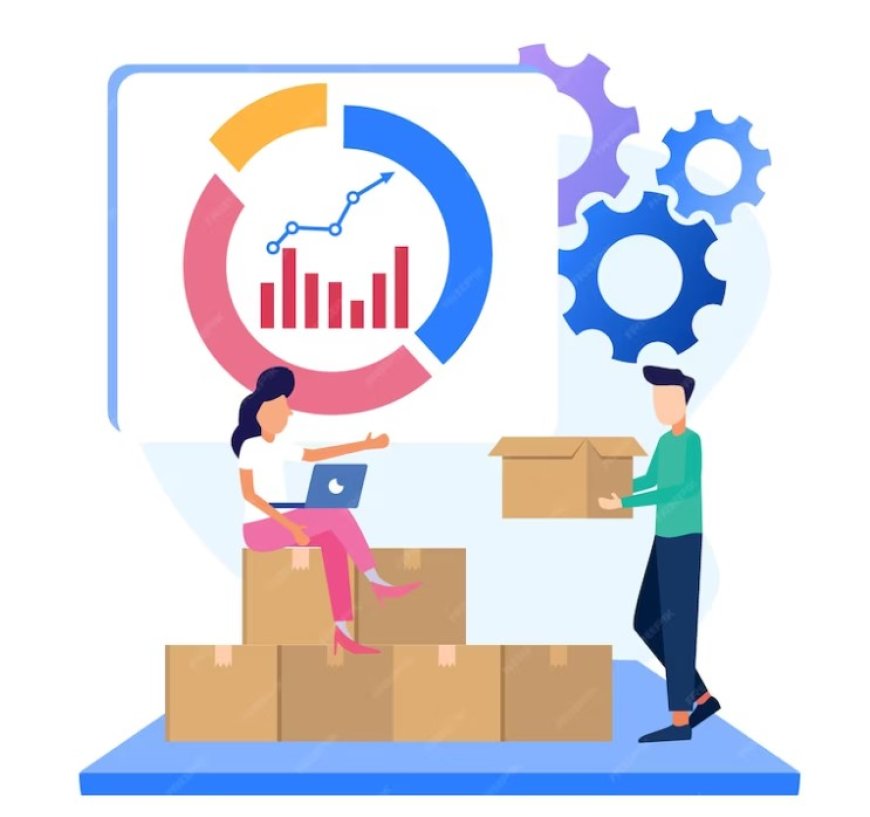
In the fast-paced world of supply chain management, reliability and efficiency are the cornerstones of success. The traditional methods of reactive or preventive maintenance have their limitations, often leading to unplanned disruptions and increased operational costs. However, there's a game-changing revolution taking place – predictive maintenance powered by data analytics. This innovative approach leverages real-time data, sensor technology, and advanced algorithms to predict equipment failures before they occur.
The Traditional Approach to Maintenance
-
Reactive Maintenance
Reactive maintenance is essentially a "break-fix" approach. In this method, maintenance activities are only initiated when a piece of equipment or machinery has already malfunctioned or broken down. This means that maintenance teams only spring into action once an issue becomes apparent or when the equipment has already failed. While this approach might seem cost-effective in the short term because it avoids planned maintenance expenses, it comes with significant downsides. These include unplanned downtime, which can be costly in terms of lost productivity and potential emergency repairs, which are often more expensive than planned maintenance.
-
Preventive Maintenance
Preventive maintenance, on the other hand, involves scheduled, routine maintenance activities based on time intervals or usage thresholds. These maintenance tasks are performed regardless of whether there are any observable issues with the equipment. For example, a company might decide to service its machinery every three months or replace a particular component every 10,000 operating hours. While preventive maintenance helps reduce the risk of equipment breakdowns and associated downtime, it has its drawbacks. It can lead to unnecessary maintenance, which increases operational costs, consumes resources, and disrupts production unnecessarily.
In both reactive and preventive maintenance approaches, there is a lack of data-driven decision-making. These strategies are typically based on fixed schedules or responses to breakdowns, rather than considering the actual condition of the equipment. As a result, they can be less efficient and more costly in the long run. To address these shortcomings, companies are increasingly turning to predictive maintenance, which leverages data analytics and technology to forecast when maintenance is genuinely needed, improving overall reliability and cost-effectiveness.
The Predictive Maintenance Paradigm
The predictive maintenance paradigm represents a fundamental shift in how companies manage their assets and equipment, especially in the context of supply chain operations. Unlike traditional maintenance approaches, such as reactive and preventive maintenance, which are often characterized by inefficiencies and unexpected downtimes, predictive maintenance takes a proactive stance driven by data analytics and advanced technology.
