Machine Learning's Role in Risk Management
Explore how machine learning is transforming risk management. Discover the impact of ML algorithms on risk assessment and decision-making.
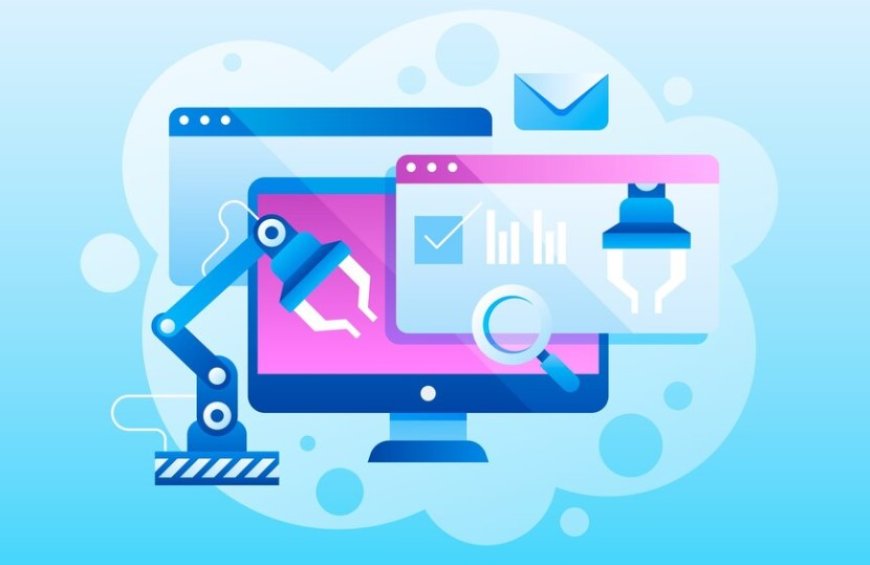
Machine learning has emerged as a transformative force in the realm of risk management, offering new avenues for identifying, assessing, and mitigating risks across various industries. In an increasingly complex and data-driven world, machine learning techniques are being harnessed to enhance traditional risk management practices. This technological evolution enables organizations to gain deeper insights, make more informed decisions, and proactively address risks, ultimately improving their overall resilience and competitiveness. In this exploration of machine learning's role in risk management, we will delve into its fundamental principles, applications, challenges, and the promising future it holds for optimizing risk-related processes.
Understanding Risk Management
Understanding risk management is fundamental to comprehending the crucial role that machine learning plays in this domain. Risk management is a multifaceted discipline aimed at identifying, assessing, and mitigating various types of risks that organizations face in their operations. These risks can range from financial uncertainties to operational vulnerabilities and strategic challenges. By systematically evaluating and addressing these risks, organizations can make informed decisions and better protect their assets, reputation, and long-term sustainability.
One of the key aspects of risk management is its proactive nature. Rather than merely reacting to adverse events, effective risk management involves a comprehensive assessment of potential risks beforehand. This allows organizations to develop strategies and contingency plans to minimize or even prevent negative outcomes. In traditional risk management, this assessment is often based on historical data, expert judgment, and mathematical models. However, with the advent of machine learning, risk management has gained a powerful tool to enhance its capabilities.
Furthermore, risk management is not confined to financial institutions alone. It is a critical practice across various industries, including healthcare, manufacturing, energy, and even the public sector. Each industry faces its own unique set of risks, and understanding the nuances of these risks is vital to tailor risk management strategies effectively.
Machine Learning Fundamentals
Machine learning is a subset of artificial intelligence (AI) that focuses on the development of algorithms and statistical models that enable computer systems to improve their performance on a specific task through experience and data, rather than through explicit programming. To understand the fundamentals of machine learning, it's important to delve into key concepts and components:
-
Data: Data is the lifeblood of machine learning. It can be structured (tabular data like spreadsheets) or unstructured (text, images, audio). Machine learning models learn patterns and make predictions or decisions based on data.
-
Algorithms: Machine learning algorithms are mathematical procedures that enable the model to learn from data. They transform the input data into a useful output, such as predictions or classifications. Common machine learning algorithms include linear regression, decision trees, support vector machines, and deep neural networks.
-
Models: A model is a representation of a system, process, or problem that a machine learning algorithm creates. It's the learned knowledge from the data. Models can be simple or complex, depending on the problem and algorithm used.
-
Training: Training a machine learning model involves providing it with a labeled dataset, which consists of input data and corresponding correct output or target values. The model learns to make predictions by iteratively adjusting its internal parameters to minimize the difference between its predictions and the actual targets.
-
Testing and Evaluation: Once a model is trained, it needs to be tested on new, unseen data to assess its performance. Common evaluation metrics include accuracy, precision, recall, F1-score, and more, depending on the type of problem (classification, regression, etc.).