At its core, predictive maintenance harnesses the power of data and cutting-edge algorithms to anticipate when equipment or machinery is likely to fail. This transformational approach relies on a combination of real-time data collected through sensors embedded in assets and historical data analysis. By continuously monitoring equipment health and analyzing data patterns, predictive maintenance provides early warnings of potential issues, allowing companies to intervene before a breakdown occurs.
One of the key distinguishing features of predictive maintenance is its ability to provide actionable insights. It empowers organizations to make informed decisions about when to schedule maintenance activities, ensuring that resources are allocated efficiently. This approach optimizes the use of maintenance personnel, spare parts, and downtime, resulting in significant cost savings and enhanced asset longevity.
Benefits of Predictive Maintenance in Supply Chains
-
Minimized Downtime: Predictive maintenance allows companies to schedule maintenance activities when they are most needed, preventing unexpected breakdowns. This minimizes downtime, ensuring that production and logistics operations run smoothly. Reduced downtime translates into higher productivity and, ultimately, increased revenue.
-
Cost Reduction: By identifying maintenance needs precisely and in advance, predictive maintenance helps companies avoid unnecessary maintenance activities. This means they can allocate their maintenance budgets more efficiently, focusing resources on critical assets rather than applying a one-size-fits-all preventive maintenance approach. Consequently, companies can achieve significant cost savings.
-
Improved Asset Lifespan: Predictive maintenance ensures that equipment is serviced or repaired at the right time and in the right way. This extends the lifespan of assets, reducing the need for frequent replacements. Since capital expenditures on new equipment are often one of the most significant costs in supply chain operations, extending the lifespan of existing assets can lead to substantial savings over time.
-
Enhanced Safety: Proactively identifying and addressing equipment issues through predictive maintenance enhances workplace safety. It reduces the risk of accidents and injuries resulting from equipment failures, which can have severe human and financial consequences.
-
Increased Productivity: Reduced downtime and efficient maintenance scheduling result in increased productivity within the supply chain. Orders can be fulfilled on time, and the entire production and distribution process becomes more efficient. This not only meets customer expectations but also creates opportunities for expanding business operations.
-
Data-Driven Decision Making: Predictive maintenance generates a wealth of data related to equipment health, performance, and maintenance history. This data can be used for informed decision-making across the supply chain. For instance, it can help in optimizing routes for transportation, managing inventory more effectively, and allocating resources where they are needed most. Data-driven decisions lead to improved overall supply chain performance and customer satisfaction.
Challenges of Implementing Predictive Maintenance
Implementing predictive maintenance in supply chains offers numerous benefits, but it also presents several challenges that organizations need to overcome. Let's delve deeper into the challenges associated with implementing predictive maintenance:
Data Quality and Integration
-
Data Accuracy: Predictive maintenance heavily relies on data, and the quality of this data is paramount. Inaccurate or incomplete data can lead to incorrect predictions and decisions.
-
Data Sources: Supply chains often have data stored in various systems, including legacy systems. Integrating data from these disparate sources into a cohesive and accessible format can be a significant challenge.
Sensor Deployment
-
Cost:Installing sensors on machinery and equipment can be expensive, especially for older equipment that was not originally designed with sensor integration in mind.
-
Logistics: The logistics of deploying sensors across a large supply chain network can be complex and time-consuming.
Talent and Training
-
Data Expertise: Organizations need to employ or train individuals with expertise in data science, machine learning, and predictive analytics to effectively implement and maintain predictive maintenance systems.
-
Technical Skills: Maintenance teams also need training to understand and act upon the insights provided by predictive maintenance systems.
Change Management
-
Cultural Shift: Transitioning from reactive or preventive maintenance to predictive maintenance requires a cultural shift within the organization. Employees at all levels need to embrace the new approach and trust the data-driven insights.
-
Resistance to Change: Resistance to change is common when implementing new technologies. Convincing employees of the benefits and providing adequate training is essential.
Scalability
-
Scaling Up: Expanding predictive maintenance initiatives across a large and complex supply chain can be challenging. What works for a small pilot project may not necessarily work at scale.
-
Maintenance of Sensors: As the number of sensors and data points increases, managing and maintaining them becomes more complex.
Predictive maintenance is transforming supply chain reliability by enabling companies to make informed decisions, reduce downtime, and cut costs. In today's highly competitive business landscape, supply chain optimization is a critical factor for success. By harnessing the power of data analytics and predictive maintenance, companies can stay ahead of the curve, meet customer demands efficiently, and ensure a reliable and resilient supply chain that can adapt to the challenges of the modern world. As technology continues to advance, predictive maintenance will undoubtedly play an increasingly pivotal role in supply chain management, allowing businesses to thrive in an ever-changing market.