-
Features: Features are the attributes or characteristics of the input data that the model uses to make predictions. Feature engineering involves selecting and transforming relevant features to improve model performance.
Machine Learning Applications in Risk Management
Machine learning applications in risk management represent a critical and rapidly evolving field that leverages advanced data analysis techniques to identify, assess, and mitigate various types of risks within organizations and industries. Here are some key explanations about the topic:
-
Credit Risk Assessment: Machine learning plays a pivotal role in assessing the creditworthiness of individuals and businesses. Traditional credit scoring models are often augmented or replaced by machine learning algorithms that analyze a wide range of data, including historical financial data, transaction records, social media activity, and more. These models predict the likelihood of borrowers defaulting on loans, helping financial institutions make more informed lending decisions.
-
Fraud Detection: Machine learning is instrumental in the detection and prevention of fraud in financial transactions. It can analyze large datasets in real-time to identify suspicious activities, such as unauthorized credit card transactions, identity theft, or fraudulent insurance claims. ML models continuously learn from new data to adapt and improve their fraud detection capabilities.
-
Market Risk Analysis: For investment firms and traders, predicting market trends and assessing market risk is essential. Machine learning models analyze historical market data, news sentiment, economic indicators, and other factors to make predictions about future market movements. These models help investors make informed decisions and manage their portfolios more effectively.
-
Operational Risk Management: Operational risks encompass a wide range of potential issues, from supply chain disruptions to IT system failures. Machine learning can be used to identify and quantify these risks by analyzing historical operational data, monitoring real-time operations, and predicting potential failures or vulnerabilities. Predictive maintenance, for instance, uses ML algorithms to anticipate equipment failures and prevent downtime.
Data Sources and Preprocessing
Data Sources and Preprocessing play a crucial role in the successful application of machine learning in risk management and many other domains. This stage of the data analysis pipeline involves the collection, selection, and preparation of data to ensure that it's suitable for feeding into machine learning models. Here are some key points to consider:
Data Collection Methods
Data can be sourced from a variety of places, including internal databases, external data providers, web scraping, and even sensor data in some cases. The choice of data source depends on the specific risk management problem being addressed. For instance, in credit risk assessment, historical financial records and credit reports are common sources, while in fraud detection, transaction logs and user behavior data are essential.
Data Quality
Data must be accurate, consistent, and reliable. Any errors or inconsistencies can significantly impact the performance of machine learning models. Data quality checks, cleansing, and transformation steps are often necessary to ensure that the data is fit for analysis. This can involve handling missing values, removing outliers, and addressing duplicate entries.
Structured vs. Unstructured Data
Data comes in various formats. Structured data, which is organized into tables or databases, is relatively easy to work with and is common in risk management. However, unstructured data, like text documents or images, may also contain valuable insights. Preprocessing techniques for unstructured data include natural language processing (NLP) for text and computer vision for images.
Data Privacy and Security
Risk management often involves sensitive and confidential data, such as customer financial records. Ensuring data privacy and security is paramount. Data should be anonymized or pseudonymized to protect individuals' identities and comply with data protection regulations like GDPR. Robust encryption and access controls should be in place to safeguard the data.
Machine Learning Models in Risk Management
Risk management is a critical function in various industries, including finance, insurance, healthcare, and supply chain management. Machine learning (ML) models have gained significant traction in enhancing risk management processes due to their ability to analyze vast amounts of data, identify patterns, and make predictions or decisions. Here's an explanation of the role of machine learning models in risk management:
Supervised Learning for Risk Prediction
-
Supervised learning is a fundamental ML approach where models are trained on labeled historical data.
-
In risk management, supervised learning is used for risk prediction and classification tasks.
-
Regression Models: These models are used to predict numerical values, such as estimating the probability of loan default or the potential financial loss in a specific scenario.
-
Classification Models: They are employed to classify instances into predefined categories, such as identifying whether a transaction is fraudulent or not.
-
Supervised ML models can leverage historical data to learn relationships and trends, helping organizations make informed risk assessments.
Unsupervised Learning for Anomaly Detection
-
Unsupervised learning is suitable for detecting anomalies or outliers in data without labeled examples.
-
In risk management, this is crucial for identifying unusual patterns that may indicate fraud, operational errors, or other unexpected events.
-
Clustering Algorithms: These group similar data points together, helping to detect clusters of risk in a dataset. For instance, identifying customer segments with similar credit risk profiles.
-
Outlier Detection: Unsupervised models can spot data points that deviate significantly from the norm, which is essential for detecting irregularities like fraudulent transactions.
Machine learning has become a cornerstone of modern risk management, offering unparalleled capabilities in data analysis, predictive modeling, and automation. As organizations continue to accumulate vast amounts of data, the integration of machine learning in risk assessment will only become more essential. By harnessing the power of machine learning, businesses and industries can make more informed decisions, reduce losses, and adapt to an ever-changing risk landscape.